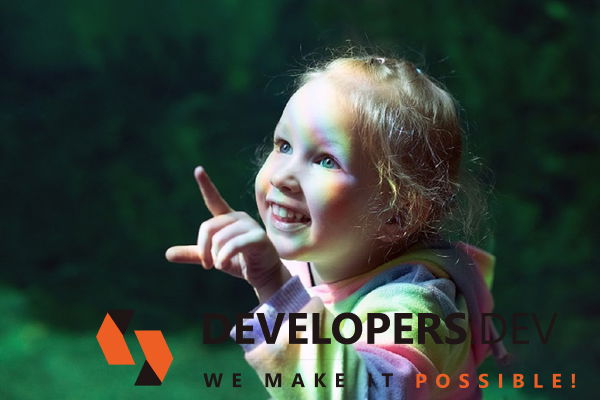
Volume is the total amount of structured and unstructured data that is collected. Velocity is the frequency of receiving the data.
Variety is the different formats, such as audio, video, text and numerical data. Value is the usefulness of the collected data.
Big Data does not necessarily refer to the quantity of data.
However, implementations that use big data typically include gigabytes or terabytes (or even zettabytes) of collected data over time. Companies are now using large datasets for a variety of purposes, including improving management, providing better customer support, generating targeted marketing campaigns and much more.
Big Data can provide businesses with valuable Consumer Analytics, which they can use to enhance marketing practices and strategies.
What Types Of Big Data Are There?
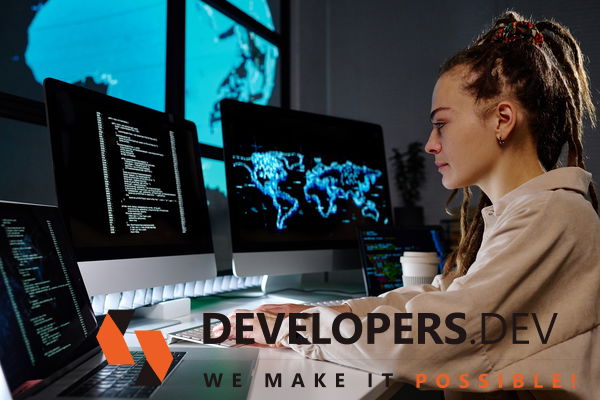
Structured Data
Data Structured is a standard format that has a clearly defined structure. The structured data is organized into a table, with columns and rows arranged in relationships.
Excel or SQL databases, for example, contain columns and rows of Structured data. For Structured Data, it is necessary to have a data model, which is a conceptualization of the way data is stored and accessed.
Each field can be accessed separately or with other information.
Semi-Structural Data
Semi-structured data is defined as information that cant be organized in Relational Databases or lacks an exact functional structure.
However, it has some structural characteristics. Semi-structured Data is information that has been grouped into topics or can be arranged in a hierarchy programming language.
This is structured data which does not have any table format or Data Models relating to Relational databases. Semi-structured information is often found in object-oriented databases that contain XML files, HTML documents and tables.
Semi-structured Data is good because its widely available. It can also be used for in-depth analysis.
Unstructured Data
Qualitative data, which is unstructured and has no structure or predetermined form, can be classified. Businesses receive huge volumes of Unstructured Data every day - audio, video, text and more - which are used to create massive Deep minimal Learning Models to solve complex real-world issues.
Unstructured data can be difficult to analyze and generate insights. This requires a lot of computational power.
Use No-Code Data Pipeline To Duplicate Data In Minutes
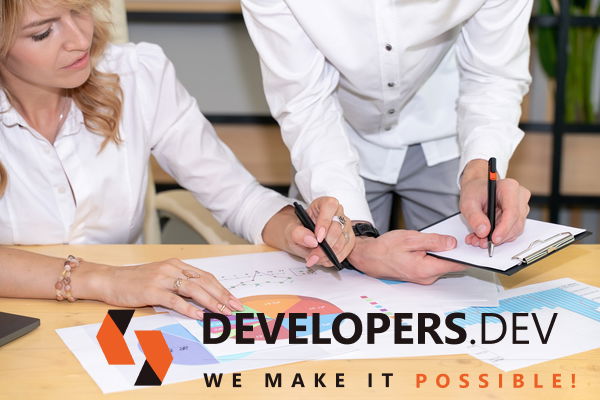
A Fully-managed Data Pipeline Platform can automate, streamline & enrich the data replication business process with just a few mouse clicks.
Big Data Processing refers to a collection of frameworks or methodologies that enable accessing enormous amounts of data and extracting valuable insights. Big Data Processing begins with data collection and cleaning. After youve gathered quality data, it can be used for Statistical Analysis and building Machine Learning Models for prediction.
Five Stages Of Big Data Processing
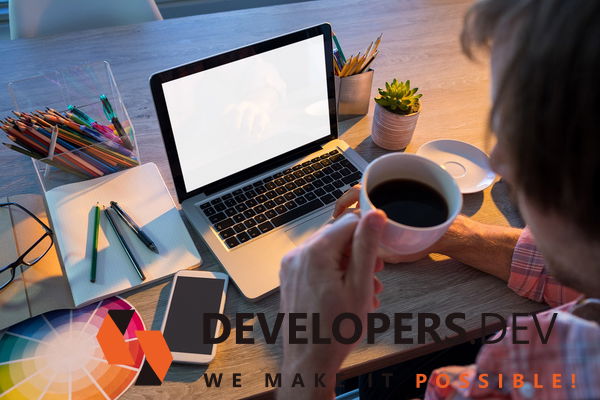
Data Extraction
The first step in Big Data Processing is to collect information using diverse sources such as enterprise applications, websites, sensors, marketing, transactional data, etc.
Professionals in data processing extract information from many Unstructured and Structured Streams.
In building a Data Warehouse, for example, the extraction process involves merging data from different sources and then verifying it by removing any incorrect information.
Data collected in the Big Data Processing phase must be accurate and labeled to make future decisions. The data collection phase establishes both a standard and a target for improvement.
Data Transformation
Data transformation is a phase in Big Data Processing that enables data to be transformed into the required format.
This allows for different visualizations and insights. Many transformation techniques are available, including Aggregation and Normalization, Feature selection, Clustering and Binning, as well as concept hierarchy generation.
These techniques are used by developers to transform Unstructured Data into Structured Data and Structured Data then into an easily understandable format.
The transformation makes business and analytical operations more efficient and allows firms to make data-driven decisions.
Data Loading
In the Big Data Processing load phase, the converted data are transported to a central database system. Index the database before loading data to improve the efficiency of the process.
The process of data loading was automated, defined, uniform, batch-driven, or real-time, using Big Data ETL.
Data Visualization/BI Analytics
Data Analytics methods and tools for Big Data Processing allow firms to create dashboards that provide a comprehensive view of their entire operations.
Business Intelligence Analytics (BI) answers questions about business strategy and growth. The BI tool makes predictions and analyses of what-ifs on transformed data to help stakeholders better understand data patterns and correlations.
Machine Learning Application
Machine Learning is the first phase in Big Data Processing. It is concerned with creating models which can adapt to new inputs.
Learning algorithms make it possible to analyze large quantities of data more quickly.
Supervised Learning is the first form of Machine Learning. It uses labeled data to train the models and predict the outcome.
In Supervised Learning, data patterns are utilized to determine new labels for information. It is used by applications which use historical data to predict the future. The second kind of Learning is called unsupervised.
In this case, the data are not labeled, but the algorithm is trained. Unsupervised Machine Learning uses the information without any labels.
Reinforcement learning is the last type of machine learning where there is no input data to be used as models. Algorithms must make decisions based solely on the observations and situations around them.
With a reward system, the algorithms are encouraged to make the right decisions. Machine Learning is a phase in Big Data Processing that allows automatic pattern recognition and feature extraction from unstructured data without human intervention.
This makes it a valuable resource for Big Data Research.
How Big Data Analytics Can Increase Productivity and Profits
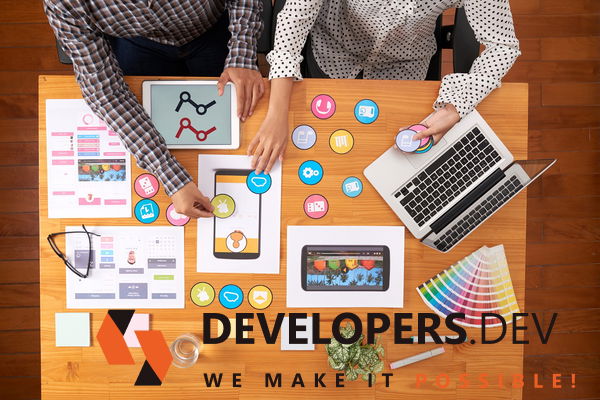
Were drowning in data, astronauts used a tiny fraction of data that was available on computers. Data is increasing exponentially.
Its expected to double in size every two years. The vast majority of digital data is lost at the corporate level. This can lead to valuable insights being lost, just 4% of businesses make effective use of data analysis - utilizing the right combination of people, tools and data to improve.
A report on workforce analysis summarized seven years of business research in the U.S. as follows: The Fortune 500 are investing billions in Big Data projects to predict future behavior rather than guessing accurately.
Analytics-based solutions are changing the way forward-thinking companies manage their employees to become more profitable and the production process."
Benefits of Business Data Analytics
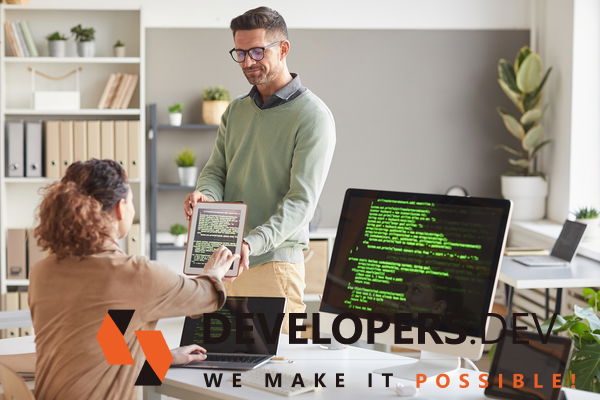
The Data Drive Continuous Performance Improvement
Data can have a major impact on team and individual performance. This data can help managers identify areas of improvement, and employees become more aware of their work behaviors and activities.
This data, rather than being a one-time training investment or seminar, can become an ongoing tool that will reveal new ways to improve as data continues to be collected.
Data Encourages Well-Informed Decision-Making
In situations of wide-ranging opinions or high emotions, data can provide a balance and back decisions up with solid evidence.
The more data you analyze, the better it is. The workforce performance report mentioned earlier points out that analytics engines become "smarter," as they connect actual results to data collected and do so "without biases or other limitations experienced by individual decision makers."
Data Enables Smarter Hiring Choices
Staffing is one area where data can be used to the employers advantage. Finding qualified employees can be difficult, particularly in industries with high levels of skill, such as technology innovation or healthcare.
This report describes how companies that are data-driven use algorithms to predict employee engagement help managers determine the best management practices, make hiring decisions, and much more.
Data-driven insights can be used to improve training methods after employees are already on board. Data can be used to improve training after employees have been hired.
This concept was put into action by a global company that analyzed internal data to find out why its employees werent meeting customer service goals.
The company changed its recruitment and training process based on the analysis. Within 12 months, they saw measurable improvements, such as an improvement of 5-10% in resolution rates for customer issues.
A single data-driven project can save between 5 and 12 million dollars, data-driven hiring and training methods can be used to help companies recruit the best talent. They will also gain insights on how they can improve performance. Data-driven methods of hiring and training produced benefits in this instance, including improved customer service as well as cost savings.
Data Delivers A Competitive Advantage
The use of data and analytics is a must in the workplace today. Thanks to the tools and technology available, its easier to do.
Companies that do not mine the value of their data risk being left behind by their forward-thinking and data-driven competition.
These companies are twice as likely to perform in the top quarter of their respective industries and five times faster to make decisions than other market participants.
Data Drives Revenue Gains
Most business strategies have one common goal: to increase profits. What can data do to help? Take a look to see what research has found.
Even small changes to data could have a big impact. The study revealed some interesting findings, including that the Fortune 1000 average company can: Increase revenue by over $2 billion in records per minute per year simply by improving data usability.
Increase the return on equity by 16% just by improving both data quality and salespeoples ability to access them by 10%.
By increasing the quality of the data, its usability and its accessibility by 10%, you can increase the return on your investment (which is equivalent to an additional $ 2.87 million in income).
Customers Acquisition and Retention
Suggestions are generated based on past purchases, products purchased by other customers, browsing habits, etc.
Promote Your Business With A Targeted And Focused Approach
Businesses can now deliver tailored product life cycles directly to the market they are targeting. No more massive promotional campaigns which fail to produce results.
By monitoring point-of-sale transactions and online purchases, businesses can use big data to analyze consumer trends. The insights gained are used to create targeted and focused campaigns to help brands meet customer expectations and to build brand loyalty.
Identifying Potential Risks
Risk management is essential for businesses that operate in environments with high levels of risk. The use of big data is essential for developing strategies and processes that are effective in risk management.
By optimizing complex process decision-making for unpredictable events and threats, big data analytics tools reduce risk by reducing it quickly.
Innovate
Big data analytics is the secret to driving innovation. You can update and innovate existing products and services using big data.
Data collected in large volumes helps companies identify their target audience. It is important to know what other people think about your product/service. These insights are also useful for improving marketing strategies, customer service and employee productivity.
Businesses must implement systems that track the reviews of customers, monitor product success, and keep an eye on competitors in todays highly competitive marketplace.
The real-time market tracking provided by big data analytics keeps businesses ahead of their competitors.
Complex Supplier Networks
Big data companies offer greater precision and insight to their supplier communities or B2B networks. Big data analytics can be used by suppliers to overcome the constraints that they face.
Cost Optimization
Big data tools such as Hadoop or Spark provide significant advantages in terms of cost for the storage, processing and analysis of large data volumes.
A logistics example demonstrates the cost-saving benefits of big data.
The cost of returning production challenges is typically 1.5 times more than the normal shipping charges. By calculating product return probabilities, companies use analytics and big data to reduce product return costs.
They can then take appropriate measures to reduce product return losses.
Improve Efficiency
Tools that use big data strategy can help improve your operational efficiency.
Your interaction with clients and the valuable feedback they provide will allow you to gather large quantities of customer information. The data can be analyzed to find patterns that are useful for creating customized products. These tools automate repetitive processes, freeing employees to focus on cognitive tasks.
Crossover Is Committed To Improving Workplace Productivity Through Data-Driven Technologies
WorkSmart, a solution for workforce management and productivity developed by Crossover, helps workers and managers access the data and analytics which drive improvements in performance at the team and individual levels.
WorkSmart is a data-driven personal productivity coach. The software measures data in many areas, including the time you spend switching between applications and surfing the internet.
WorkSmart is a coach that helps individuals build upon patterns of success to become more productive. WorkSmart provides insights into behaviors that need to be changed or avoided, such as spending too much time multitasking, checking email, or attending meetings.
This is information that employees rarely have access to but that businesses and managers desperately require.
Crossover, with its combination of global talent sourcing combined with effective productivity analyses powered by WorkSmart Data, regularly helps clients achieve productivity gains up to 50 per cent higher than their previous results.
Big Data Trends
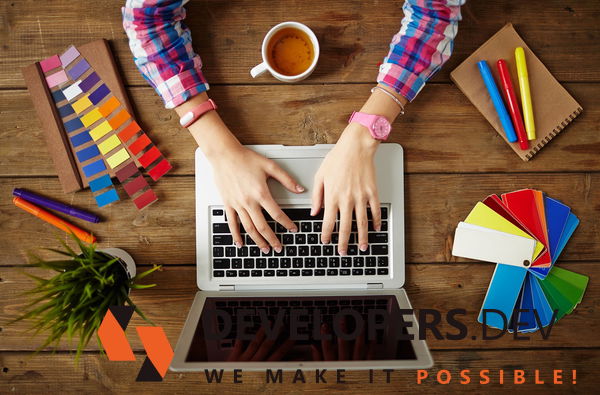
It feels as if a better term was missed when scientists refer to the origin of the universe by the phrase "the Big Bang".
Big data is no different. "Big" doesnt quite do it justice. This is not at all big, but its massive. It grows at an astonishing rate.
The 7 Vs, which are volume, variety of sources and velocity, as well as veracity, accuracy, value, variable, and visualization, will be the driving force behind a massive shift in technology.
Our experts examine the 23 Big Data Trends to help you shape your data strategies for this year and beyond.
Cloud Computing Can Be A Valuable Support Technology Cloud
Computing, and Big Data will be inseparable due to the rate and size of their expansion. Cloud computing is a major factor in the revolution of data.
Cloud computing gives us both the capability and scalability needed to deal with large quantities of data. Cloud computing is a cost-effective way to make big data available. Cloud computing is an important infrastructure for the wide-scale implementation of big data.
The Use Of Big Data To Drive Innovation Is A Growing Trend In Organizations Across Industries And Sizes
We can improve the operations of any business operation decision by collecting, using and exploiting data to its maximum extent.
Big data drives innovation in a wide range of industries, from manufacturing and transport to agriculture and beyond.
We can anticipate that the power of Big Data will improve all business decisions sectors in the future, regardless of their size. Smaller companies, and sometimes even sole traders, are forming data cooperatives or alliances in order to harness the power of Big Data.
The Internet Of Things Is One Of The Major Contributors
Big data is a key driver of this. Everything from your refrigerator to your car could be connected one day. You can do it right now.
The Internet of Things is a powerful tool for big data, as it allows real-time analysis and data collection.
This technology is now available to companies in the form of industrial internet. It allows them to gain insights that they could only dream of before.
Artificial Intelligence And Machine Learning Are Becoming More Complex As Big Data Analytics Become Increasingly Sophisticated
We will witness a relationship between artificial intelligence, machine learning, and big data as a result of a large amount of data.
Machine learning and AI are key themes to unlocking insights. This is the technology that allows us to use big data. We can use pattern recognition to identify ways of streamlining processes and boosting sales.
Predictive modeling is used to help us prepare for future uncertainty. Real-time analytics helps us analyze the data and get the insight we require right when we need them.
We will continue to develop artificial intelligence (AI) and machine-learning tools as big data increases. Both will grow as the first does.
Data Is No Longer Limited To What We Think Of As Data
Variety is one of the seven Vs of Big Data. Were now seeing a trend where the types of acceptable data are increasing and becoming more diverse.
The traditional data that is stored in databases consists of words and numbers, which are arranged in files and columns. Big data is a new way to think about structured data.
The data that is considered big data may be in a variety of formats, from unstructured to structured, such as audio, video, and image files.
It can also include sensor logs, social media data, and more. Our infrastructure must grow in order to cope with the increasing diversity and volume of data that we will be generating.
Data Storage And Processing Will Be Improved With The Advent Of Big Data
What is the difference between data lakes, data warehouses and databases? Everybody has heard about a database. A database is a way to store traditional data.
There are databases for products or customers where all information is stored. The database is designed to allow for data movement and flexibility so that you can get the specifics from it. Data warehouses are a type of database that is used to report and analyze data.
Data lakes are designed to handle a variety of data formats, and big data is no exception. This is a new way to store data that can handle unstructured data of any format.
Read More: Utilize Big Data Analytics Tools
Increased Efficiency And Improved Decision-Making
We can gain big insights from huge datasets. We can spot emerging market trends with the help of precise data on changing customer behavior and market conditions, often at a global level.
The use of big data allows companies to predict the impact of their actions and offer a better experience for customers based on the knowledge that they have accumulated from previous interactions.
The use of big data can also help companies to improve their accuracy by allowing them to base their decisions on larger samples.
It promotes improved risk management, and it helps with agility.
Predictive Analytics Can Use Big Data To Help Businesses Anticipate Future Events And Trends
Companies can use big data to perform predictive analysis. This includes historical data in its many forms and variations, machine-learning algorithms and statistical modeling.
Organizations can improve their efficiency and competitiveness by incorporating predictive analytics into big data.
Big Data Can Be Used For Sentiment Analysis To Help Businesses Understand The Opinions Of Their Customers
Sentiment analysis is a major problem when artificial intelligence performs customer interaction. Even computers can have difficulty identifying and conveying things such as tone of voice.
This is especially true when writing. Machines cannot experience or feel emotions or sentiments the way humans do, which can cause communication breakdowns.
The use of big data can be a powerful tool to understand better and improve this problem. Although it seems impossible that a computer will be able to analyze sentiment consistently, the reality of this is closer than ever.
Want More Information About Our Services? Talk to Our Consultants!
Conclusion
This article will teach you about the characteristics of big data solution.
The use of big data has been gaining popularity, and the tools that are used to analyze data in organizations play an important role. In particular, the use of tools for Big Data in storing, processing, and analyzing data, has transformed data discovery processes.
Organizations use a number of data sources to collect a wide variety of useful information. Transferring data into a Data Warehouse to perform a comprehensive analysis can be a difficult task.
You will need to maintain and code complex functions in order to achieve an efficient flow of data.