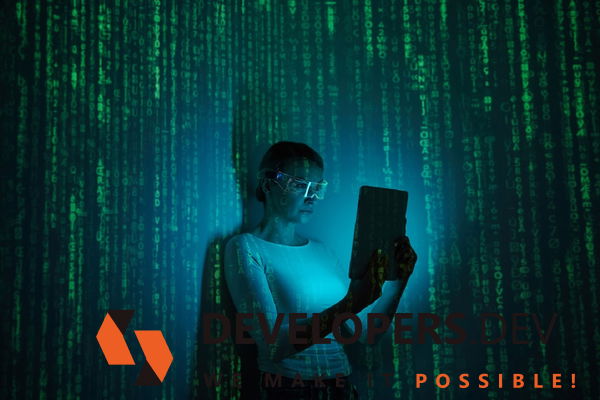
According to Bitkoms spring 2021 survey, AI systems are receiving a lot of attention. AI is a top technology that companies consider the future, with two-thirds of those surveyed believing it to be the most important.
One in four companies would like to invest in this field. One third expects to gain expert knowledge via artificial intelligence that is otherwise impossible. Predictive analytics is a hot topic.
There are many opportunities for predictive analytics. These include reducing the use of resources, creating new products and solutions that customers can use, and making processes more efficient.
If the AIs predictions do not come to pass or there is no gain in knowledge, it is not unusual for people to feel disillusioned.
Ai Cant Do Miracles
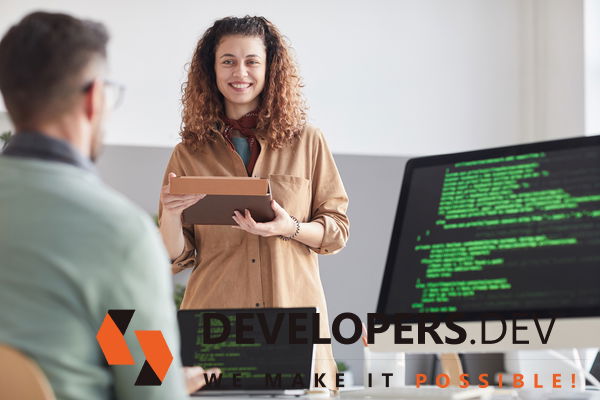
Artificial intelligence is a complex field that requires intensive attention. Anyone who works with it more often knows that their foundations are not as reliable as the system itself.
People who are susceptible to cognitive distortions may create the algorithms. In the context of automated credit checks, the bias phenomenon has been widely discussed in the media. AI cannot be impartial because it is made by humans.
This is because programming can be influenced by the biases of developers, no matter how intentional or not. The results can also be affected by the unconscious or conscious data ethics used by developers.
The quality of the input can also play a role in determining why an AI process does not produce the desired results.
This is the same as image processing. She cant turn a bad photo into a masterpiece. AI cannot produce a great outcome if there isnt enough input.
Improve Data Quality
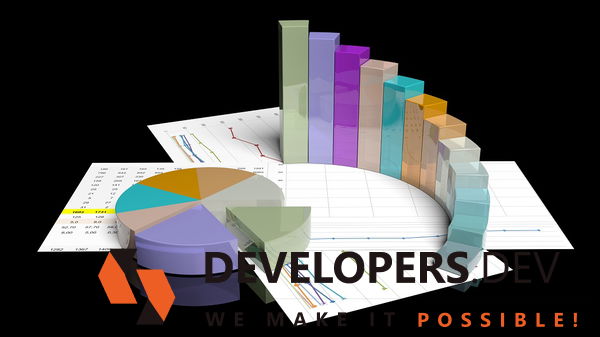
Combining data with the ETL (Extract Transform, Load) is the key to convincing results when using artificial intelligence.
It is essential to have a solid data strategy that ensures data quality.
The Quality Criteria are for Measuring Data Quality
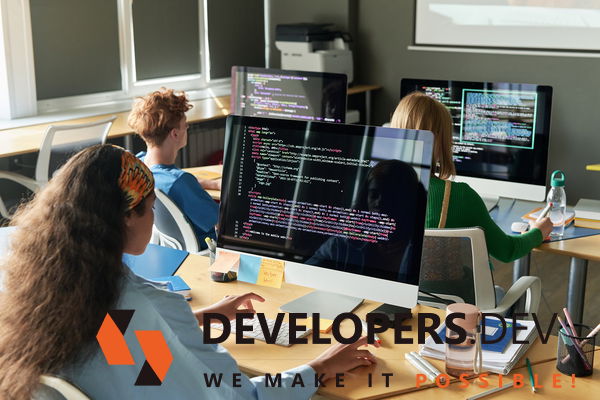
-
Completeness: This concept refers to various dimensions.
Complete data is when all content and data are fully adopted in an ETL process.
When 100% completeness is reached, the business rules of the company determine.
- Correctness: A data set is correct when it matches reality.
- Consistency: A datasets properties must not be in contradiction with any other data sources or other datasets.
- Uniqueness: is when only one of the objects (real) described in the dataset is represented.
- Conformance: Data must adhere to the specified format.
- Validity: Data correspond to the specified value ranges.
Rules can improve the quality of data by determining its quality. There are two types of rules:
- Formal-technical and technical rules
- content rules.
Software can easily implement formal-technical rules. The softwares data will be the starting point for analysis.
It is easy to determine if a data record has been completed by using a simple formula (e.g. the ratio of the attributes that are filled and the number of features that exist). Technical support is available for content-related rules.
Data quality is automatically improved by using plausible checks to prevent inadvertent entries.
The fundamental issue is that AI systems cannot deliver accurate (and impartial) results if the data is not in a suitable and clean format.
This is the most common error in AI analysis. The data quality must also be assessed based on the use cases. These results are then used to create a GAP analysis, which shows if additional data is required or where gaps remain.
AI Projects dont Just have to be IT Projects
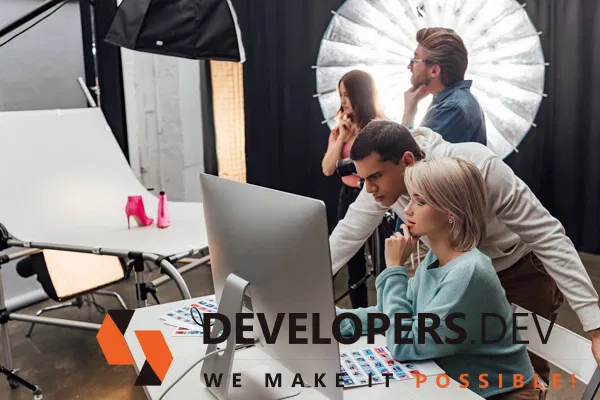
The second mistake is closely related to the poor quality of data. Its a misinterpretation of AI systems.
This is not a project thats solely technology-related. It is often misleading to assume that IT service providers and contractors can set up a complete system of software and technologies, and then the rest will run by themselves.
Most people understand the need to eliminate data silos from a technical standpoint. It takes more than just letting data flow freely to be successful in AI analytics projects. People must be able to see past the silos metaphor.
AI projects are also changing.
Data Owners dont know What Quality Data Should Have
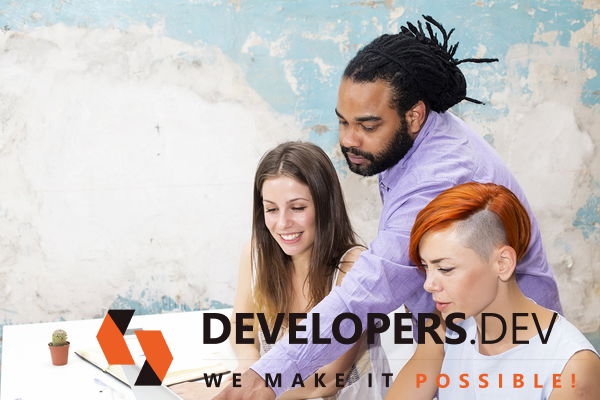
This is also connected to the aspect of data quality. Data quality is often a responsibility of the department (data owner or information owner) that holds the required data.
This can lead to misinterpretation of the importance of data quality. This is not a bad idea. The department does not see the benefits that this brings to their department. This is often seen as a on top job, which doesnt add any value.
There is little motivation to improve data quality.
It is not enough to just remove data silos and create data lakes, then let the data scientists work alone. Data owners in departments must be convinced that higher data quality can bring them benefits, i.e.
real added value.
Want More Information About Our Services? Talk to Our Consultants!
Data Product Team Combines Technology with Corporate Culture
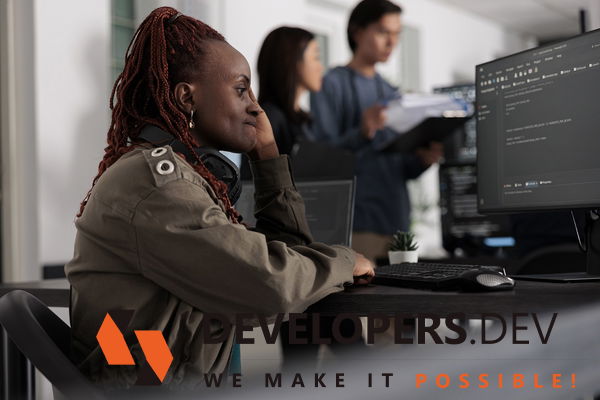
Successful AI data analytics projects require a corporate culture and the sharing of common goals.
This is possible when the work is data-centric. That means that all stakeholders, including data scientists and engineers, work together to develop specific questions and define use cases.
Direct project work communicates the importance and sustainability of data quality better to enable us to take steps to improve it. Because they will have to continue supporting the ongoing processes and working with the information, the departments needs must be the focus.
Only a team can achieve high data quality and meaningful AI analyses.