
Data growth has exponentially accelerated with digital technologies like mobility, connectivity, the Internet of Things and artificial intelligence (AI).
New big data tools will assist businesses in collecting, analyzing and processing the increased amounts of information faster to maximize its value for them.
What Is Big Datas Significance For Businesses?
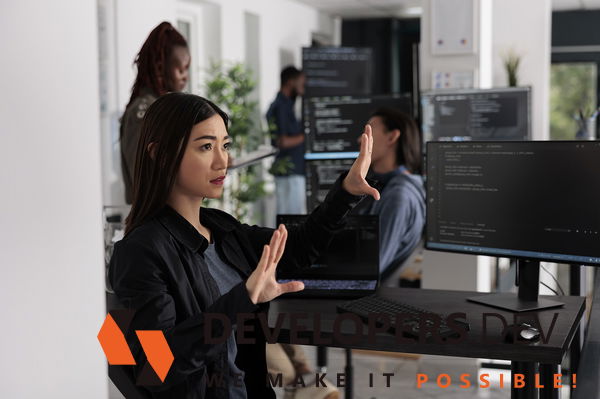
Businesses can utilize the big data analytics collected in their system for various uses, including improving operations, providing better customer service and creating targeted marketing campaigns.
Firms that can effectively utilize big data have an edge over their rivals as they can make quicker and more intelligent business decisions thanks to this data resource.
Big data offers businesses invaluable insight into their customers, which they can leverage to enhance marketing, advertising and promotions, customer engagement, and boost conversion rates.
Both historical and present-day data sets may help identify changing preferences among corporate or consumer buyers; this allows companies to better respond to customers needs.
Medical researchers and doctors utilize big data analytics tools for medical diagnosis.
Through information gleaned from social media platforms, electronic health records, the internet and other sources, big data provides government healthcare agencies with timely, precise insight about infectious diseases that could impact public health policies.
Organizations Utilize Big Data In Different Ways
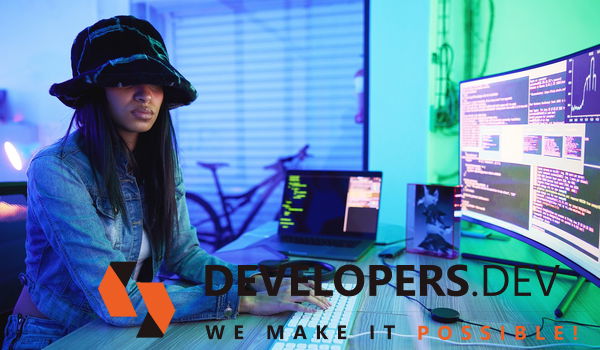
Energy providers leverage big data to identify drilling sites and monitor pipeline activities; utilities also employ it for tracking electrical grids.
Big data also allows financial services firms to observe risks while analyzing market information in real-time.
Big data is being leveraged by manufacturers and transport companies to optimize delivery routes, manage supply chains, implement emergency responses/crime prevention initiatives and create intelligent cities.
Meanwhile, the government has used big data for emergency responses/crime prevention efforts and urban renewal plans/intelligent cities initiatives.
What Is Big Data?
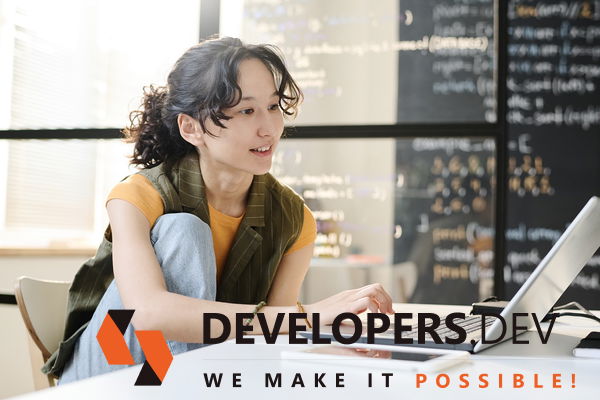
Integration: Big data refers to extensive collections of unstructured information which could span across several terabytes or more significant in volume from various sources and requires extensive processing to be put in a format usable by business analysts and users for analysis.
Management: Big Data needs massive storage solutions on-premises or in the cloud to accommodate vast amounts of information and processing in real-time, regardless of form.
Companies increasingly turn to cloud solutions to leverage unlimited computing power and scalability.
Analyze: The final step in analyzing big data and taking action on it involves acting upon it.
Otherwise, your investment would prove futile. Therefore, its essential not only to explore but also to communicate insights in an easy-to-understand format using tools like charts, graphs and dashboards.
Big Data Examples
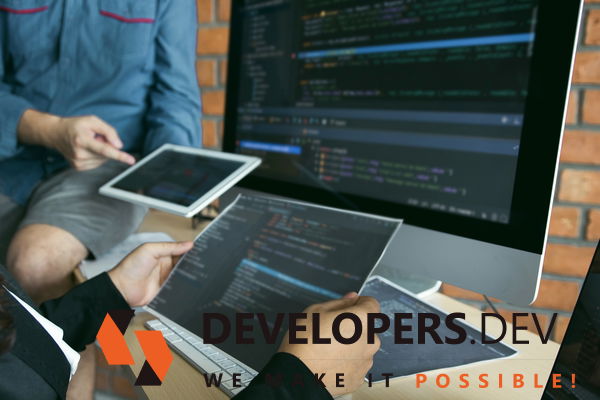
This can take many forms; examples may include transaction processing systems (TPS), customer databases, email, medical records and clickstream logs from the internet, as well as logs generated by servers and networks in addition to sensor data from industrial equipment, manufacturing machines or Internet of Things devices.
Big data environments encompass external information on consumers, financial markets, weather and traffic conditions, geographical details and scientific studies, and internal files such as images, audio or video files.
Many streaming data applications also utilize big data applications in this manner for real-time collection and processing of data streams.
Big Data: More Characteristics
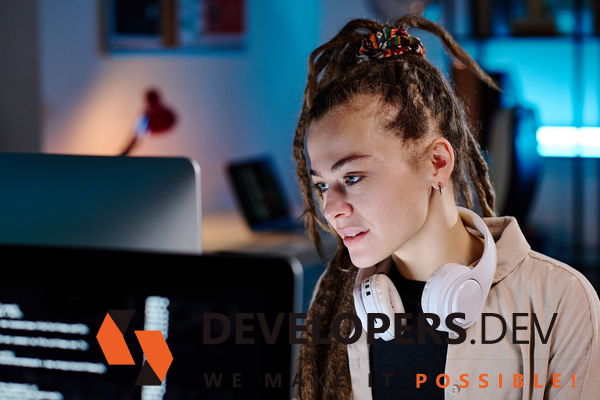
Veracity in data refers to its accuracy and trustworthiness; issues can arise due to raw data coming from various sources that are difficult or impossible to spot; insufficient data could potentially cause errors during analysis if left untouched through cleaning; the team responsible for data management must also ensure they possess enough accurate information to generate valid results.
Data scientists, consultants and other specialists may also prove invaluable. Data collected may have no real meaning or application to your business - before using big data analytics applications techniques, all collected information must pertain directly to business-relevant issues.
Big data sets exhibit variability. Their meaning may change over time, or they might contradict each other - complicating management and analytics processes.
Read more - What is Big Data? Types & Main users of Big Data
What Is The Process Of Storing And Processing Big Data?
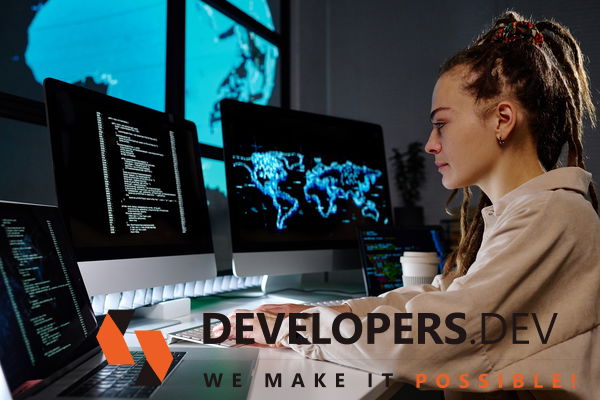
Data lakes are commonly utilized as storage solutions for large volumes of data.
Data lakes do not limit themselves to structured data alone and often use relational databases when building them - they also support different data types that need storage solutions.
Big data environments often combine various platforms in an architectural model to optimize ample data storage and retrieval.
Relational databases and warehouses, for instance, may connect to one central data lake for analytics systems; data stored raw is then organized or filtered as necessary by advanced analytics, analytics features programs; sometimes, however, it must first go through data-mining programs before being ready for regular applications.
Big data processing places immense strain on computing infrastructure. Clustered systems which distribute workload across thousands of commodity servers with Hadoop or Spark as processing engines often come under pressure when providing the processing power needed.
Finding affordable processing power to analyze big data projects can be challenging.cloud platform vendors, Cloud-based extensive data systems offer organizations a solution at a reasonable price: organizations can deploy their own or use managed cloud services costs offerings from managed providers; users have the flexibility of scaling their servers up and down as necessary while only being charged when their instances are needed for analysis projects.
Big Data Analytics: What It Is And How It Works
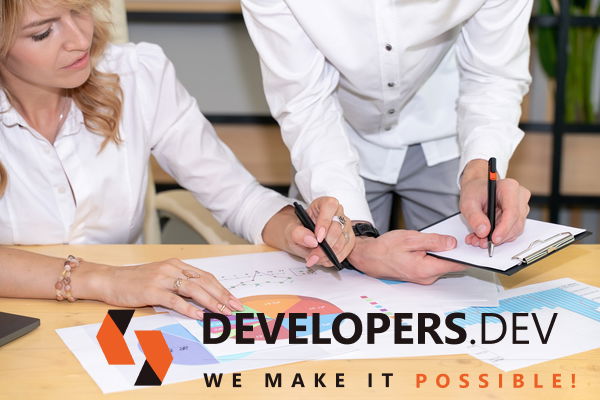
Data scientists and analysts need a complete knowledge of available data and what their analysis seeks, as well as any required preparation work before beginning analysis processes such as profiling, cleaning, validating or transforming that information.
This step must also take place as early as possible during analytics initiatives project development processes to get the maximum benefit out of every analytical exercise performed on it.
Once data collection is completed, it can be utilized in many different applications using features of big data analytics professionals to perform various applications involving predictive modeling, machine learning, and its deep learning variant.
Here Is An Illustration Of Some Of The Analyses Possible With Big Data
Comparison analysis: Examines customer behavior metrics, real-time engagement with customers and brand recognition in order to compare products and services offered by one business with those provided by competitors.
Examining Social Media: This practice allows businesses and products to analyze what users are posting about them on social media platforms such as Twitter or Facebook and identify any problems while at the same time helping target marketing campaigns more precisely.
Analysis of Customer Sentiment: Data on customers is examined to ascertain their opinion of brands/companies they interact with, their satisfaction level with services provided to them and potential issues which might exist, as well as ways in which customer services could be improved.
The Big Data Challenge
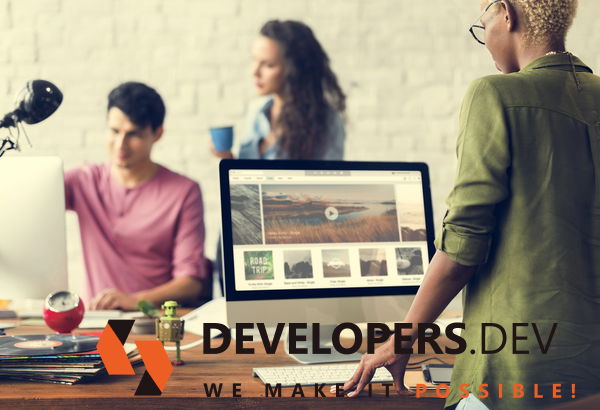
Designing significant data architecture: can present users with numerous processing power issues and be brutal.
Tailoring systems to meet organizational requirements involves IT teams and data managers gathering specialized technologies and tools and designing an organization-specific Big Data environment. Furthermore, the skills required for managing such extensive data systems differ considerably from those needed by database administrators or developers working on relational software platforms.
Cloud services can offer solutions for both issues; however, managers must monitor costs carefully to prevent charges from spiraling out of control.
Migrating data and workloads from on-premise systems to cloud applications, cloud users, platforms may prove complex; managers should entrust this task solely to professionals for maximum success.
Managing large data sets: also presents unique challenges when making them available for analysts and scientists, especially in environments with various platforms and data sources.
Data management teams and analysts are creating catalogs with relevant data in them that make finding relevant pieces easy; some even feature metadata management functions and lineages! Integrating Big Data sets may prove more complex due to data velocity or a variety of factors;
Data talent and skill shortage: The IT industry faces a talent and skill deficit for data scientists, analysts and engineers in particular - highly paid professionals sought-after across sectors due to the demand.
Unfortunately, however, limited experience or skills with big data technologies prevent maximizing datas potential value.
Speed of growth: By its very nature, big data is constantly shifting and expanding; without an infrastructure capable of handling its storage, processing, networking, and security needs efficiently and successfully, managing it may prove challenging.
Data Quality Issues: Data quality has an immediate effect on decision-making processes, analytics software, analytics technologies and strategy planning methods.
Raw data can be messy and complicated to curate, while big data only guarantees success with accurate, relevant and organized analysis data that fits this bill. Failing to address it may result in misleading insights, leading to costly insights with zero valuable results.
Complexity of Integration: Data can often become siloed across an organization.
Merging disparate sources into accessible formats requires skilled hands to reap any returns from big data Real-Time analytics.
Concerns About Security Regarding significant data security: hackers tend to target it for the valuable information it holds about businesses and customers.
Yet, datasets can often be complex and diverse, preventing comprehensive policies or strategies from being implemented to secure them properly.
Big Data: The Keys To A Successful Strategy
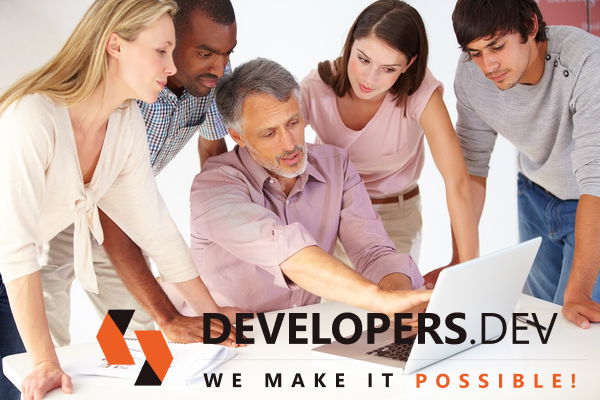
An effective big data strategy requires understanding business goals, the available data sets and whether any additional information may be needed in order to meet goals.
The following steps for organizations include the following: Prioritize use cases and applications; Establish tools and systems needed; Develop an implementation roadmap; Assess internal skill set and determine if any need retraining or hiring.
As part of an effective data governance and quality management system, prioritizing Data Governance and Quality Management processes should ensure big data sets remain uniform, clean and adequately utilized.
When considering practices for extensive data analysis and management, business requirements over available technologies are often best used; data visualization also offers ample potential.
Regulations And Practices For The Collection Of Big Data
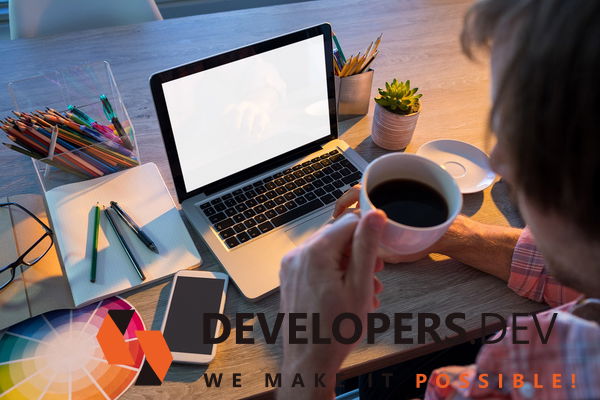
Data collection and use have increased exponentially over time, along with its associated risks.
Responding to public displeasure over data breaches and privacy concerns in general, European Union legislators approved the General Data Protection Regulation ( GDPR), which became law on May 18 2018. Under GDPR, organizations can only collect certain kinds of personal information with permission and for specific reasons outlined.
Furthermore, it includes an opt-out provision which lets EU residents ask companies for deletion.
California Consumer Privacy Act (CCPA) is a new state-level privacy law designed to give California residents greater control over how their information is collected and utilized by businesses within its borders.
CCPA became the rule effective January 1, 2020. Businesses must monitor the collection of big data business analytics closely in order to comply with relevant laws, while controls should also be put in place in order to secure any sensitive or confidential data which would allow unauthorized employees access.
Big Data: A History
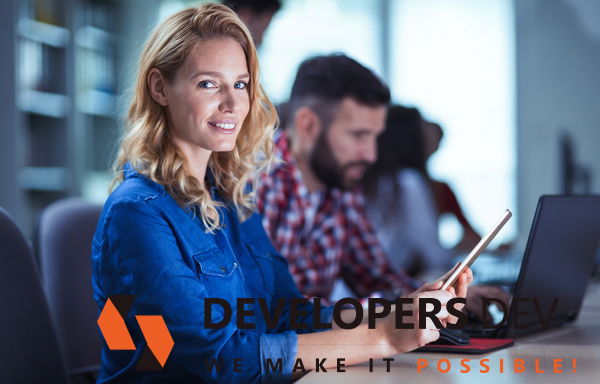
John Grant had to navigate an immense volume of data while investigating Europes bubonic epidemic in 1663 - it marked the very first instance of Big Data analysis! Grant pioneered statistical data analysis during this era; 18th Century statistics extended further with collection and analysis as an additional focus, finally becoming widely aware in 1880 when there was an abundance of it all around.
The United States Census Bureau estimated that managing and processing data collected during its 1880 census would take eight years.
Hence, Herman Hollerith built his Hollerith Tabulating Machine, which allowed him to cut back the calculations needed drastically. By 1881, a Hollerith Tabulating Machine had already reduced the measures required. Meanwhile, in the 20th Century, big data saw rapid expansion; computers, scanners for message patterns, and magnetic storage devices were developed, while in 1965, for tax returns storage and millions of fingerprint sets being stored at once, the US government built their first data center!
Big Data Has Become A Buzzword Over Time
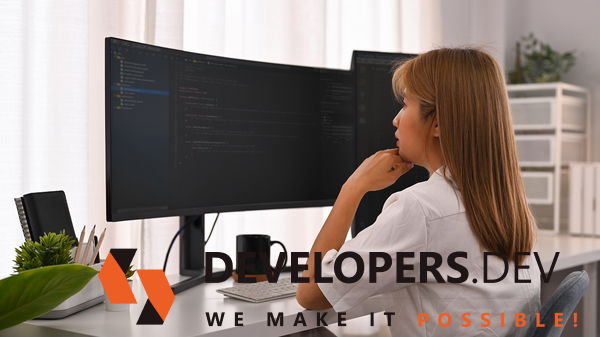
Gartner defines Big Data as, "big volumes, fast velocity or wide variety information assets which require new processing frameworks methods in order to enhance decision-making, insights discovery and stream processing engines complex process optimization".
Lets dive deeper and simplify this concept further.
Big data refers to large data sets which exceed what can be processed using traditional computer techniques and are therefore inaccessible through normal means of analysis.
Not just the physical data itself is affected as well as tools and frameworks. Social media channels, as well as devices able to handle more powerful computing, have presented industry players with new challenges when taking big data sets.
Since 2003, Earth has only produced five gigabytes of data since its origins. That amount was generated every two days in 2011 and 10 minutes daily by 2013! Thus, it should come as no surprise that 90% of global data production occurred only over recent years.
Once processed, this data can be highly beneficial; unfortunately, before the term big data was coined and adopted widely used to be ignored or forgotten about altogether.
Professional training in Big Data will enable you to enter the Data Science sector, including technologies like Impala and Cassandra.
Big Data: The three Vs
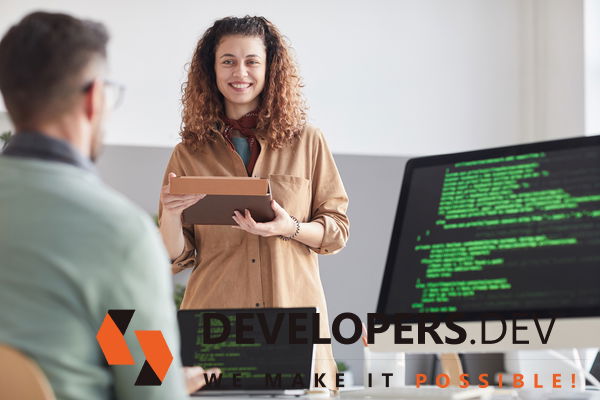
Volume
Lets begin with one of the more obvious points. Quantity is vital when discussing big data, with volumes reaching unimaginable heights.
According to estimates, there will be over 40 zettabytes by 2020, representing an exponential rise since 2005. Terabytes or Petabytes worth of information stored on servers or storage can then be utilized by companies to monitor success and shape future endeavors.
Velocity
Data has evolved our understanding of its significance and expansion over time. Although once underestimated in business settings, its usage requires us to rely heavily on it.
Velocity measures how quickly data enters a system - this may vary between large batches being delivered simultaneously with intermittent streams being received intermittently - due to different systems handling it differently, its wise to refrain from making assumptions until all necessary details have been acquired before making assumptions based on inadequate knowledge alone.
Variety
Data was once only accessible in one format and from one source, typically delivered as traditional file types like Excel, CSV or Access files.
Now, data can come through wearable devices or social media sources in less-than-traditional forms like video, text and PDF documents. This non-traditional data requires additional work regarding analytic skills to interpret, manage and utilize.
Want More Information About Our Services? Talk to Our Consultants!
Big Data And Its Implications
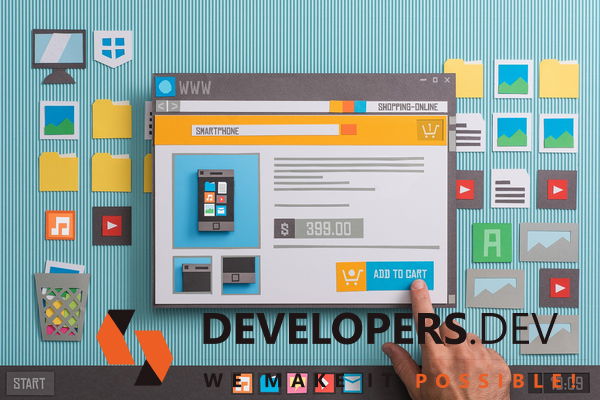
With the proliferation of social media, apps, and online businesses has come an increase in data collection at an astounding rate - social media sites alone reach millions daily! Data collection has thus increased dramatically, but how is all this data to be stored, processed, managed or handled efficiently? That is where Big Data enters into play.
Big data analytics has transformed IT fields, adding immense value and expanding organizations.
Utilizing cutting-edge tech like machine learning, data mining, statistics, and analytics modeling , analytics platform, analytics findings, analytics platform provider, analytics products BIg Data can enable organizations and teams to perform multiple functions using a single platform - including storing Tbs of information before processing velocity and analyzing it all simultaneously.
Big Data
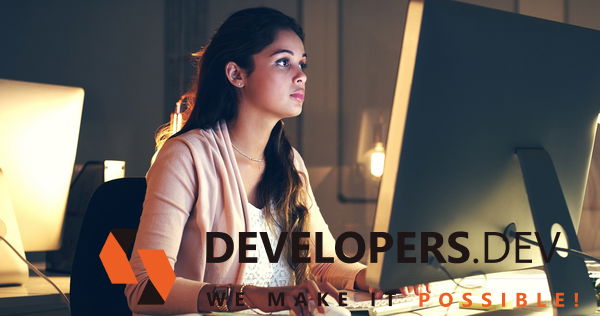
Analytics programs of big data require the identification of trends, patterns and correlations among vast quantities of raw data to make data-driven decisions.
Methods exist that use traditional statistical analyses, such as clustering and regression in large datasets using more modern instruments.
Data Collection
Each big data company takes a different approach to collecting its data differently.
With modern technologys support, businesses can collect both unstructured and structured information from many other sources, including cloud object storage services, mobile apps and IoT sensors in stores - creating more granular datasets to inform business decisions.
Arrange Your Data
To achieve accurate analytical queries, its vitally important that once gathered, data be adequately organized - particularly if stored as unstructured volumes of unsorted information.
Clean Data
To increase data quality, all information must be cleaned. Incorrect or unnecessary details need to be eliminated, while any duplicate or redundant records should also be eliminated from consideration.
Poorly cleaned or misclassified information can conceal hidden realities or lead to false conclusions; any dirty data must, therefore, be reviewed thoroughly before analysis and removal from consideration.
Read More - What Is Big Data? Why Is It Important Today?
Big Data Types (With Examples)
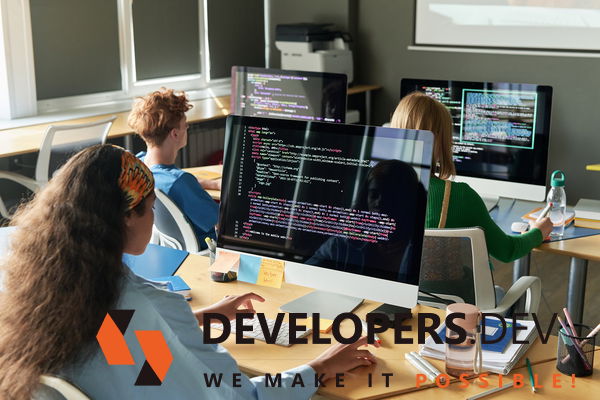
Structured Data
Structured data makes sorting and assessing easier because its form has already been determined.
It provides each field with its schema or structure that makes accessing individual areas or groups of fields simpler. Structured data has become essential due to its speedy collection from multiple database locations.
Unstructured Data
Unstructured data refers to information without predefined concepts that are hard for humans or conventional databases or predictive analytics, predictive modeling, machine learning model, statistical models, pricing models to make sense of, making analysis harder than necessary.
Typical forms of unstructured data include facts, numbers and dates. In contrast, big data provides video/audio files plus mobile activity monitor data as well as satellite image captures from mobile phones, etc.
Semi-Structured Data
Semi-structured data combines features and characteristics associated with both structured and unstructured information; it shares some organizational structures with structured data but lacks its strict form; examples of semi-structured data could include JSON or XML documents.
Characteristics Of Big Data
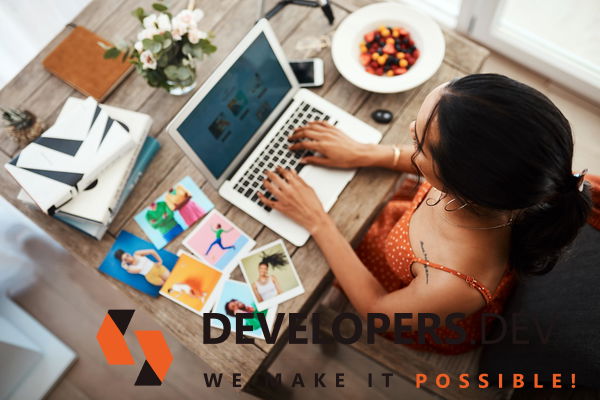
- Big data refers to data sets with extreme volumes that increase rapidly and span vast territories.
- Big Data Analytics techniques encompasses numerous applications - social media platforms, airplane engines and financial markets - providing opportunities to analyze large volumes of data.
- Big data can be divided into unstructured or semi-structured categories.
- Several features, including volume, variety, velocity and variability, can define Big data.
- Big data offers immense opportunities for improving customer service and operational efficiencies.
Big Data Use Cases
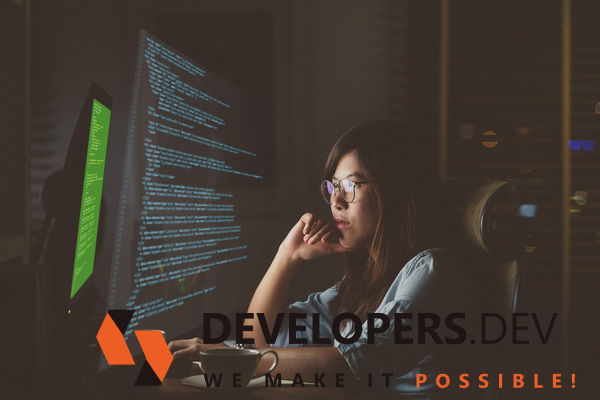
360° View Of The Product
Businesses use big data to develop dashboards that offer customers a 360-degree perspective on them.
The dashboards collect and evaluate information from both internal and external variety of sources before providing it in such a way as to assist their customer service, marketing, or sales departments with their work.
Enhancing Client Retention And Acquisition
Businesses can utilize big data analytics external data sources to better understand their clients interests, patterns of usage and reasons they stop purchasing their products or services.
Big data apps allow businesses to comprehend customer behavior better - helping companies better understand what customers desire from products/services while using these patterns to optimize outcomes for more excellent conversion and retention rates.
Businesses Are In A Constant Battle Against Fraud
Organizations are leveraging big data analytics projects, analytics vendors, business analytics initiatives, supply chain analytics, approach to analytics, branches of analytics, channel analytics to detect trends of fraudulent activities, abnormal system behaviors and criminals committing them.
Extensive data systems can scour data from servers, databases, and apps to detect, deter and minimize such criminal acts.
Improved Forecasting And Pricing Optimization
Big data may not be able to forecast or optimize pricing decisions in advance accurately; however, it enables businesses to recognize patterns and trends early.
When problems emerge early enough in supply chains, companies can adjust them immediately, while information on demand helps sales forecasting improve and determine optimal product prices prior to release. Big data allowed these organizations to make better decisions as it provided probabilistic analyses of the potential outcomes of specific outcomes.
Conclusion
Consumers today are highly demanding. They use social media to connect with other customers and consider different options; customers seek recognition after purchasing an item; big data offers actionable information that enables you to engage your customers immediately by checking customer profiles in real-time as well as receiving data regarding any complaints made against your product/service, then performing reputation management with this data.