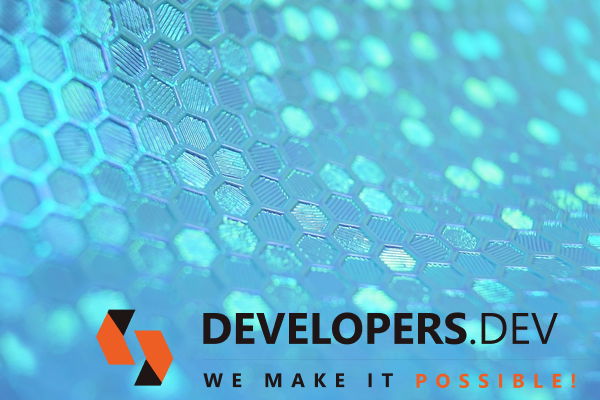
"Big data" is an umbrella term used to describe the storage and processing of large volumes of data. Therefore, the big data industry is emerging as one of the critical drivers, not just in the US but across countries and continents.
Regarding big data solutions, certain organizations have substantial obstacles to overcome. A company intending to integrate big data solutions must take into account all of the available alternatives, including software and deployment strategies.
In any big data project, defining your business needs is the first step. You can see the outcomes expected thanks to this step-by-step instruction.
The organizations effectiveness will be based on how successfully the big data analytics system is connected with the current applications to give real-time and seamless insights.
Big Data - A New Upcoming Sector
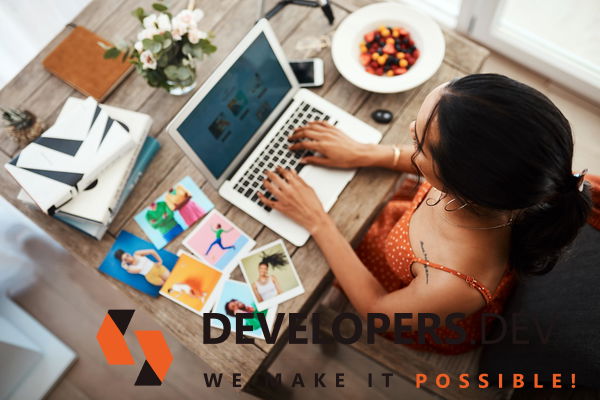
Big data is a hot, emerging industry where ample opportunities lie. Businesses, start-ups, and established companies are investing in big data, as are governments, which capture data on their citizens and use it to garner insights.
Big data has several significant implications for businesses. Still, one of its most significant aspects is its impact on marketing and its potential for transforming customers experiences.
The big data revolution has disrupted how businesses collect data and, in turn, the data analytics they carry out.
A crucial part of this transformation is the move from batch processing to real-time processing. Traditionally, data analysis was carried out using batch processing, where data was processed in batches.
The batch processing approach resulted in a substantial delay. This, in turn, limited businesses ability to respond to changing consumer needs, which could have a tremendous impact on customer loyalty and the bottom line.
Now, however, real-time processing is taking center stage with the advent of the big data revolution. Real-time processing, based on collecting and processing data at the point of transaction, enables businesses to make better decisions, leading to faster profits.
Big data is a phenomenon that has been in the making for quite some time. It began with early attempts at storing and accessing data.
However, with the emergence of the cloud and the onset of the SaaS revolution, we saw a true data revolution. Integrating SaaS and big data has enabled organizations to store and access data and use it effectively. Along with big data, the cloud is now facilitating a digital transformation that changes how organizations work.
Big data analytics is also fuelling a data revolution in many sectors.
The retail industry, for example, is witnessing a data-driven revolution, with big data enabling retailers to understand their customers better. Big data also transforms how organizations work by enabling enterprises to extract actionable insights from large data sets.
The amount of data available to organizations has skyrocketed, which has opened up opportunities for enterprises to analyze data in different ways.
Integrating big data and the cloud has meant that enterprises have access to vast amounts of data and can use these to analyze their businesses or derive actionable insights.
The cloud, too, has given rise to a new kind of analytics, and organizations are leveraging the cloud environment to analyze vast amounts of data. The cloud has driven the big data revolution because organizations can integrate big data and analytics seamlessly.
The SaaS revolution also facilitated the integration of big data and analytics.
The cloud has played a vital role in enabling big data and analytics because organizations can now leverage cloud environments to store, access, manage, and analyze data.
The cloud has also made it easier to access data because it is now possible for developers to access data from anywhere.
What is Big Data?
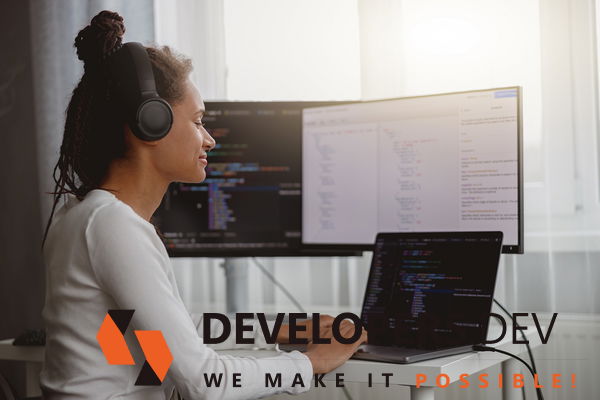
Many define "big data" as a set of data so large that traditional data analysis methods cannot handle it. Yet, this definition merely scratches the surface: exhaustivity, relativity, extensionality, variability, and veracity are other essential features of big data.
Exhaustiveness: In big data, data exhaustiveness refers to the number of data units. Data exhaustivity refers to the quantity and variety of data that needs to be processed.
In big data, data exhaustivity is much higher than in traditional data processing.
Relativity: In big data, "data relativity" refers to the degree of data transformation. With traditional data processing, data transformation is relatively more straightforward, while data transformation in big data is much more complex.
Extensionality: In big data, data extensionality refers to the number of data dimensions. In big data, data extensionality is much higher than in traditional data processing.
Variability: In big data, data variability refers to the degree of data transformation. Traditional data processing has a stable input, but in big data, the information changes significantly.
Veracity: In big data, data veracity refers to the degree of data verification. Data verification is relatively simple with traditional data processing, while data verification is much more complex with big data.
Big data is a by-product of the internet. Still, it is also a product of an economy that sends signals across oceans, runs on assembly lines with robots, and involves sophisticated management.
There are far fewer bad ideas in a data-driven environment, and those who manage to survive tend to be better. "Big data" is a tidal wave. But its hard to see exactly where the tide is coming from. From which direction?
The clearest direction is from the consumer. With a personal computer, camera, and microphone, customers have cheap ways of collecting and storing data.
They can then analyze it using software or upload it to companies such as Google, which offer services for storing, analyzing, and presenting the data. This data is precious.
To compete, traditional companies are also developing their databases, and they are also spending billions of dollars on software that can analyze customer behavior.
These databases offer companies the chance to be more flexible and make better decisions. But they also give customers more power.
They allow customers to see what companies are doing with them. If a customer likes a product, she can buy it repeatedly, and the company will know.
If she doesnt like the product, she can stop using it, and the company will know. This is tremendously powerful. It means that customers can make companies do things that customers want them to do.
Big Data Solutions
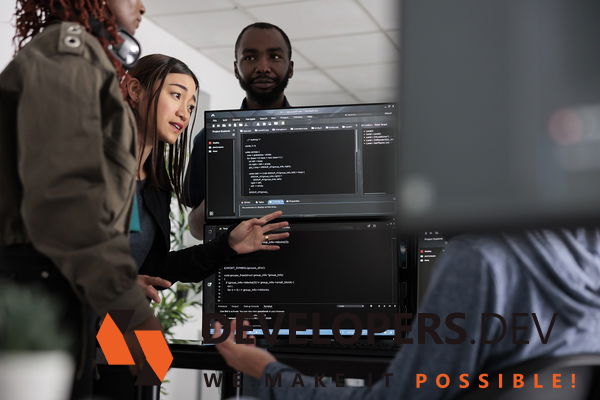
Lets look at some of the examples out there:
Big Data in Healthcare
One of the most exciting things in healthcare is the possibility of using big data solutions for tracking patients biometrics.
Researchers are currently investigating the possibility of using iris recognition technology, for instance, to monitor elderly patients health. It is a technology that is already being tested. The technology can accurately track the patients iris patterns, and therefore, doctors could monitor their health more accurately.
Iris recognition has been used for access control, face recognition, and biometric identification.
Big data in the healthcare industry refers to both genomics-driven experiments (such as genotyping and gene expression data) that are driven by payers and providers (such as EMRs, prescriptions, and insurance records), as well as the genomics-driven experiments data (IoT).
It is typical to examine vast volumes of data from numerous studies to get fresh insights. A surge in articles regarding big data in healthcare lends credence to this.
Big Data in Education
We know that big data and analytics can help education, but how can we know if theyre working? The answer to this question focuses on much current research, and the answer is far from obvious.
Big data and analytics have given us powerful new tools for assessing students and evaluating ourselves. Using these methods, we can measure almost every aspect of learning-from test scores to engagement to the number of words a student has read.
One of the most intriguing metrics is learning analytics, which attempts to measure what students do in school.
"Big data" is the term used to describe the enormous and ever-increasing data that various companies gather. Engineers and scientists acquire, evaluate, and structure data using quantitative and qualitative methods to gain knowledge that will aid decision-making by leaders.
Organizations can forecast trends, comprehend human behavior, and develop better new solutions thanks to these insights.
Big data in education is a tool that can change academic outcomes for students and educators, as well as schools and institutions.
Big data insights can also enhance educational institutions digital infrastructure. Big data transforms how schools evaluate information and make choices regarding organizational outreach, faculty effectiveness, and academic performance.
Want More Information About Our Services? Talk to Our Consultants!
Big Data in Banking
Money is fuel for the engine of capitalism, a primary form of money. But it doesnt have to be. It can be something else.
Or it can be multiple other things. A bank could sell a promise to repay the money and then collect the money back later. It might sell a bond and collect interest.
It might sell a policy and collect a death benefit. But any amount of money, if you sell it this way, will put a ceiling on the amount of money the bank can make. The bank can only make so much money by lending out cash, which has to be enough to cover the interest payments on the bonds and policies sold.
Because a banks assets are all tied up in what has been lent and what has been collected as interest, it cant make more assets than the money it has lent out.
And since the assets it lends out are our money, it cant make more money out of the money it has lent out. The bank is stuck with the money it has lent out and the money it has collected as interest.
But what if it didnt have to lend out the money it has? What if it did have enough assets to cover everything? What if the bank could sell assets other than money? It could sell assets that other people wanted but couldnt afford.
It could sell assets that some people didnt want but others did. It could sell assets that other people wanted. Still, it couldnt afford assets that other people didnt want but could afford.
The first big-data insight was a big one. People of all income levels use smartphones, and smartphones have sensors.
So, smartphones provided a significant opportunity for banks. Banks could figure out who was spending the most time on their smartphones and use this insight to underwrite loans.
If a borrower spent enough time using a smartphone, the bank could safely assume the borrower had a job and, therefore, could afford a loan.
Big Data in Smart Cities
There will be 64 billion connected devices, generating nearly ten zettabytes of data. This data will enable smart cities, where the Internet of Things (IoT) will significantly improve quality of life.
IoT, which refers to communication between physical objects, is expected to generate tremendous innovations, leading to changes in business processes, products, and services. The IoT value chain includes devices and sensors, networks, analytics, and applications.
Smart city initiatives use big data to improve urban infrastructure, increase the efficiency of public services, develop city services and policies, and increase productivity.
Smart energy and utility initiatives use big data to improve energy efficiency, reduce energy losses, and improve grid reliability. Utilities can optimize energy distribution, improve equipment performance, increase equipment utilization, and enhance the customer experience with data and analytics.
Big Data in Smart Manufacturing
Smart manufacturing initiatives use big data to improve manufacturing processes. Big data can be helpful in places where the physical infrastructure is already in place, but people arent using it.
Big data can help people do things they wouldnt otherwise do. It can make travel more efficient by predicting where congestion will occur and rerouting around it. Or it can help people find more information.
In education, big data can make it easier for teachers to find things for students to read. It can help teachers find more books that students want to read and help students find books that teachers think they will want to read.
Big Data in Agriculture
There are two reasons to use big data in agriculture. One is to get more out of the land. The other is to avoid wasting it-first, getting more out of less.
If agriculture has followed the manufacturing path, then the focus of improvement has been on getting more output per worker. In agriculture, labor is scarce and expensive, so you shouldnt waste it.
If you could measure how much labor went into producing a crop, you could shift money away from the most expensive inputs, like fertilizer, and put more into labor.
If you knew exactly how much fertilizer, labor, and machinery were needed, you could plan how much of each to buy, and shift resources from what you didnt need toward what you did.
The second reason to use big data is to avoid wasting it. If you only need to produce one crop a year, it makes sense to use the newest techniques and most modern technology.
If you can grow 20 crops a year, you might as well use what you already have. But if you can only grow one crop a year, then it makes sense to use the newest techniques and most modern technology.
Ill use the example of rice: if you plant one rice field, it makes sense to use the newest techniques and most modern equipment.
But if you plant 20 fields, then it makes sense to use whatever works: whatever works for your soil type, your climate, your irrigation system, your equipment, your labor, and your timetable.
The complexity of the modern agricultural industry makes it hard for farmers to keep up. To manage all the inputs, outputs, and variable conditions, farmers need information.
Today, that information is voluminous and varied. Farm sensors help control conditions in the fields and on farms. They track everything from soil moisture to weather forecasts to soil acidity.
But data alone cant guide farmers. They need to know what the data mean, and they need to know how to use the data.
Data-driven farming also relies on smart devices. Farmers can use GPS units to trace where crops have been grown and where theyve been stored.
They can also use smart tractors that monitor soil conditions and automatically apply fertilizer and chemicals.
Read More: What Are The Possible Solutions Of Using Big Data?
Big Data Implementation Roadmap
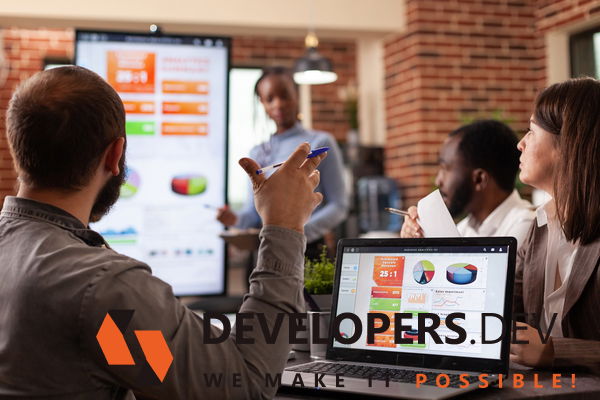
Refine your Existing Data
The quality of your data is just as important as the size of your data pool. Most organizations spend time and money collecting and storing data but neglect to evaluate the datas quality objectively.
This lack of attention to the data quality process may be a significant reason why the latest big data technology has not had the same adoption rate as other technologies.
Poor data quality leads to inaccurate analysis and incorrect decision-making. Poor data quality causes data miners to spend countless hours cleaning data.
This wasted effort can cost your organization thousands of dollars per month.
The data scientists role may be the most misunderstood of all the IT roles being asked to fill. One reason is that while data scientists roles are often specified and described in general terms, there is no standard job description.
Data scientists are often recruited to fill positions with titles such as "data analyst" or "data engineer." However, these roles cover all aspects of data, including data quality.
Data quality is an area often overlooked in job descriptions and candidate interviews. This is not because hiring managers are unaware of how important data quality is.
Instead, data quality is considered the purview of the data administrator, a data architect, and a developer.
Data quality is, in fact, one of the most critical components of modern big data projects. Often, a data quality measure is the most important factor in determining whether an enterprise big data project is successful.
Whether it is a data quality measure related to data quality or one that evaluates the quality of data integration, the success or failure of a big data initiative often depends on the quality of data. Data quality requires three primary components: data quality tools, data quality policies, and data quality training.
Assess the Results and Evaluate your Progress
Organizations invest heavily in data analytics but fail to get the most out of their big data analytics projects.
The problems often lie in poor implementation and inadequate management. Data analytics projects fail because of a lack of ownership, poor project management, a lack of executive sponsorship, and a lack of data governance.
Lack of ownership: While data analytics is in the early stages of maturity, most organizations use analytics to address real business problems.
But ownership of analytics projects is often in the hands of different, often competing, teams.
Poor project management: Poor project management often leads to problems and delays.
Lack of executive sponsorship: This ties in with project management. Executives should be determined to see that data analytics projects succeed.
Lack of data governance: data governance is the process an organization has put in place to manage data and define how it should be used and shared.
Want More Information About Our Services? Talk to Our Consultants!
Conclusion
The rise of big data technologies has already disrupted almost every industry. The financial industry is the most obvious example, but other sectors are also experiencing profound changes.
Big data technologies are already permeating almost every industry, increasing revenue and efficiency, and accelerating the digital transformation.
By now, its evident that big data implementation laggards will soon find themselves in a highly vulnerable position, unable to withstand the growing competition and keep up with the changing standards.
A well-thought-out and strategic approach are necessary for the adoption of big data solutions, nevertheless. By articulating your company objectives and assessing your resources, you can assure a seamless and disruption-free transition to big data.
A fruitful IT alliance is also essential to maximizing the business potential of big data.