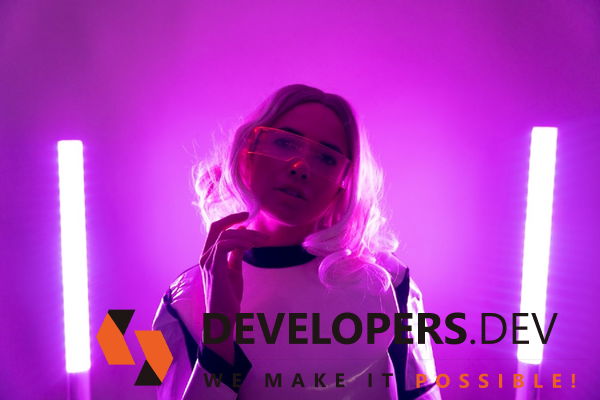
This is an example where translating doesnt necessarily mean that it is what it is. Data size is insufficient to determine whether something is appropriate for the strata.
What is excellent for one person may only work for one person. When certain aspects are combined, and the needs have been met in a database? Information is considered to be in this classification.
Although a large quantity of data is crucial, there are other prerequisites. Other factors to consider include how much data is growing over time, how quickly it happens, how diverse and accurate it is, how easily it can be categorized as authenticity and reliability of data, and whether it can be used to generate scenario-switching.
Big data can be described as a mixture of semi structured, organized and unorganized data that is banked by the corporation and can be used to mine for information in machine learning tasks and predictive modeling, together with modern analytics applications.
Extensive data knowledge management systems are now a standard element of data management structure within corporations.
They can also be used together with equipment that favors big data analytics. Big-data analytics. The 3 Vs are often used to describe big data:
- Humongous data quantity in all dynamic environments.
- The extensive range of data types that are stocked in large data banks.
- Velocity is the point at which most data is developed and collected.
The above attributes were recognized in 2001 when he was an analyst at Meta Group Inc. Gartner made them more popular after it bought Meta Group Inc.
in 2005. Several other Vs were added to various descriptions of big data, including integrity and value.
While big data does not refer to a specific volume of data, large data deployments often include terabytes or petabytes of data collected over time.
Advancement of Data Size
A vast database can contain everything your company has done over the years. It is not surprising that the volume of data is so large.
However, the importance of data will grow over time due to the addition of sales, billings, inventory, expenses and revenues. This would have little effect on the total volume but, most importantly, the nature of the data. This is why we cant classify the data.
If the amount of information you have added is significant in absolute terms and can alter the overall profile of the data, then we can accept that other foremost specifications have been fulfilled.
The bank can become it. Imagine the external data sources volume and speed of data growth that Google, Facebook and other social media sites have daily.
Although these, this is more or less what we are talking about.
Persona & Diversification of Data
You must understand that modern key technology and the world we live in produce vast amounts of Databases that were initially intended to store and classified into well-defined, narrow categories.
Anyone who designed a database to help organize inventory or billing tables had no difficulty.
The zestful, variable content? A Facebook profile is very personal and interactive social media content. People share text, images, and videos, and we are still determining what else might be coming soon! How does Facebook or any other company dealing with this reality classify every information posted by its over 2.3 billion users?
This is an example of the complexity and variety of massive amounts of data that requires new ways to store, organize, manipulate, and interpret information to make future decisions with key features.
Information Authenticity
To be authentic, you must first define yourself as your person. Its the exact same thing in extensive data services.
Does data integrity security exist? Is the data being provided by the source secure? Is the data safe?
It is essential to ensure that all data collected is protected by reliable mechanisms. They are resistant to data assemblage, communication, classification and sophisticated data deposits.
They are also not susceptible to being tampered with by any means. And is acceptable, as incorrect conclusions can be drawn from false data. A good example? Think about it.
Relevance
Every information in data banks must be necessary, in an understandable form. It must serve a motive or an aim. A "database" is simply a data collection with no value.
This is not unusual. However, the results of various databases intended for different purposes show that a lot of deposited data has no station.
They may be insensitive data, or any analysis cannot change them.
The data must be able to modify, alter, reveal things and be authentic to a significant pace to specific business users and types of agile decisions-making.
An example of this is the NASA probe launch. Knowing that there will be a severe solar storm on the day of the launch is data of great relevance to vision victory.
Want More Information About Our Services? Talk to Our Consultants!
What is the Significance of Big Data?
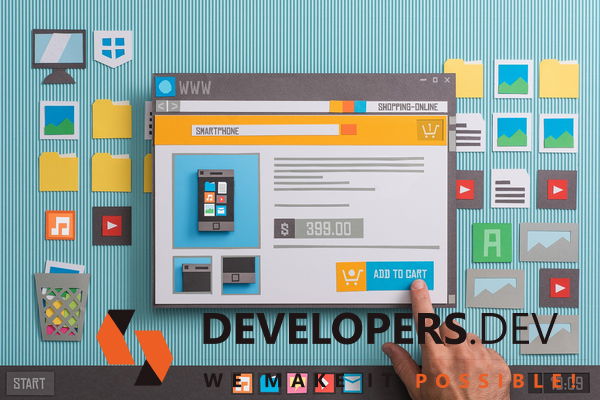
Companies know the importance of big data analytics and utilize big data in their systems for improved operations, better customer service, personalized marketing campaigns, and other actions that can ultimately increase revenue and profit.
It can give businesses an edge over others who dont use it because they can make better business decisions and act faster.
Big data, for example, can provide valuable insight into customers that companies can use to improve their marketing, advertising, and promotions to increase customer engagement.
Historical and real-time data can be analyzed to determine the changing preferences of corporate buyers and consumers, allowing businesses to respond more quickly to their customers needs.
Medical researchers also use big data to identify risk factors and disease signs. Doctors use it to diagnose and treat patients with medical conditions and illnesses.
A combination of data from social media sites, electronic health records, and the internet provides healthcare agencies and government agencies with up-to-date information about infectious disease outbreaks and threats.
These are just a few examples of big data being used by organizations:
- Oil and gas companies use big data to identify potential drilling sites and monitor pipeline operations. Utilities use it to track the electrical grids.
- Financial services companies use extensive data systems for risk management and real-time market data analysis.
- Big data is used by manufacturers and transport companies to optimize their supply chains and delivery routes.
- Other government uses include emergency response, crime prevention, and innovative city analytics initiatives.
What are Some Examples of Big Data?
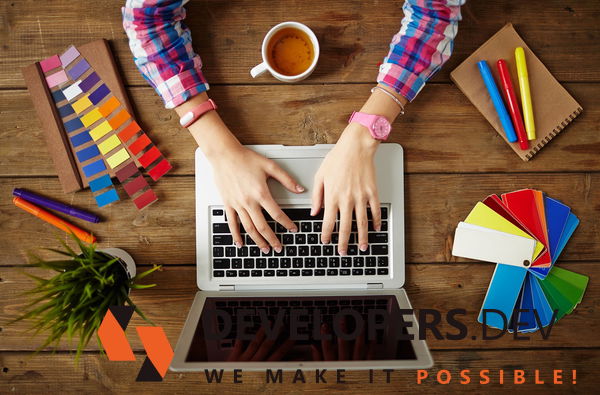
Many significant data sources exist, including transaction processing systems, customer databases and documents.
Also, email, documents, emails, medical records, internet clickstream logs, mobile applications, social networks, and medical records. Machine-generated data includes network logs, server logs, and data from sensors on industrial equipment, manufacturing machines, and internet of things devices.
Big data environments include data from the internal system and external data about consumers, financial markets and weather conditions.
Geographic information, scientific research, and other data can be added to these systems. Big data also includes images, videos, and audio files. Many big data applications use streaming data to process complex processes and collect data on a regular basis.
The Vs of Big Data: How to Break it Down
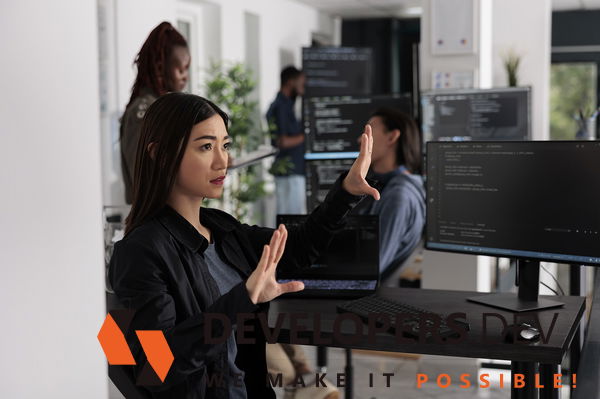
The most common application characteristic of big data is volume. Although a large data environment does not have to hold a lot of data, it should contain enough data due to the nature of the data that is being stored and collected.
Many sources produce large volumes of data, including clickstreams, system logs, and stream processing systems.
A wide range of data types are also included in big data, such as the following:
- Structured data, such as transactions and financial records
- Unstructured data such as text and documents;
- Semistructured data includes web server logs, streaming data from sensors and other data.
Extensive data systems need to store and manage many data types. Big data applications may include multiple data sets, which cannot be integrated upfront.
A real time -time analytics processes project might attempt to predict sales by combining data from past sales, returns and customer service calls.
Velocity is the speed with which data must be generated, processed and analyzed. Many cases see big data being updated in real-time or very close to it, rather than the weekly, monthly, or daily updates made in traditional data warehouses.
As extensive data analysis expands into machine learning ( AI), which uses data to find patterns and generate insights, managing data velocity is essential.
Big Data has More Characteristics
Well be looking beyond the three original Vs to see details about other terms often associated with big data.
- Veracity is the level of accuracy and trustworthiness in data sets. Raw data from different sources can cause data quality issues. This may make it challenging to identify. Insufficient data can lead to errors in analysis that could undermine the effectiveness of business analytics efforts. streaming Analytics technologies teams and data management teams must also ensure they have sufficiently accurate data to produce valid results.
- Some data scientists and consultants also add value to the big datas characteristics. Some data collected may have little business benefits or value. Before big data analytics projects are initiated, organizations must verify that the data they collect has business value.
- Variability can also be applied to big data sets, which have multiple meanings and be formatted differently in different data sources. These factors further complicate big-data management as well as analytics.
Some people attribute even more of Vs big data to them; there are a variety of lists with seven-ten.
What is the Best Way to Store and Process Big Data?
A data lake is a common place to store big data. Data warehouses are often built using relational databases and only contain structured data.
However, data lakes can keep a variety of data types. They are typically based on Hadoop Clusters, cloud object storage systems and other big data analytics platforms, such as NoSQL tables or other big data streaming platforms.
Big data environments often combine many systems in a distributed architecture. For example, a central database might be integrated with relational databases and a data warehouse.
Big data systems can store data in raw form. Still, it may be filtered and organized according to specific analytics needs.
It takes work to get that much processing power cost-effectively. The cloud is becoming a trendy place for extensive data systems.
Organizations can deploy their cloud-based systems or use managed big-data-as-a-service offerings from cloud providers. Cloud users can increase the servers needed to complete large data analytics projects. The company only has to pay for the computing model and storage time used.
Cloud instances can also be shut down until needed.
Read More: Big Data: What are they?
How Big Data Analytics Works
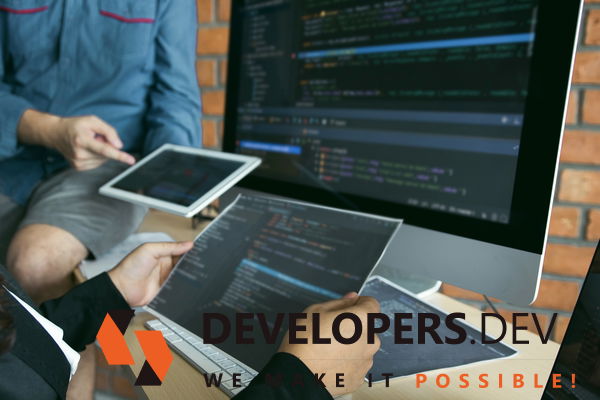
It is impossible to answer this question in a way that reflects all reality. Depending on what you consider simple, it can be simpler or more complicated.
A data solution can include specific factors that could lead to significant technological or financial investments.
Technology is plural.
The data is the first part of the infrastructure. Systems must collect and store data correctly. This is done using infrastructure (hardware, software, and hardware).
Storage servers are servers that specialize in ample data storage. They can take up significant space in data centers, depending on the solutions size.
Data scientists and other analysts need to have a deep understanding of the data available and an idea of what they are looking for.
Data preparation is a critical first step in any analytics process. It includes data cleansing, validation, transformation, and profiling.
After the data is gathered, it can analyze a wide variety of applications using advanced analytical tools. These include predictive analytical models, machine learning, and its deep learning offshoot.
As an example, lets take customer data and show you the various branches of analytics that can use big data:
- Comparative analysis: This analyzes customer behavior, customer experience and real-time engagement to determine if a companys products and services are comparable with its competitors.
- Social media listening: This analysis analyzes the social media conversations about a product or business to help target customers and identify potential issues.
- Marketing analytics: provides information and analytics features that can help improve marketing campaigns and promote products, services, and business initiatives.
- Sentiment analysis: Customers can use their data to analyze how they feel about a company, brand, and potential problems.
Big Data Management Technologies
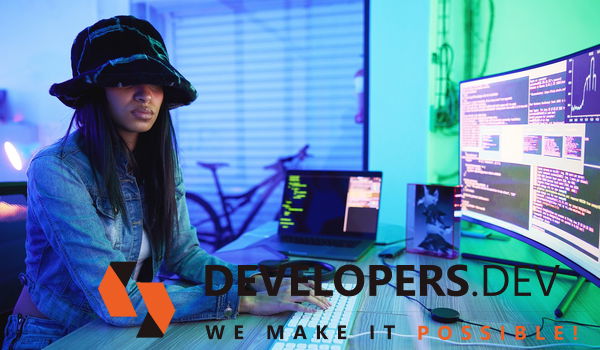
Hadoop, an open-source distributed processing framework, was initially at the heart of many big data architectures.
Spark and other processing engines led to MapReduce being built into Hadoop. This ecosystem of big data technologies can be used in different applications but are often deployed together.
IT vendors offer managed services and big data platforms that combine several technologies into a single package.
This is primarily for cloud use. These offerings are listed alphabetically at the moment.
- Amazon EMR (formerly ElasticMapReduce)
- Cloudera Data Platform
- Google Cloud Dataproc
- HPE Ezmeral Data Fabric (formerly MapR Data Platform).
- Microsoft Azure HDInsight
The following technologies are available for organizations that want to implement extensive data systems on their own, either in the cloud or on-premises.
- Storage repositories such as Hadoop Distributed File System ( HFS) or cloud objects storage services like Amazon Simple Storage Service ( SS), Google Cloud Storage, and Azure Blob Storage.
- Cluster management frameworks like Kubernetes or Mesos and YARN are Hadoops built-in resource manager and job scheduler. This acronym stands for Yet Another Resource Negator, but the singular often refers to it;
- Stream processing engines such as Flink and Hudi, Kafka and Samza, Storm, and the Spark Streaming or Structured Streaming modules integrated into Spark
- NoSQL databases include Cassandra and Couchbase, CouchDB and HBase. MarkLogic Data Hub, MongoDB. Neo4j. Redis, MarkLogic Data Hub and other technologies.
- Data lake and data warehouse platforms include Amazon Redshift, Delta Lake and Google BigQuery, Kylin, Snowflake, and Google BigQuery.
- SQL query engines like Drill, Hive and Impala.
Big Data Challenges
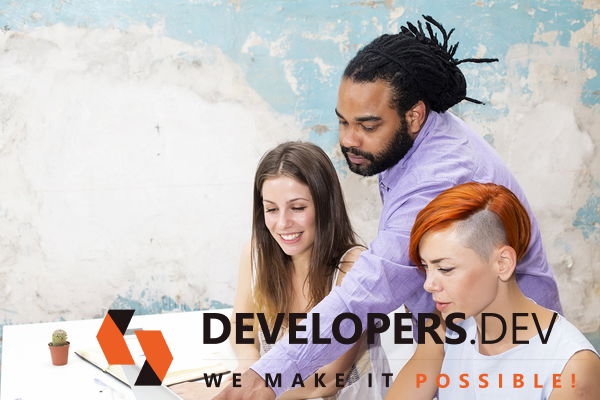
Designing a big-data architecture is often challenging concerning processing capacity issues. The design of extensive data systems should be customized to the needs of each organization.
This DIY project requires IT and data managers to create a custom set of technologies and tools. Management and deployment of extensive data systems require new skills than those required by database administrators or developers who work with relational visualization software.
These issues can be solved by using managed cloud services. However, IT managers must monitor cloud usage to ensure that costs are manageable.
It can be difficult to migrate data sets from on-premises and processing workloads into the cloud.
Another challenge in big data technology is making data available to data scientists and analysts in distributed environments with various data stores and platforms.
Data management and basic analytics teams are becoming more adept at building data catalogs that include metadata management and data lineage function to aid analysts in finding relevant data. Integrating big data sets is often complicated, especially when there are many variables like data velocity and data variety.
How to Create a Big Data Strategy That Works
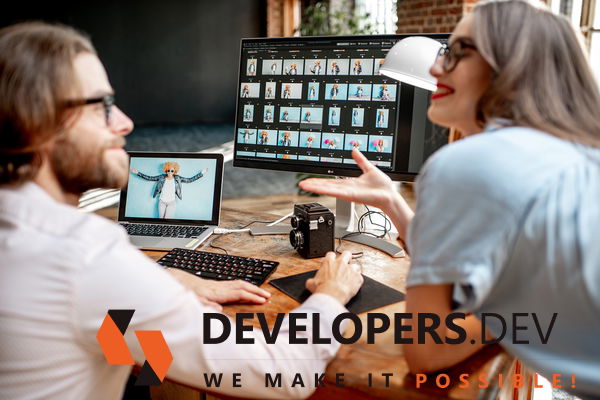
Developing a big-data strategy in an organization requires an understanding and assessment of business goals, data available, and data needed to meet those objectives.
Next, you need to consider the following:
- Prioritizing applications and use cases planned is critical.
- Identifying new systems and tools that are required;
- A deployment roadmap is created.
- They are assessing internal skills to determine if retraining and hiring are necessary.
A data governance program and the associated quality business processes are essential to ensure that big data sets are consistent, clean, and properly used.
Another best practice for managing and advanced analytics big data is to focus on business requirements and use data visualization to assist in data discovery and analysis.
Regulations and Practices for Extensive Data Collection
Data misuse has become more common as data collection and data use have increased. The European Union approved the General Data Protection Regulation ( ) in May 2018 after a public outcry over data breaches and other violations of personal privacy.
GDPR restricts the data organizations can collect. It also requires individuals to consent or comply with specific reasons for collecting personal information. It also includes a right-to-be-forgotten provision, which lets EU residents ask companies to delete their data.
Although there arent any similar federal laws in the U.S.A, the California Consumer Privacy Act ( CCPA) is designed to give California residents greater control over collecting and using their personal data by companies doing business in California.
The CCPA became effective on January 1, 2020, after it was passed into law in 2018.
Businesses must adhere to such laws by carefully managing extensive data collection. Companies must have controls to identify the regulated data and prevent unauthorized employees from accessing it.
Extensive Data Management and Analytics: The Human Side
The workers responsible for analyzing and managing the data ultimately determine the business value and additional benefits of significant data initiatives.
Big data tools allow less-technical users to run predictive analytics tools in apps or help businesses set up the infrastructure needed for big data projects.
One way to compare big data with small data is to say that they are data sets that can easily be used for self-service BI and analytics.
One common hypothesis is, "Big data is only for machines; small data is only for people."
Want More Information About Our Services? Talk to Our Consultants!
Conclusion
Big data technologies have many benefits for companies, including seamless integration, reduced learning curves, real-time analysis, and intelligent decision-making.
Which can play a huge role in increasing your business revenue to a large extent. Developers.dev is here to give the most dependable and most sophisticated technological solution in big data analytics contact us at any good time to get the most trusted name in the industry with state of the art ultra modern solutions.