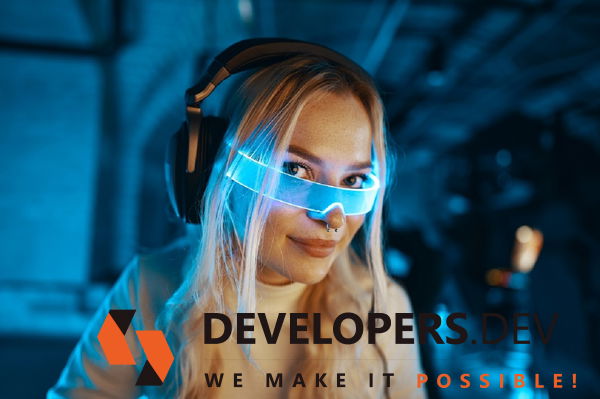
What is Big Data?
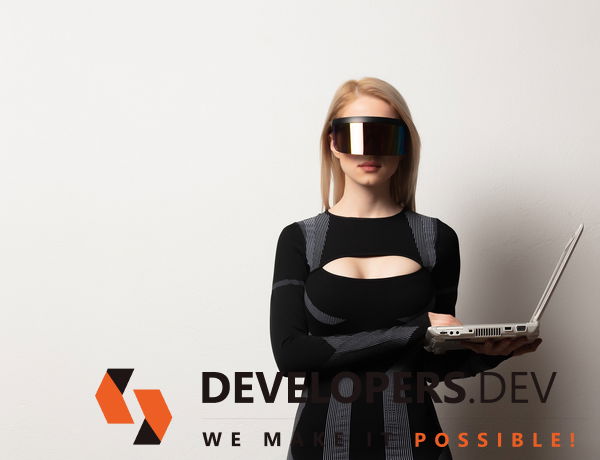
Before I explain what Big Data is, let me briefly discuss its opposite! One common misunderstanding is that Big Data refers to large volumes or sizes of collected information.
Still, Big Data refers to an abundance of diverse and unique datasets from various sources that come flooding in from all directions, creating multiple formats of this massive amount of information that formerly was stored using personalized recommendations of traditional relational database systems that cannot keep pace. Big Data represents much more than an accumulation of datasets; rather, it represents an invaluable asset that can bring many tangible advantages in return.
Big data refers to information assets (data) with high volumes, velocity, and variety that are extracted, analyzed, and processed for decision-making or control actions.
Big Data poses unique challenges that make its analysis nearly impossible through traditional data analytics techniques. In simple terms, big data refers to information that contains greater variety, arrives more rapidly, and has more incredible velocity; also known as the three Vs of big data.
Big data refers to larger and more complex data sets from new sources that traditional data processing software cannot handle effectively.
However, this finance domains immense volume of information may allow businesses to address challenges they previously couldnt address effectively. Big datas value lies in uncovering patterns and insights hidden in significant information assets that can influence business decisions.
When extracted using advanced analytics technologies, these insights enable organizations to comprehend better how their users, markets, societies, or the entire global environment behaves.
Read More: All You Need To Know About Big Data
Characteristics of Big Data
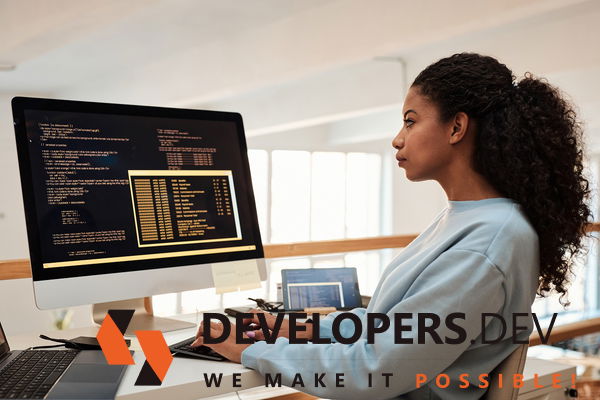
Here are the traits associated with big data
As Big Data continues to change and develop, so will its constituent parts - I will list five additional Vs that have come about slowly over time in this image:
- Validity refers to correct data, while Variability refers to the dynamic behavior
- Volatility refers to temporally changing events.
- Vulnerability refers to being vulnerable to breaches or attacks
- Visualization represents meaningful usages of data.
Volume
Volume of Data High-volume data often holds valuable insights. A minimum threshold for Big Data generally begins between terabytes and petabytes in size; to fully explore Big Data, it requires hyperscale computing environments with ample storage and fast IOPS (Input/Output Operations per Second) for fast analytics processing.
Big data means processing high volumes of low-density, unstructured information. This may come in the form of Twitter data feeds, clickstreams from websites or apps, or sensor-enabled equipment that produces unknowable values like Twitter feeds or clickstreams from mobile apps.
Depending on an organizations size and goals, this amount could reach several petabytes.
Velocity
Velocity measures the speed at which data is produced and processed. Big Data typically comes out in streams that become readily available in real time use cases for decision-making purposes.
The rate refers to how quickly received and potentially processed information arrives - typically directly into memory rather than disk; some internet-enabled innovative products operate in real-time or near real-time, which requires real-time evaluation and action taken accordingly.
Variety
Considerable data assets come in all forms and varieties. Raw big data is often unstructured or multi-structured and produced using different attributes and standards that must all fit together perfectly into file formats compatible with analysis and decision-making - such as datasets collected via sensors, log files, or social media networks, which must then be processed into structured databases for data analytics and decision-making purposes.
Variety refers to the diverse forms of data available today. Traditional structured predictive analytics databases suited standard data types well.
But with big datas rise came more unstructured types such as texts, audio files and videos, which require further preprocessing to find meaning or support metadata.
Veracity
Data reliability or truthfulness. How relevant the output from extensive data analysis is to associated business goals is determined by factors including data quality, processing technology used and mechanisms utilized for analyzing information assets.
Value
Value in big data analyses provide businesses with valuable assets; their results should be judged against specific objectives for business evaluation.
Big Data Vs Small Data Vs Thick Data
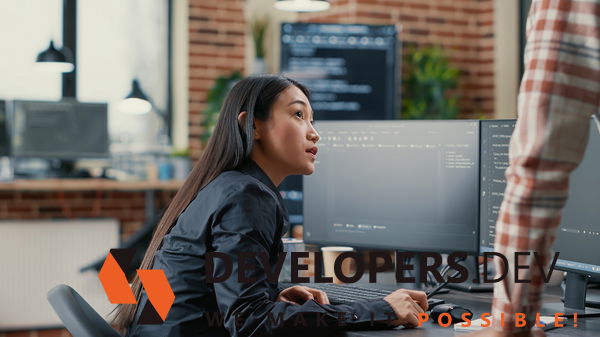
Contrasting these characteristics are two other forms of data: small and thick.
Small Data
"Small Data" refers to manageable assets of numerical or structured form that can be easily analyzed using simple technologies, like Microsoft Excel or an open-source alternative.
Thick Data
"Thick Data" refers to any textual or qualitative data that can be quickly processed manually with limited errors, for instance:
Video transcripts
Qualitative analytics help uncover sentiment and behavioral aspects easily conveyed through individual conversations by integrating qualitative processing with spark quantitative big data.
Thick Data can prove particularly effective in medicine and scientific research, where human responses hold more value and insight versus large, significant data streams.
Significant Data Trends
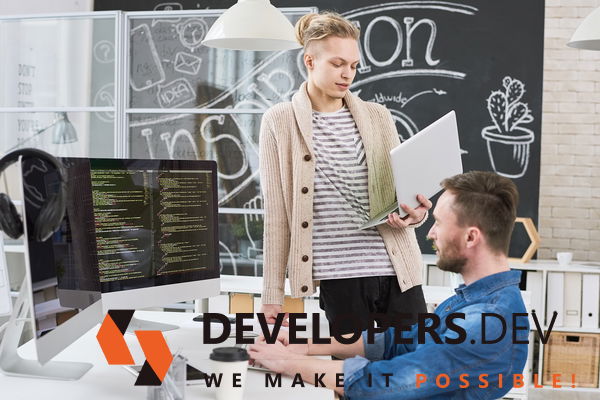
Big Data technologies continue to advance as data quickly becomes the wide variety cornerstone of every successful organization.
Internet of Things (IoT), Cloud Computing and Artificial Intelligence (AI) technologies have made it simpler than job descriptions ever for organizations to transform raw data into actionable knowledge. Here are three of the critical significant data technology trends to keep an eye on in 2021:
Augmented Analytics: By 2021, the Big Data industry will reach approximately $274 billion. Technologies that assist organizations in data management processes, like Augmented Analytics, are projected to experience rapid expansion, reaching $18.4 billion by 2023.
Continuous Intelligence: Integrating real-time analytics into business operations allows organizations to leapfrog competitors with timely, actionable insights delivered instantly.
Stringent data security: Laws such as GDPR and HIPAA encourage organizations to secure, accessible, and reliable their information systems.
Blockchain has quickly gained prominence within financial services as a data governance and security instrument capable of countering privacy risks effectively; this EU resource discusses how blockchain fulfills some key GDPR objectives.
Big Data Exists everywhere, and peoples curiosity about it has increased over the wide variety of past years. Forbes reports that users watch 4.15 million YouTube videos every minute, tweet 456,000 tweets, post 46,740 photos to Instagram, create 510,000 comments, and update 293,000 statuses on Facebook! All that in one minute alone!
Just imagine all of the data generated through such activities! From social media, business applications and telecom to various domains - this constant creation of data leads to Big Data being created constantly - giving rise to its formation.
You can better understand this subject matter with the Hadoop Course.
As part of my explanation of what Big Data entails. Big Data Can be Defined as (and Defined by) Critical Elements for Understanding Big Data at This Stage and in Industrial Applications, but It Can Still Have Many Applications at Once as per Industry.
Finally, it Has Many Scope Applications as Per Industry Needs.
Read More: How important will be Big Data in the upcoming decade
Evolution of Big Data
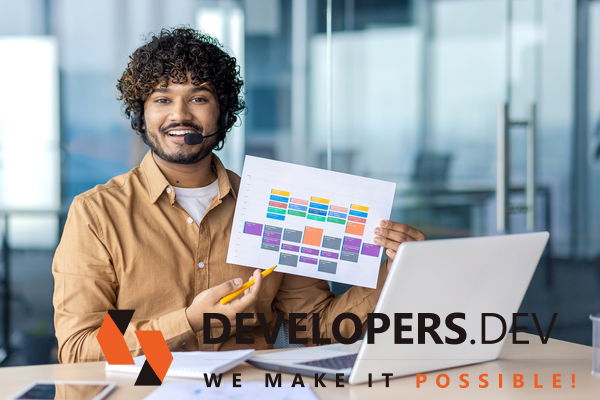
Before exploring further, let me provide some background on why this technology has gained so much significance.
Remember the last time you used a CD or floppy batch processing to store data? My guess would be back in the early 21st century. Due to exponential data growth, manual paper records, files, and Slack and disc storage options have become outdated and insufficient due to demand from inventions, technologies, and applications with fast response times and internet applications.
However, relational database systems were initially adequate. Now, with continuous and massive generations generating steady data flows known as Big Data, which I will elaborate further in this blog.
Forbes estimates that each day, we generate 2.5 quintillion bytes of data - an amount only set to increase with technology such as wide range IoTs involvement accelerating that figure further; 90% of todays data was created within two years!
Big Data Analytics
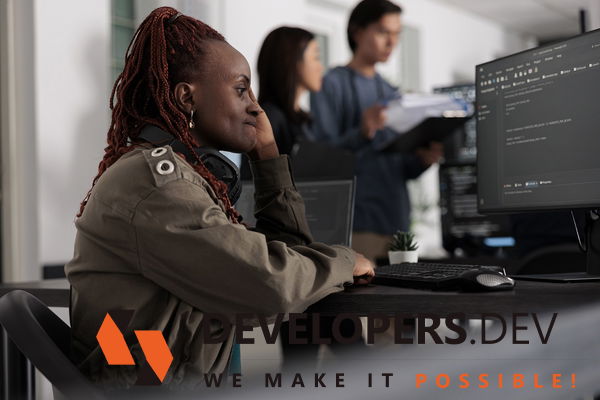
Now that Ive discussed what Big Data is and its rapid proliferation let me give an interesting example of how Starbucks, one of the leading coffeehouse chains, uses Big Data in their business practices.
Forbes featured this article, which detailed how Starbucks utilized this technology to gain more insights into customer preferences to enhance and personalize the customer experience. They analyzed members coffee purchasing behaviors, from when and what type they order to when it usually happens.
Therefore, when customers visit "new" Starbucks locations, their point-of-sale system can identify customers through smartphones and provide baristas with their preferred orders.
Based on customer ordering patterns and product preferences, their app will deep learning recommend new items that may pique customers interests - this practice is known as Big Data Analytics; for more details, take the Data Engineering Course!
Big Data Applications
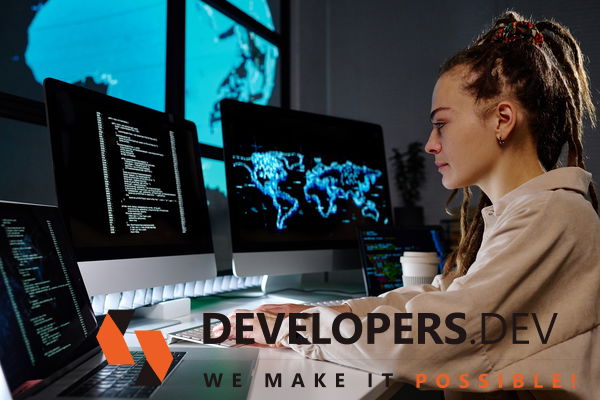
Below are just a few fields where Big Data Applications have proven revolutionary:
Entertainment: Netflix and Amazon leverage it to recommend shows and movies to their users. Insurance uses it to predict illness or accidents to price products accordingly.
Driverless Cars: Googles driverless cars collect an average of approximately one gigabit per second for experimentation purposes, necessitating more data as each experiment progresses successfully.
Education: Utilizing big data-powered technology as a learning tool instead of traditional lecture methods has significantly enhanced students learning experiences and helped teachers track performance more closely.
Automobile: Rolls Royce has taken great strides toward adopting this technology by installing thousands of sensors into its engines and propulsion systems to monitor every element of their operation in real-time, then reporting back the information directly to engineers who determine the most suitable course of action, machine learning and big data whether scheduling maintenance visits or dispatching engineering teams should additional help be required for solving specific problems.
Government: Another exciting use case lies within politics to study patterns and influence election results.
Cambridge Analytica Ltd is one organization that utilizes data analysis techniques to change audience behaviors - they play an essential part in electoral politics!
Scope of Big Data
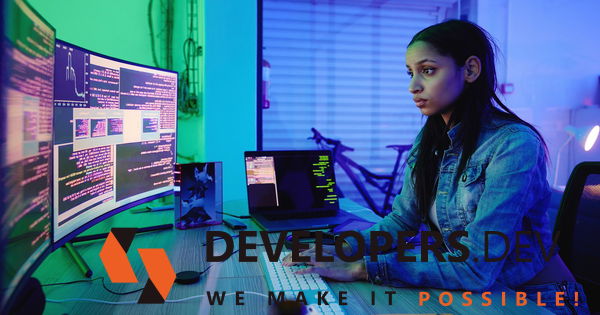
Numerous Job Prospects: Big data offers multiple career options such as Analyst, Engineer and Solution Architect roles.
According to IBM data science analytics job demand is highest within Finance & Insurance, Professional Services and IT industries.
Demand for Analytics Professionals Is on the Rise: Forbes reported that IBM predicts data scientist demand will surge 28% by 2020 and create 364,000 more job openings to reach 2,720,000, per their projections.
Salary Aspects: According to Forbes, employers are willing to offer an additional $8,736 above median bachelor and graduate-level salaries; successful candidates typically start their wages at around $80,265
The Value Of Big Data
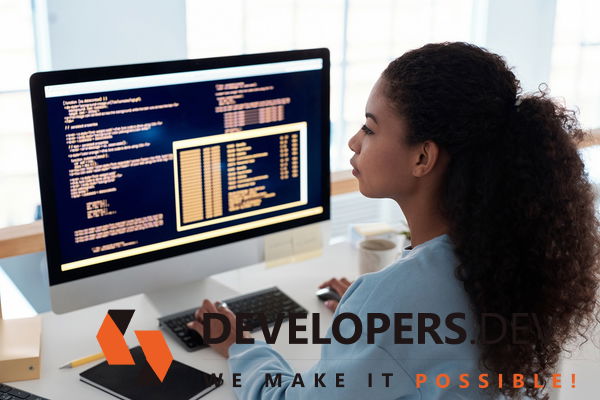
Over the past years, two additional Vs have taken shape: value and integrity meaningful insights. Although data possesses intrinsic worth, its full utility depends on aviation, tourism, finance being unlocked through proper analysis.
Furthermore, how truthful or reliable is your data? Nowadays, big data has become capital. Look at some of the biggest tech companies; their value largely stems from analyzing their vast amounts of information to increase efficiency and develop innovative new products.
Recent technological breakthroughs have significantly reduced data storage and computing costs, making it simpler and cheaper than ever to store more information than ever.
Now, with access to big data being more affordable and more accessible, the finance domain sparks streaming digital transformation than ever before, making decisions more accurately and precisely than before can become more straightforward and cheaper. Finding value in big data doesnt just involve analyzing it (although it can benefit you). It requires insightful analysts, business users, and executives who ask the appropriate questions, recognize patterns, make informed assumptions, and predict behavior.
The History Of Big Data
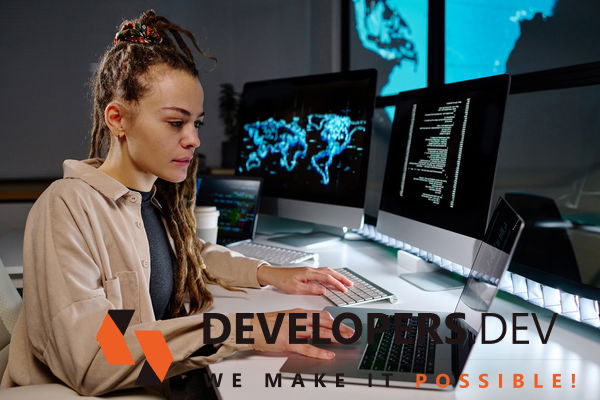
Though big data as we understand it today is relatively recent, its roots can be traced back to the 1960s and 1970s when data centers and relational databases first started emerging as entities in our world of data.
Around 2005, people realized how much data users generated via platforms like Facebook and YouTube. Hadoop (an open-source framework designed specifically for analyzing big data to store and analyze large sets of data) was developed that year, while NoSQL also began growing increasingly popular during this time.
Hadoop (and more recently Spark) was instrumental in driving big datas rise, making it simpler and cheaper to manage than its predecessor real time use cases; big data production continues to skyrocket as more users generate massive quantities of information--with AI also playing its part.
Since the advent of the Internet of Things (IoT), more objects and devices have been connected to the web and collecting customer usage patterns and product performance data via machine learning technologies.
Machine learning itself has also produced even more data that needs analyzing.
Big data may have come a long way since its debut, yet its true potential remains to be fully exploited. Cloud computing has expanded big datas application even further to absolute data - offering elastic scalability with no cluster spin up time required when testing subsets of data sets, and graph databases continue to play an ever-increasing role as they display massive quantities of information efficiently for analysis.
Big data offers us more comprehensive answers due to having access to more information. More confidence in the data means taking an entirely different approach towards solving problems.
Big Data Use Cases
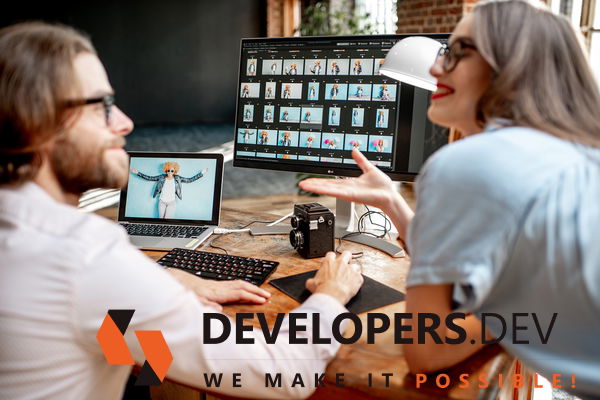
Big data offers businesses many potential benefits in business operations, ranging from customer experience and analytics to managing a growing enterprise and workforce development.
Below are just a few.
Product Development Companies like Netflix and Procter & Gamble use big data analytics solutions to anticipate customer demands.
Predictive models for new products and services are developed by categorizing critical attributes of existing natural language processing, analyzing big data and past offerings and modeling the relationships between those features and commercial success. P&G uses data and analytics from focus groups, social media channels, test markets, aviation, tourism, finance and early store rollouts to plan, produce and launch new products.
Predictive maintenance factors that predict mechanical failure may lie deep within structured data, such as model year/make/model information, as well as unstructured sources, such as log entries/sensor data/error messages and engine temperature records.
By anticipating potential issues before they manifest into actual matters, organizations can reduce maintenance expenses while improving parts and equipment uptime.
Customer ExperienceThe: The competition to win customers hearts and minds has just begun. An accurate view of customer experience has never been simpler with massive data sets, big datas capabilities to compile customer insights from social media, website visits, call logs and other sources to optimize interactions while increasing the value delivered.
Start making personalized offers, reduce customer churn, and address issues proactively with these fraud and compliance solutions.
Fraud and complianceWhen it comes to security, your competitors dont just comprise an isolated hacker; instead, entire expert teams exist whose goal is to gain entry through vulnerabilities in systems.
Their security landscape and compliance needs keep evolving - you need the right strategy now to remain compliant and survive the battles ahead. Big data helps identify patterns in data that indicate fraud and aggregate large volumes of information for quicker regulatory reporting.
Machine learning Machine learning has recently become one of the hottest trends, and big data plays an instrumental role.
Now, we can teach machines instead of programming them thanks to big datas availability to train machine learning models, and operational efficiency has never been more significant due to this development.
Although its impact might not but wide variety interactive analytic always make headlines, big datas most important contribution may lie elsewhere: in operational efficiency. Big data allows companies to analyze production, customer feedback, returns, and other factors to reduce outages and anticipate future demands.
Big data can also help businesses enhance decision-making based on current market demand and drive innovation by studying interdependencies among humans, institutions, entities and processes to uncover fraud detection and new insights that may aid creation. Leverage this knowledge for financial planning considerations as well as customer trends analysis as you deliver new products and services while using dynamic pricing structures - theres endless possibility.
Big Data Challenges
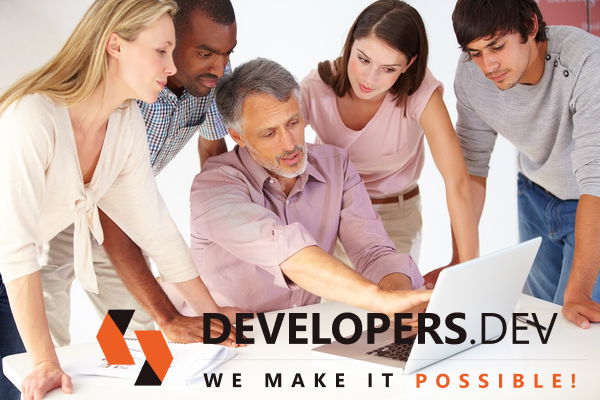
Big data holds great promise but does present its share of hurdles. First and foremost, big data is indeed "big." While new technologies exist to store it safely and effectively, business and web analytics data volumes continue to double every two years, and organizations need help finding ways to keep their information wide variety.
Curation is critical in making data valuable; data scientists often spend up to 80% of their time curating and prepping information before it can be used.
Big data technology is changing quickly. A few years ago, Apache Hadoop was widely used with in-depth knowledge for processing big data; in 2014, Apache Spark was an alternative framework.
Today, the best practice is combining these frameworks. Staying abreast of big data accenture analytics technology remains an ongoing challenge with structured data.
How Big Data Works
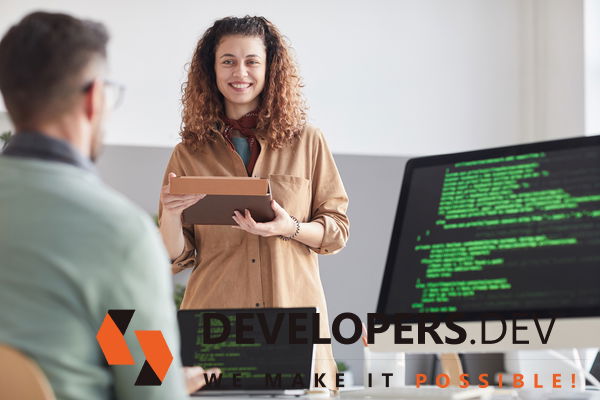
Big data offers insights that open new business models. Getting started involves three key actions.
Integrate
Big data brings together information from numerous disparate sources and applications. Traditional methods, like extract, transform and load (ETL), may need to be revised in processing this enormous volume of information - new strategies and technologies must be devised to analyze such large-scale sets containing multiple petabytes or more effectively! Integration requires gathering relevant data, processing it appropriately, and ensuring that business analysts can use it immediately.
Manage
Big data requires storage. Your solution could include either cloud, on-premises, or both options; your choice depends on where your data resides.
Most often, natural language processing people choose their solution based on where their current computing requirements exist: article big data analytics with structured data industry while having access to resources as necessary - more and more people are opting for cloud solutions, which offer increased flexibility concerning scaling resources as needed and access.
Analyze
Your investment in big data pays dividends when you analyze and act upon it. Gain perspective with visual analyses of different sets, explore deeper to make discoveries, share findings with others and create models with machine learning and artificial intelligence - put your data to work!
Want More Information About Our Services? Talk to Our Consultants!
Big Data Best Practices
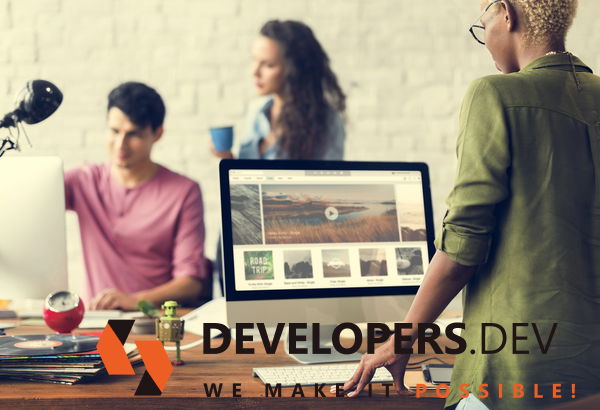
As part of your big data journey, here are a few essential best practices we suggest integrating. Here is our framework for developing an adequate, significant data foundation analytical big data.To determine whether big data supports and facilitates your crucial business and IT priorities, ask how it enables these goals.
Examples include:
- Learning how to filter web logs to understand ecommerce behavior.
- Deriving sentiment from social media posts and customer support interactions.
- Understanding statistical correlation methods relevant to customer, product, manufacturing and engineering data.
One significant barrier to realizing value from your investment in big data is its need for more skilled personnel for analysis and visualization.
Adopting standards, massive data sets and a variety of application governance measures can alleviate skills shortage issues of machine learning and big data that impede progress with significant data investments. As part of your IT governance program, ensure big data technologies, considerations and decisions are included within its guidelines to reduce this risk.
Standardizing your approach will enable you to control costs better while optimizing resources. Organizations looking to implement big data solutions or strategies must assess their skill requirements early and often to identify any possible gaps or skills deficiencies that could impact implementation success machine learning and big data.
These challenges can be met through training/cross-training existing resources, hiring new ones and using consulting firms as needed. To optimize knowledge transfer with a center of excellence.
Utilize this approach for sharing knowledge, controlling oversight and overseeing streaming platform project communications.
Whether it is new or expanding sophisticated Parallel-Data Processing Pipelines investment in big data, its soft and hard costs should be spread among the enterprise to reduce overall expenses and costs more systematically and distributing streaming methodically-one key payoff from using this approach to align unstructured and structured data. Analyzing big data alone is valuable, but for even greater business insight, you could connect and combine low-density significant data sources with existing structured information you are currently using.
No matter whether its customer, product, equipment or environmental data being captured via extensive data capture methods, your goal should always be to add meaningful insights more relevant points that lead to improved conclusions - for instance, distinguishing all customer sentiment from that of only your best analytics industry
Plotly can also be used to style Interactive Graphs with Jupyter notebook.TensorFlow has a Comprehensive, Flexible Ecosystem of tools, Libraries and Community resources that lets Researchers push the state-of-the-art in Machine Learning and Developers can easily build and deploy Machine Learning powered applications.
Beam provides a Portable API layer for building sophisticated Parallel-Data Processing Pipelines that may be executed across a diversity of Execution Engines or Runners.Docker is a tool designed to make it easier to Create, Deploy, and Run applications by using Containers.
Plotly can also be used to style Interactive Graphs with Jupyter notebook.TensorFlow has a Comprehensive, Flexible Ecosystem of tools, Libraries and Community resources that lets Researchers push the state-of-the-art in Machine Learning and Developers can easily build and deploy Machine Learning powered applications.BeamApache Beam provides a Portable API layer for building sophisticated Parallel-Data Processing Pipelines that may be executed across a diversity of Execution Engines or Runners.Docker is a tool designed to make it easier to Create, Deploy, and Run applications by using Containers.
Plotly can also be used to style Interactive Graphs with Jupyter notebook.TensorFlow has a Comprehensive, Flexible Ecosystem of tools, Libraries and Community resources that lets Researchers push the state-of-the-art in Machine Learning and Developers can easily build and deploy Machine Learning powered applications.BeamApache Beam provides a Portable API layer for building sophisticated Parallel-Data Processing Pipelines that may be executed across a diversity of Execution Engines or Runners.Docker is a tool designed to make it easier to Create, Deploy, and Run applications by using Containers.
customers can help make better conclusions; many consider big data an integral component of their existing business intelligence capabilities, data warehouse platform or information architecture platform.
Remember that big data analytical models and processes may involve humans and machines google research with in depth knowledge.
Big data analytical capabilities include statistics, spatial analysis, semantics, interactive discovery and visualization. With analytical models in hand, you can connect different sources and types of information to make google AI associations or meaningful discoveries digital transformation.
Discovering meaning in data may take some work. Sometimes, we are still determining exactly what we are searching for - that is expected. Therefore, Management and IT must support this "lack of direction" or lack of precise requirements as necessary.
At the same time, analysts and data scientists must work closely with businesses to understand critical knowledge gaps and business operations requirements.
To facilitate interactive exploration of data and experimentation of statistical algorithms, high-performance work areas are needed; ensure your sandbox environments have all necessary support--and are appropriately governed.
Want More Information About Our Services? Talk to Our Consultants!
Conclusion
Aligned with the cloud operating model, big data companies processes and users require access to an extensive set of resources for both iterative experimentation and running production jobs, with solutions comprising all realms, including transactions, master data, reference data and summarized data forming part of their solution.
Analytical Sandboxes should also be created on demand. Resource management is crucial in overseeing data flow, including pre-and postprocessing operations and integration, in-database summarizations and analytical modeling activities.
Therefore, planning out provisioning and security strategies is integral in meeting these evolving requirements.