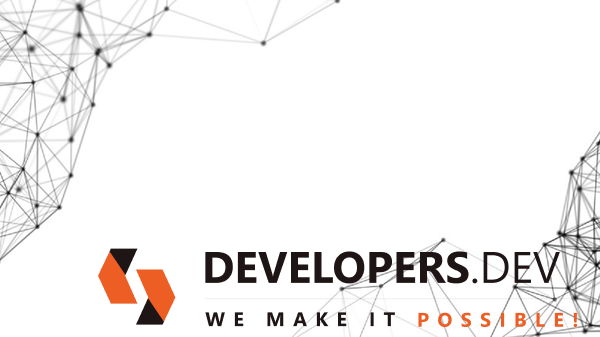
Business Analytics
Business analytics is a common practice today because companies want to improve their sustainable business performance and analyze data in order to make better business decisions.
Businesses are aiming to gain more value from analytics, using more data and generating deeper insights faster for more people. You need a cloud analytics platform (PDF) that can support the entire analytics process while providing the security, flexibility, and reliability you demand.
It must empower users to perform self-service analyses without sacrificing governance. It must also be easy to use.
How Can You Achieve The Same Benefits As An Enterprise System Without Having To Pay For Enterprise Costs And Infrastructure?
Business analytics, which uses personalization, machine learning, and domain-specific knowledge, can help companies gain actionable insights by analyzing data from applications, data warehouses and data lakes.
Business analytics is a process that should lead to a specific action. Once insights have been gained, businesses can reevaluate, re-execute and reconfigure their processes. The key is to take action.
Analytics Fundamentals
Data by itself has no meaning. All our hard work will be in vain if, after learning every lesson possible, we do not act.
We will not get the full value of our investment if we do not leverage all technology trends available to us. Today, our data can be used to answer questions, predict future outcomes and learn new patterns. Your data has this potential.
Analytics: The Value To Business
-
New Ways Of Working
With the changing nature of business comes a whole new way to compete. To keep up with todays tech-savvy workers, you need to be able to create value quickly and efficiently. You can deliver speed and simplicity while maintaining high standards of data quality and security. Your business analytics strategy should include a centralized analytics platform in which IT plays an important role. Innovation is best achieved when business and IT initiatives are combined.
-
Discover New Opportunities
The latest advances in analytics technology offer you new ways to leverage your data. Modern analytics is adaptive, adaptive, and self-learning to uncover hidden patterns. The analytics are also intuitive, with stunning visualizations allowing you to quickly understand millions of rows of data. Modern business analytics is mobile and simple to use. They connect you with the right data, at the right moment, and require little or no training.
-
Visualize Your Data
You want to be able to read the signals in your data before your competitors. The analytics tool allows you to get a clear picture of your business. You can easily understand the value and share the story of your data with colleagues by combining personal, corporate and big data.
The History of Analytics
Analyzing data and comparing statistics predates written history. However, there were some important milestones which helped to develop the analytics process we know today.
William Playfair invented the bar chart in 1785. It is now one of the most widely used data visualization tools.
According to the story, he created bar charts in order to display a few dozen points of data.
Charles Joseph Minard, a mapmaker in 1812, plotted the losses Napoleons army suffered during their march to Moscow.
He began at the Polish-Russian frontier and created a map with thick, thin lines that showed how Napoleons army suffered losses due to the cold winter and the length of time they were away from the supply lines.
Herman Hollerith created a "tabulating" machine in 1890 that recorded data using punch cards. The data could be analyzed more quickly, which sped up the U.S.
Census counting process from seven years down to 18 months. The business requirement was to improve data collection and trend analysis. This is still followed today.
Want More Information About Our Services? Talk to Our Consultants!
Today, Analytics Is A Present
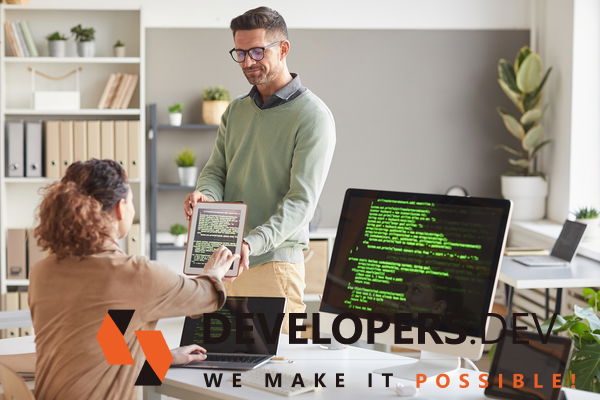
In the 1970s and 80s, software such as Standard Query Language and Relational Database (RDB) were developed to allow data analysis on demand.
An Analyst in the late 1980s proposed the idea of a data warehouse where information could easily be accessed repeatedly.
analysts coined the term "business intelligence" which led to an industry push towards analyzing data in order to better understand business processes.
Data mining was a concept that emerged in the 1990s. It allowed companies to discover patterns and analyze large datasets.
Data scientists and analysts used programming languages such as R and Python to create machine learning algorithms, manipulate large datasets and create complex data visualizations.
Future: Next-Generation Analytics
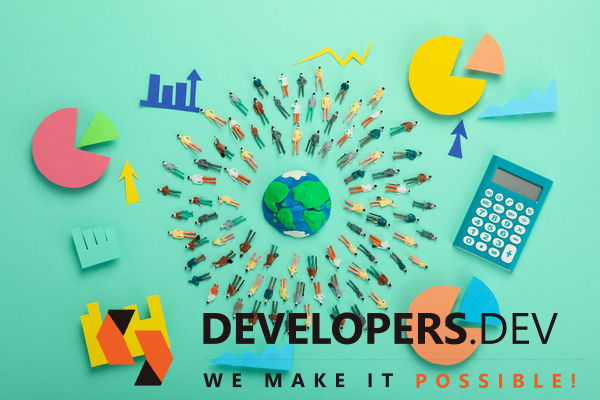
The tools have also evolved to meet the needs of businesses that are no longer just interested in gaining visibility but require more insight.
The first analytics toolkits were built on semantic models created by business intelligence software. They helped establish strong governance, data analyses, and alignment between functions.
exclusive reporting was not always available in a timely manner. The results of the query were not always aligned to what was asked. These models are not cost-effective from a technical perspective, as they are used primarily on premises.
Data is often locked in silos.
The evolution of AI and BI services tools has advanced analytics for a wider audience.
They were easy to use and did not require any special skills. In the last few years, these desktop business analytics tools gained in popularity. Users are interested in exploring the wide range of data assets.
The ease of use may be appealing, but the blending of data to create a "single truth" is becoming increasingly complex. Desktop analytics may not be scalable for larger groups. Also, they are susceptible to inconsistent or ambiguous definitions.
In recent years, analytics tools have enabled a wider transformation of business insights with tools that automatically upgrade, automate, and cleanse data.
Users can work with any device, in context, to harness information in real-time and achieve business outcomes.
Automation is becoming more popular. Humans still do the majority of work today, but its gaining in popularity. It is easy to combine data from different sources.
Consumers can gain insight from visual representations and build models by interacting. All of these are managed and controlled at a very fine level by humans. Data gathering, data discovery and machine learning give the user more options than ever.
Business Analytics: Embrace It
Every aspect of our life is affected by analytics. Analytics can help you answer any question, whether it is about your employees, finances or customers and their likes and dislikes.
It also helps you make better decisions.
Analytics Trends
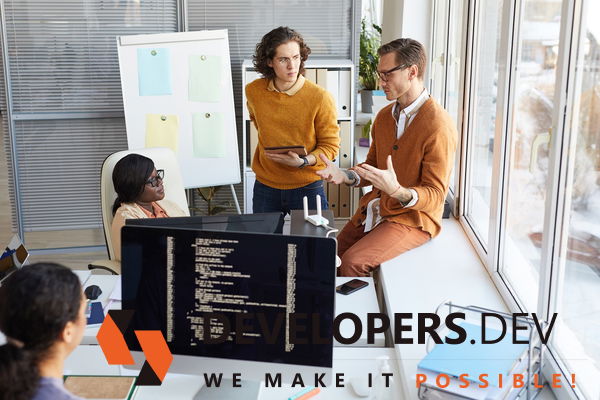
In the ever-evolving analytics market, it is the norm to have the business and IT work together to make decisions.
It is clear that analytics has been adopted as a strategic tool by most organizations. This has led to a whole new generation of consumers, with new expectations.
The way decisions are made and communicated in real-time with a large audience has changed. This change in the workforce brings with it a new approach to working.
The days of office training manuals have passed. Todays workforce is used to a quick and intuitive interface. It doesnt stop there. Business leaders have high standards for data security and quality, even though speed and simplicity is key.
Any analytics strategy must still include a centralized analytics platform in which IT plays an important role. Innovation is best achieved by combining business-led initiatives with IT-led ones.
We believe that placing analytics in the cloud goes beyond just being a choice of deployment. It breaks down barriers between people, places and data systems, allowing people to interact more effectively with technology and information.
Read More: Machine Learning, AI, And Deep Learning
Adaptive AI systems
Reengineering decision-making is becoming increasingly important as decisions become more contextual, continuous and connected.
You can achieve this by using adaptive AI, which allows for more flexible and faster decisions.
To build and manage adaptive AI, you should adopt AI engineering techniques. AI engineering orchestrates, optimizes and adapts applications in order to resist, absorb or adapt to disruptions.
This facilitates the management of adaptive systems.
Data-centric AI
Data management is often overlooked by organizations when tackling AI. Sallam says that building AI without the right data is dangerous and risky.
It is therefore crucial to formalize AI-centric and data-centric AI. As part of your data strategy, they address data biases, diversity, and labeling more systematically.
Metadata-Driven Data Fabric
Data fabric is a data-driven system that listens to, learns from and responds to metadata. It flags and suggests actions to people and systems.
It improves the trust and use of data within an organization.
For example, Turku in Finland discovered that gaps in its data were holding back its innovation. It was able, by integrating fragmented assets of data, to reuse data and reduce the time to market in half.
Always Share Data
Data and analytics leaders acknowledge the importance of data sharing as a digital transformation capability. However, they do not have the knowledge to share data with confidence and at scale.
Collaboration across industries and business lines is key to promoting data-sharing and increasing access to the right data that aligns with the business case.
This will increase the buy-in to increased budget authority and investments in data sharing. Consider adopting a data fabric design in order to create a single architecture that allows data sharing between heterogeneous data sources, both internal and external.
Context-enriched Analysis
Context-enriched analyses are based on graph technology. A graph is used to store information about the context and needs of the user.
This allows for a deeper analysis by using both the relationships between the data points and the data itself. It allows for a deeper analysis of the data points based on their relationships, constraints, paths, and communities.
To capture, store and use contextual data, you need to have the skills and capabilities in building data pipelines.
You also need AI cloud services and X analytics that can handle different types of data. By 2025, 60% of the current models based on historical data will be replaced by AI and context-driven analytics.
Business-Composed D&A
Analysts advocates a modular approach to analytics and data, also known as "composable D&A". Business-composed analytics and data builds on this trend but the focus shifts from IT to the business side.
Business-composed D&A allows business users and business technologists to collaborate to create business-driven data analytics capabilities.
Embedded Business Intelligence
Reports, dashboards, and data visualizations are all integrated into an application through embedded business intelligence.
Information is displayed and managed directly in the user interface of an application by a BI Platform. This improves data usability and helps make better decisions and avoid poor decisions.
This embedding of BI into existing applications gives users and customers fresher information, context, and richer context within the operational applications that they are already familiar with.
The integration of BI into operational or customized applications that are used every day increases user adoption and makes the applications more valuable than traditional analytics or BI. The adoption of BI solutions puts data in the hands of users, allowing them to gain greater insights and take smarter action based on that data.
Decision-centric D&A
Organizations are rethinking their investment in D&A due to the discipline of decision intelligence. This is the careful consideration of how decisions need to be made.
You can deliver the required inputs and design the finest decisions with the aid of decision intelligence disciplines.
Researchers predict that in 2023 more than 33 percent of large organizations will employ analysts who are trained in decision intelligence and decision modeling.
Shortfall In Literacy And Skills
D&A leaders must have talent on their team in order to achieve measurable results. Virtual workplaces and increased competition for talent has led to a lack of data literacy - the ability to read and write data and present it in context - among the workforce.
Researcher predicts that most CDOs, by 2025 will have failed to instill the data literacy necessary within their workforce to meet their strategic data-driven goals.
In order to recover the costs incurred by investing in employee training and data literacy, you can include "clawback" or "payback clauses" in contracts for new employees.
Connected Governance
The organizations need to have effective governance on all levels. This includes addressing their operational challenges but also being flexible, scalable, and responsive to the changing dynamics of the market and organizational strategic challenges.
The pandemic has highlighted the need for cross-functional collaboration, and the willingness to change organizational structure to achieve business model flexibility.
Connected governance can be used to create a virtual D&A layer that spans across functions and geographical areas to achieve desired business outcomes.
AI Risk Management
When organizations invest time and resources in supporting AI TRiSM (Trust, Risk and Security Management), they can expect to see better AI outcomes, including adoption, business goals achieved, and internal and external user acceptability.
Study estimates that organizations who develop AI that is trustworthy and purpose-driven will succeed in 75% of their AI initiatives by 2026.
This compares to only 40% for those who dont.
A greater focus on AI TRiSM leads to a controlled and stable implementation of AI models. We also expect fewer AI failures including incomplete AI projects and a decrease in unintended outcomes.
Read More: The benefits of Machine Learning and Artificial Intelligence
Cloud Analytics
Cloud analytics is a process that involves storing, analyzing and using data in the cloud to gain actionable insights.
Cloud analytics algorithms work similarly to on-premises analytics. They are used on massive datasets to find patterns, forecast outcomes, and give decision-makers in business other helpful data.
Cloud analytics, however, is a much more cost-effective alternative to on premises analytics, which require businesses to buy, maintain and house expensive data centers.
On-premises solutions are more secure and give businesses greater control of their data, but they can be difficult to scale. Cloud analytics benefits from the scalability of cloud computing, its service models, and the cost savings.
In the course of their daily operations, businesses generate terabytes worth of data. Most of the data, which comes from social media, websites, IT devices, financial software and other sources, is stored in the cloud.
Cloud analytics software and tools are especially efficient at processing large data sets. They produce insights in digestible formats, and create insights from cloud data available on demand.
Cloud analytics tools are especially efficient at processing huge data sets. They produce insights in digestible formats, on demand.
This results in a more streamlined and better user experience.
Vendors And Regions Ecosystems
In the new multipolar environment, this trend will intensify.
Consider migrating or duplicating all or part of your D&A Stack within certain regions. Manage a multi cloud or multi vendor approach by design or default.
Consider taking several steps to create a cohesive ecosystem of cloud data. Consider aligning your solutions with those of your vendors based on their ecosystem and extensibility.
Reevaluate your policies for a best of breed or best fit strategy in cloud D&A by evaluating the advantages of a single vendor ecosystem, in terms of speed, cost and agility.
AI/ML
Artificial Intelligence (AI) is a term that refers to algorithms and processes capable of simulating human intelligence.
This includes mimicking cognitive functions like perception, learning, and problem-solving. AI includes machine learning and deep-learning (DL).
AI has many practical applications, including modern web search engines and personal virtual assistants that can understand natural language.
Other examples include self-driving cars and recommendation engines like those used by Spotify or Netflix.
Machine Learning (ML), a subset AI, falls under the "limited memory" group. AI (machines) can learn and improve over time.
There are many different machine-learning algorithms. The three main types include supervised learning (supervised learning), unsupervised learning (unsupervised learning) and reinforcement learning.
Data is a valuable business asset. The amount of data being generated and stored worldwide is increasing at an exponential pace.
Its true that collecting data is useless if nothing is done with it. However, these massive floods of information are impossible to manage without automated systems.
Artificial intelligence, deep learning, and machine learning allow organizations to get the most out of their data, by delivering insights, automating processes, and improving system capabilities.
Expansion At The Edge
D&A operations are increasingly being carried out on distributed servers, gateways, or other hardware outside of data centers and the infrastructure of public clouds.
Edge computing environments are increasingly used to house them, as they are closer to the places where data and important decisions can be created and executed.
analysts predict that more than half of the enterprise-critical data created and processed by 2025 will be outside data centers or clouds.
Support data persistence by integrating edge-resident IT technologies (relational or non-relational database systems) and small-footprint embedded databases for processing and storing data closer to device edges.
Want More Information About Our Services? Talk to Our Consultants!
The Conclusion Of The Article Is:
Data is the new oil. But to get it, you need a powerful engine. Organizations with a solid analytics foundation, a strong analytics competency and culture will be able to innovate and make better decisions.
Analytics trends are constantly evolving, and companies need to keep up with the latest trends in order to stay competitive.
Cloud, AI/ML and embedded BI are some of the top analytics trends that have been gaining steam in recent years. These technologies allow businesses to gain deeper insights into their data, which can be used to drive better decision-making and improve overall performance.
By staying ahead of the curve on these trending technologies, organizations will be able to more effectively leverage data-driven insights for continued success.