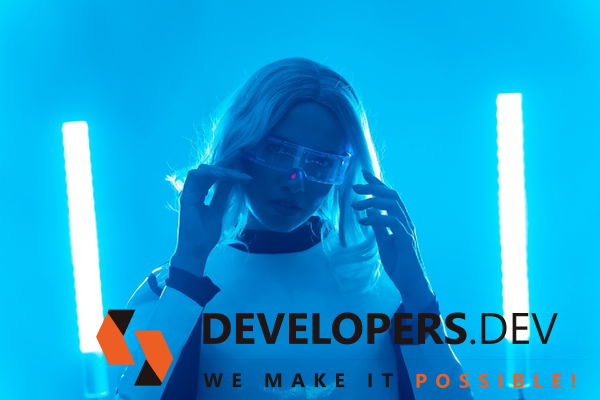
Thanks to technological developments driven by machine learning and artificial intelligence advancements, we now employ AI for game testing automation of a wide range of test cases for increased efficiency in video game production.
Before moving forward, lets define mobile game testing. Game testing refers to the practice of examining a videogame in order to produce high-quality products and maximize player satisfaction through error-free gameplay elements and improved user experiences - it plays an essential part in its overall success.
It should, therefore, never be taken for granted.
AI Vs. Conventional Game Testing Methods
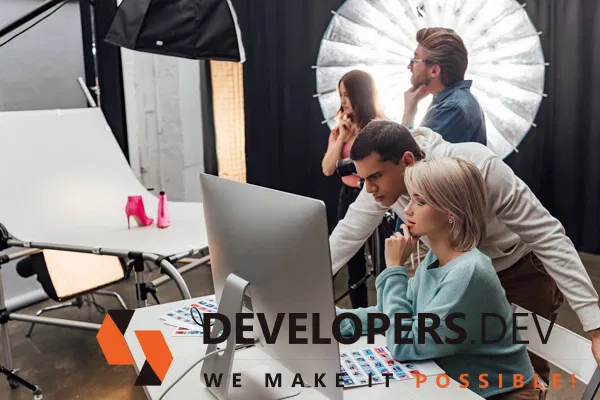
Video games have long used artificial intelligence (AI), yet human testing remains the predominant testing methodology.
Small games may be tested by their developer themselves or tested with friends and family, while larger titles might be given out to vendors to be played manually over several days or even weeks - however, AI is gradually replacing this conventional method of game testing.
Experiments Are Critical In Gaming
Testing games to ensure theyre both enjoyable and successful is integral to the game development process , yet its importance cannot be understated.
Testing takes more than just playing it in order to optimize stability and functionality while searching out bugs or faults. Game testers need to account for several variables when testing any given title - it involves considering all kinds of factors as part of improving its stability and functionality - rather than simply playing through its entirety.
The Reason Why Game Testing Is Important Is
Verifying that all aspects of a finished product function correctly allows developers to address troublesome areas for improved user experiences.
Testers help increase player enjoyment and enhance the quality of game function by testing early. Addressing bugs after release would be far more challenging and costly; testing helps avoid this costly situation altogether.
An Overview Of AI and How It Affects Game Testing
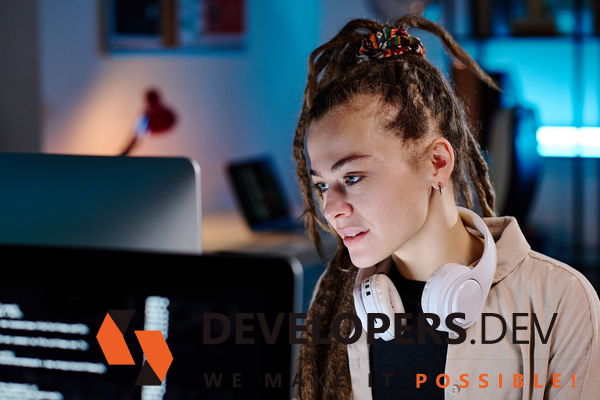
Artificial Intelligence, or AI, refers to the study and engineering behind creating intelligent devices, particularly computer programs with clever intelligence.
Although computers may attempt to replicate human intelligence using similar processes as found within biological means, AI extends further.
AI technology does not need to be limited by human intellect; thus, it can quickly outgrow all limitations. Artificial intelligence has already proven its worth across various industries, including gaming testing and production.
Its implementation for game testing helps automate and streamline procedures while increasing the speed, accuracy, and efficiency of testing procedures.
AI Systems For Video Game Testing
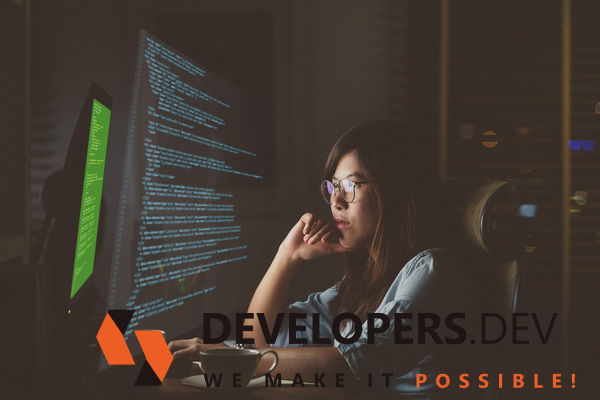
Gaming businesses have grown exponentially, necessitating sophisticated testing techniques in order to maintain game quality.
Artificial intelligence (AI) algorithms have become an invaluable asset when testing game mechanics - among their functions are evaluating player behavior, testing mechanics, and enhancing decision-making capabilities.
Lets examine how Artificial Intelligence (AI) algorithms are being applied in-game testing, particularly with regard to Machine Learning (ML), Neural Networks, and Natural Language Processing (NLP).
Machine Learning:
Machine Learning can assess player preferences and behavior to enhance user experience and optimize game design.
Machine learning algorithms may identify engaging game mechanisms from those that dont engage through evaluation of player data, as well as large volumes of gaming data to identify patterns or trends indicating issues or improvement opportunities.
Neural Networks:
Trained to recognize patterns and anticipate outcomes from large data sets, neural networks are powerful in forecasting player behavior and anticipating outcomes.
Furthermore, neural networks can optimize game dynamics while simultaneously detecting cheating, making gaming landscapes more realistic to enhance players gaming experiences, further enhancing this aspect of Neural Network technologys utility.
Natural Language Processing:
Natural Language Processing can enhance game engine and the overall user experience by helping developers detect language-specific issues as well as player review analysis to find repeat crashes or bugs, prioritizing fixes while simultaneously raising the overall quality standards of their games.
Want More Information About Our Services? Talk to Our Consultants!
Artificial Intelligence Tools For Testing Games
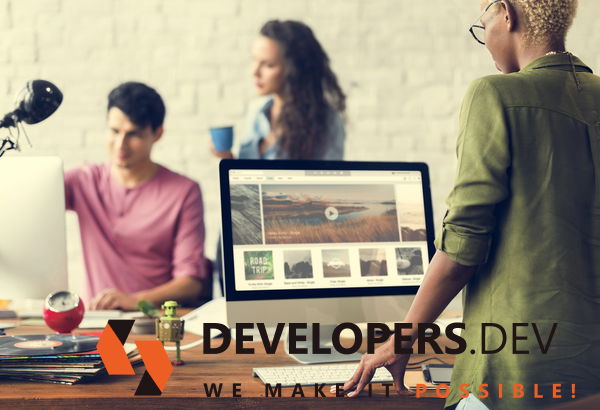
These AI-powered solutions for more efficient video game testing have been handpicked.
Gameanalytics:
This program takes advantage of machine learning algorithms to analyze player data and provide game tester jobs with key insights.
Test.Ai:
Test.ai is an AI-powered testing platform designed to automate video game testing and identify both functional and visual defects using artificial intelligence (AI).
Applitools:
This platform detects errors within the online game user interface (UI) using machine learning techniques.
Parchet Appsurify:
Parchet Appurify is another AI tool with this goal; it is used to automate testing to uncover bugs or performance problems faster.
Gamebench:
Gamebench is an AI-powered video analysis platform for game application creators that gives insights. VerSprite uses AI technology to find security flaws in video games and automate testing procedures.
Playtestcloud:
PlaytestCloud provides game designers with insights through machine learning algorithms that aggregate player feedback.
Deepmotion:
An AI-powered platform used for producing more natural and lifelike animations for video game genres.
The Advantages Of AI For Game Testing
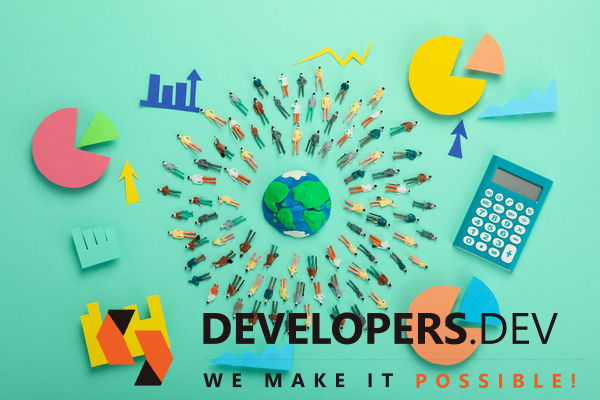
Enhance Efficiency:
Artificial intelligence programs like Test.ai and Applitools enable AI programs to automate repetitive operations much more rapidly than humans can.
Enhance Accuracy:
While humans can make errors, machines can accurately capture and interpret every piece of data collected by developers - saving even more of their time than manual testing could alone.
Reduce Time And Cost:
Correcting errors and post-release takes more time and resources; artificial intelligence allows game elements creators to save both expenses and time with this solution.
Increased Coverage:
AI allows game developers to explore a wider variety of environments than is humanly possible, giving gameplay mechanics makers greater coverage across every scenario or environment at once.
Enhancing Gaming Experience:
Artificial intelligence can assist game creators by pinpointing specific areas that need improvement to make gameplay simpler and more pleasurable for players.
Early Bug Detection:
AI technology can quickly spot potential multiplayer game production problems earlier, giving creators time to address any major ones before their release gets delayed or postponed.
Read More: Exploring the Evolution of AI in Video Game Design: A Journey Through History
AI Difficulties In Game Testing
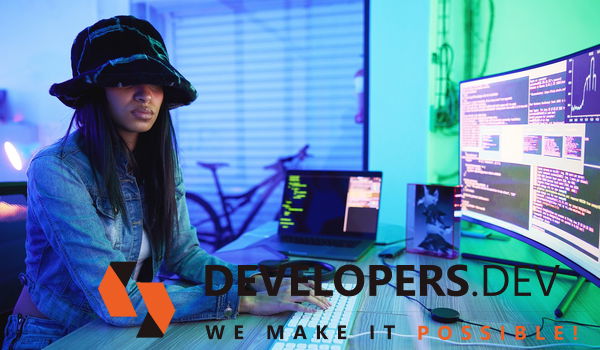
Artificial Intelligence Is Unbiased:
Artificial intelligence is designed to mimic human thinking without making judgment calls on its behalf; feedback about non cognitive and affective elements (type of games complexity, pace, or degree of engagement with gameplay experience) requires human testers.
Accurate Model Creation Can Be Complex:
In order for AI algorithms to be trained successfully, large amounts of training data need to be collected and utilized by AI functions; some algorithms may only have limited training data available, thereby making it difficult for AI systems to learn from.
Demand For Qualified Experts:
Due to an acute shortage of AI professionals with the necessary skill sets for designing and administering AI-based testing tools, game titles makers finding themselves challenged in using this technology for game testing is no simple matter.
Integration With Current Game Development Workflows:
Due to AI being such a new concept, game creators may find it challenging to combine their systems with AI algorithms.
AI Algorithms Have Their Limitations:
Although artificial intelligence has seen impressive advances, its capabilities still have some restrictions. For AI to work effectively in game testing services components such as gameplay testing, it requires too much human intuition.
Ethical Concerns:
AI algorithms could produce bias and have detrimental consequences for players depending on how theyre instructed.
How Will AI Affect Game Testing As We Advance?
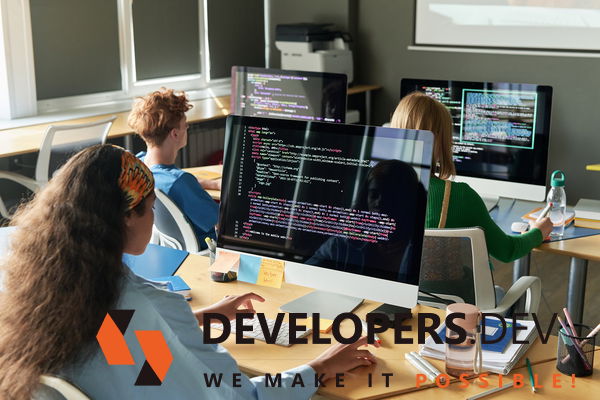
Recent years have witnessed immense advancements in the gaming business, as well as a rapid transition from conventional to AI-based procedures used for types of game testing or creation.
By making use of emerging technological breakthroughs, a more realistic and engaging gaming experience has become possible. Game console creators can focus on more intricate and imaginative aspects of their creation now that repetitive, time-consuming testing tasks have been automated.
AI can play an instrumental role in the creation of virtual and augmented reality console games that require extensive testing techniques and equipment; AI-powered testing instruments may assist developers by finding faults unique to these platforms so that players have a smooth and immersive experience.
Combining AI and cloud computing may aid scalability while making game development companies more affordable, providing testers with fast testing on multiple platforms without expensive infrastructure costs.
Google AI Is Revolutionizing Train Game Development
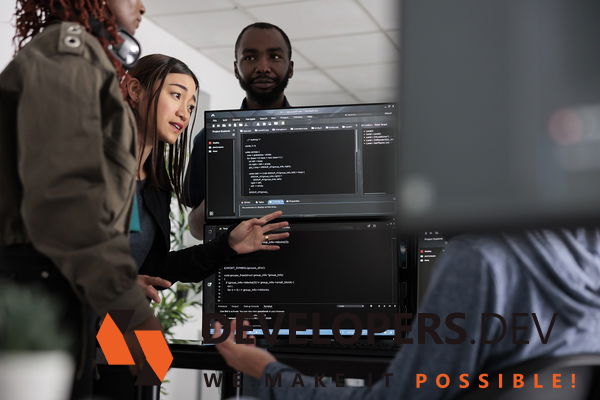
Google AIs breakthrough discovery in software engineering was DIDACT: an approach using big machine learning (ML) models to expedite software development efforts in their recent research project.
By collecting both finished product data and development process details, DIDACT distinguishes itself as it may change how programmers write, modify, and enhance code - an achievement worthy of praise by software engineering practitioners everywhere. Lets investigate this innovative technologys particulars to explore what impact its discovery holds for software engineering as an industry.
Software Excellence: An In-Depth Guide
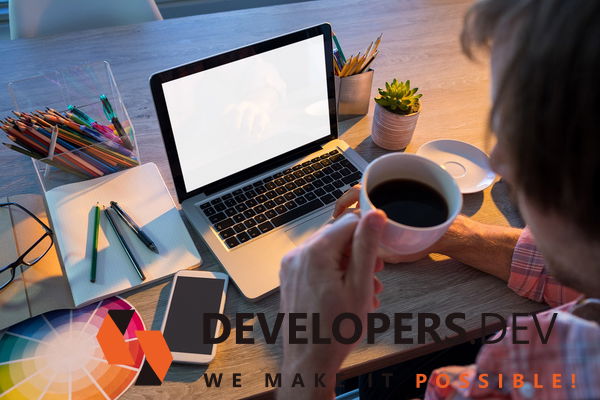
The development of software requires many iterative processes, from editing and testing through mistake correction and feedback incorporation to merging it with code repositories - each step aiding in strengthening its quality.
But thanks to Google AIs discovery, this intricate journey may now benefit from the efficiency of machine learning.
Making Use Of Googles Software Development Tools
Googles AI team takes advantage of Googles software development instrumentation to amplify developer activity data and offer meaningful recommendations that improve behavior when working on software engineering projects.
DIDACT may connect to thousands of actual developer interactions for analysis. As a result, its predictions provide software engineers with valuable advice while working on software engineering projects - ultimately with the aim of improving software engineering projects as a whole.
Unleashing Devscripts Potential
DIDACT provides a distinct methodology to manage various software engineering assignments. DIDACT enables job descriptions in an ordered fashion using "state-intent-action," an approach to task description that describes an action as the state, intent (such as code review comments or compiler errors), and subsequent actions taken against files.
DevScript serves as an autonomous scripting language that may be utilized for many functions, including error highlighting, variable renaming, code formatting, or commenting, among many others.
Unleashing Didacts Multimodal Potential
DIDACT stands out among similar products as it offers multimodal support to provide one-time assistance, yet is often surprised with unexpected abilities emerging unexpectedly - one notable aspect being history improvement, which allows suggestions based on past activities to become more accurate, especially useful when performing code completion tasks that utilize history augmented code completion where DIDACTs model makes more informed suggestions based on past edits.
Enabling Context-Aware Editing
Context plays an integral part in DIDACTs capabilities. For instance, when a developer deletes a function parameter from a function definition file, its historical context enables DIDACTs model to predict updates to related code sections, such as updating documentation strings or revising statements - without manual intervention and guaranteeing syntactical and semantic correctness.
Unleashing The Potential Of The Model
DIDACT holds even greater promise. For example, its model was trained to predict future modifications step-by-step and generate code from an empty file, much to the surprise of its programmer user.
Furthermore, its code generated was rationally constructed: first, an imports/main function structure was built, gradually growing over time to incorporate sophisticated filtering and writing/reading file functions - this shows DIDACTs outstanding ability to help developers at every step in the code generation process.
Conclusion
AI is rapidly revolutionizing everything, including game rules testing. Over the last several years, artificial intelligence (AI) has grown increasingly important within the game design document business - using systems like machine learning, natural language processing, and neural networks can save game developers both time and resources while improving efficiency while maintaining game quality and engagement levels.
AI will undoubtedly play a vital role in shaping its future development and testing environments as the gaming industry expands further.
DIDACT from Google AI could revolutionize software engineering through cutting-edge machine learning techniques.
By making use of real-world data and immersing machine learning models into the software development workflow, it provides insightful recommendations, boosts code quality, and allows developers to work more productively than ever.