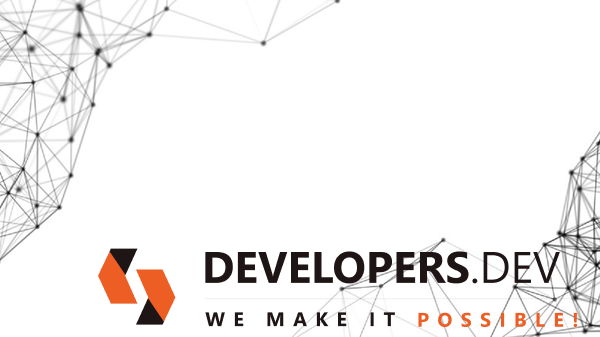
Data Analytics platforms serve as central archiving and processing facilities in any organization, collecting, purifying, transforming and using all information to gain business insight.
Companies which prioritize data have adopted data platforms to aggregate, operationalize and disseminate it across their organization.
Data teams must consider this tool when developing, managing and surfacing products derived from it.
We outlined six layers that you should include in your platform, according to how most successful teams implement them.
We have updated this post with additional layers commonly seen today like access management, data governance and machine learning that often make an appearance on modern data platforms.
The Six Layers That A Modern Data Infrastructure Must Have
FinTech startups with 200 people may require different sets of data than an e-commerce firm with 5,000 staff, nonetheless, both platforms will share common layers.
Remember this: Just as building a house requires foundation, frame and roofing components in the end, data platforms cannot function effectively without these six layers.
We will describe below what an "essential" platform looks like as well as some tools you might consider using.
Data Storage and Processing
Bottom layer for processing and storing data. A place must exist where you can safely store and process your data before sending it off for analysis; data storage/processing layers can especially prove helpful when working with large datasets over extended time frames.
Cloud-native solutions have taken over the market like never before, offering more cost-effective and accessible data storage than on-premise alternatives.
Solutions such as data lakes or warehouses (lakehouse) make storing information much simpler for organizations of any size than on-premise alternatives.
Decide for yourself whether a data lake, store or combination thereof best meets your needs.
Recent discussions regarding building data platforms with open or closed source solutions has generated much discussion.
No matter where your loyalties lie, modern data platforms cannot be constructed without making investments in cloud computing and storage solutions.
Here are some of the most popular options for cloud landscapes, lakes, and warehouses.
- Snowflake is the original cloud data warehouse- Snowflake offers a flexible pricing structure to data teams. Users are charged different rates for calculating and storing data.
- Google BigQuery- Googles cloud-based warehouse, BigQuery provides a serverless architectural design that allows quick queries due to parallel processing. It also offers separate storage for comparison and scalable memory and processing.
- Amazon Redshift (AWS) - One of the most common solutions is Amazon Redshift. It is hosted on Amazon Web Services and has simple tool integration.
- Firebolt is a SQL-based cloud-based data warehouse which claims to be up to 182x faster than the other options. This is because it handles data more efficiently thanks to new techniques of compression and data parsing.
- Microsoft Azure is Microsofts cloud computing offering, which appears on this list of teams who heavily integrate Windows.
- Amazon S3- An object storage service that stores structured and unstructured information, S3 provides the computing resources you need to create a data lake.
- Databricks- Databricks is the Apache Spark as a Service platform. It pioneered the Data Lakehouse. Users can leverage both structured data and unstructured, and it offers low-cost data storage.
- Dremio- Dremio's data lake engine offers analysts, data scientists and data engineers an integrated self-service interface to data lakes.
Data Ingestion
Todays world demands data transfer across platforms and systems, but many organizations find themselves struggling to do this effectively.
As data infrastructures become more complex, data teams face an ever-increasing task: they must ingest structured and unstructured information from multiple sources - this process is known as Extract Transform Load or ETL for short.
Zero-ETL refers to data integration from one place to another without using data pipelines; however, widespread adoption remains far off.
Data engineers often gather their information by employing batch techniques; that is, gathering it at set intervals or in predefined groups of times or dates.
More recently however, real-time streaming technologies are becoming more prominent, especially for operational uses when their near real-time nature justifies any complexity that ensues.
The following solutions are available for batch data ingestion:
- Fivetran is a leading enterprise ETL software that manages the delivery of data from the source to the destination.
- Singer - A free tool that allows you to move data from one source to another.
- Stitch is a cloud-based, open-source platform that allows users to move data quickly from one source to another.
- Airbyte is an open-source platform that allows for easy data synchronization between applications.
Data streaming ingestion includes:
- Confluent supports Apache Kafka (the open-source event streaming platform) to handle streaming analytics and data intake. A different streaming solution, Apache Flink, was recently acquired by them.
- Amazon Kinesis - A streaming service from AWS, which may be perfect for users of Redshift as a data warehouse.
- Google Pub/Sub - A service of GCP that allows you to stream data directly into BigQuery or other operational databases, such as data lakes. When Google announced that Pub/Sub data could be streamed directly into BigQuery without pipelines, it caused a stir last year.
- Apache Spark - Often used in conjunction with Databricks, this open-source solution is marketed as an analytics engine that can handle large amounts of data.
Some data teams still prefer writing custom code in order to ingest data, both from internal and external sources, into their systems; many organizations even create their own frameworks specifically for this task.
Ingestion layers frequently include orchestration and workflow automation using tools like Apache Airflow Prefect and Dagster, taking one step forward by orchestration to integrate information siloed from disparate sources into analysis-ready datasets for easy consumption.
Data Transformation And Modeling
Data transformation and modeling can often be confused; theyre actually two separate processes that should not be treated similarly.
Transforming data to prepare it for analysis or reporting can be achieved through data modeling. Visual representation allows data warehouse users to store more effectively.
Below we have provided a list of tools most frequently utilized by data engineers for data transformation and modeling:
- Data build tool (dbt) is an open-source leader in transforming data after it has been loaded into your warehouse.
- Dataform - Now part of Google Cloud, Dataform lets you transform your raw data into something that can be used by BI and analytical tools.
- Sequel Server Integration Services - Hosted and maintained by Microsoft, SSIS allows you to extract data from various sources. You can then use this data to load it into the destination of your choice.
- Apache Airflow and Custom Python Code - Before tools such as dbt or Dataform were developed, data engineers would often write their transformations using pure Python. It may be tempting to use custom code for data transformation, but it increases the risk of error as it is not easily reproducible and must be written every time the process occurs.
The data transformation layer transforms data into something more useful and prepares it for the analytics stage.
The Semantic Layer Is Still Emerging
The semantic layer (commonly referred to as metrics layer) of modern data layers serves to aggregate key metrics critical for business operations, providing one source of truth when it comes to metrics such as "customers" or "active orders." To meet shared goals effectively and achieve shared success for all operations within an enterprise, having one source of truth for key indicators like customer count or active orders becomes critical for reaching goals efficiently and on schedule.
Looker and LookML pioneered semantic layer technology years ago; however, their implementation within their BI layer created silos for users who did not interact with it (typically data scientists working in machine-learning stack).
Recently however, dbt announced its intent to claim and make semantic layer a central piece of their modern platform.
Data Observability
Data pipelines have grown increasingly complex as organizations rely on information for decision making. Organizations now rely on accurate ingestion, storage and transformation into useful, trustworthy information to make critical business decisions - the need to ensure accurate ingestion is paramount; data downtime no longer acceptable to organizations due to incomplete, inaccurate, missing or false data being presented as being correct and complete information being received at once; applying DevOps best practices ensures data pipelines can be utilized and utilized immediately without interruption from untrustworthy data pipelines; this ensures data that can be utilized immediately for use and action taken upon by both users as well as management decision makers alike.
The data observability layer should be able monitor and alert on the following pillars:
- Data freshness- Is the data current? When was it last generated? What data upstream is included/omitted?
- Distribution - Does the data fall within acceptable ranges? It is properly formatted. Is the document complete?
- Volume- have all the data been received?
- What is schema?- How has it changed over the years? Who made these changes, and why?
- Lineage- what are the downstream assets and upstream sources that are affected by a data asset? Who is the person generating these data and who relies on them for decisions?
Data Analytics solutions for data observability provide quick and seamless connection to your data platform, enabling you to observe all events.
Automatic Monitoring ensures compliance and security standards can be met at scale across your datastore, scaling to accommodate even large volumes of stored information.
Data Discovery
Most leaders opt to build platforms by compiling lists of data. Unfortunately, we believe this approach no longer suffices.
Dont misunderstand me: data catalogs and teams today require reliable and scalable ways of understanding and documenting critical assets.
With data becoming ever more complex and real-time access becoming the priority, supporting technologies and processes must evolve alongside their platform counterpart.
Data discovery fills in where traditional data catalogs leave off (i.e. manual, low scalability and lack of support for non-structured data), taking over where traditional catalogs fall short (e.g.
manual entry of records or support for unstructured data). Data discovery acts like your smartphone GPS system - constantly up to date with insights and information available today.
Data discovery should at a minimum address the following requirements:
- Self Service Discovery And Automation: Without a dedicated team, data teams should be able to use their data catalog. Data tooling that enables self-service, workflow orchestration, and automation removes the silos in your data pipeline and makes it easier to access and understand data. Data adoption increases when data is more accessible, which reduces the workload for your data engineers.
- Scalability With Data Evolution: As companies continue to ingest data, and unstructured data is becoming the norm, your ability to scale will become increasingly important for the success and sustainability of data initiatives. Data discovery uses machine learning to get a birds-eye view of your data assets, as they grow. This allows you to adapt your understanding as your data grows. Data consumers can make better decisions based on the latest information, rather than relying solely on outdated documentation.
- Real Time Visibility Into Data Health: Data discovery gives real-time insight into the current state of data, not just its "cataloged", or ideal, state. Discovery includes how data is ingested and stored, aggregated and used by users. You can gain insights into data such as outdated data sets that can be deprecated or whether a data set is of production quality.
- Supporting Governance And Warehouse/Lake Optimisation: A range of tools and technologies are frequently used to query and handle data in the lake from a governance perspective. There is often no single source of truth (as there would be in a warehouse) for both reads and writes. The central source of information can be a data discovery tool.
Data discovery empowers teams to be confident that their assumptions are based on reality. This allows for dynamic discovery, and a high level of reliability in your data infrastructure regardless of domain.
Additional Data Platform Layers
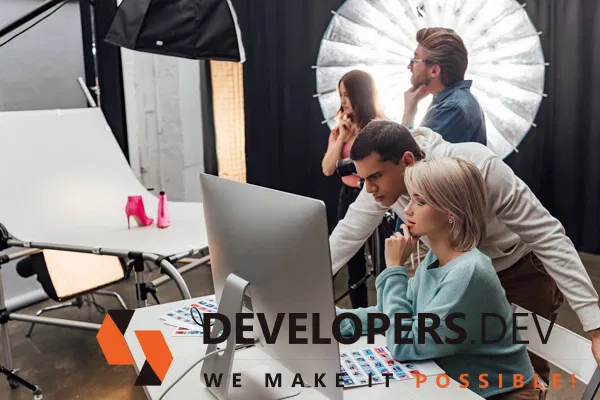
Data Catalog
Data catalog solutions have become an integral component of data governance and compliance for some organizations, providing descriptive data on tables, key metrics and more automatically updated from modern data platforms.
Imagine having your own personalized encyclopedia!
We have compiled a list of the top tools for data catalogs available today in 2023.
Access Management
The data on your modern data platform should be accessible but only by those with a real need for it. Access management solutions are becoming more important to protect sensitive data and PII, as well as avoid fines under regulations like GDPR or CCPA.
Vendors that are active in this market include:
- Immuta- Eliminates the complexity in managing and enforcing policies for data access so that organizations are able to speed up data access without compromising security.
- BigID- A modern and extensible platform that provides privacy, protection and perspective for all data.
- Privacera- Automates data management, security, and policy across multiple cloud services using a single interface.
- Okera- Provides a variety of enforcement patterns, platform-agnostic data access control and policy-based data management. Policies are enforced consistently in all environments.
- SatoriCyber - Decouples data security, privacy, and access control from the data layer. This allows data teams to work faster.
Machine Learning and AI
This category represents an umbrella under which we will gradually explore solutions for deploying and maintaining machine learning applications, including notebooks, ML OPs and Observability solutions.
Buy Or Build Your 6-Layer Data Platforms? It Depends
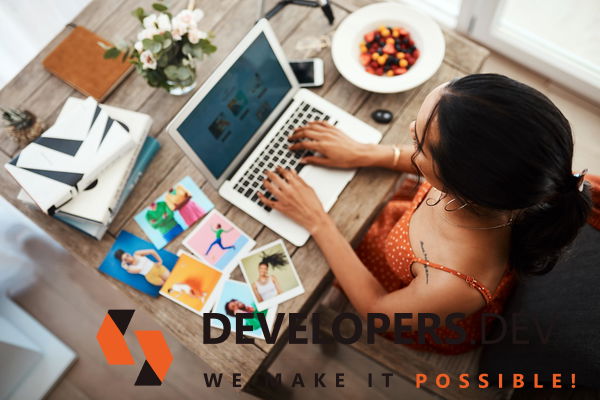
Building a data platform can be complex. There are several factors to keep in mind during its creation; one of the toughest choices facing our customers when building their platform is whether to develop specific layers themselves, utilize SaaS solutions or turn to open source for help.
Whats Our Answer? Unless your business is Airbnb, Netflix or Uber; all three should be considered when creating an effective data strategy.
Freshlys Senior Data Director shares how his company built their 5-layer data platform.
Each of these solutions has pros and cons, but the decision you make will be based on a number of factors, such as:
- Size of your data team- Your data engineers and analysts already have enough on their plates. Asking them to develop an internal tool could cost both money and time more than anticipated; lean data teams dont have time or budget for training new team members on in-house tools created from scratch; non-Uber/Airbnb/Netflix data teams tend to invest more heavily in flexible, automated or user-friendly solutions that they find accessible instead.(i.e., open-source or low-code/no-code SaaS).
- How much data your company stores and processes- Select a solution that can grow with your company. A data analyst working at an office of 20 would unlikely opt for an expensive transformation system costing $10K annually when only needing a few lines to fulfill a task.
- The budget of your data team- Open source solutions may be an ideal fit for teams operating with limited funds and numerous employees, though setup and implementation tends to fall on one person alone. You must rely heavily on community members or project creators when selecting and using open-source projects; only two percent grow beyond their first few years! Nonetheless, when making decisions using this route it must be approached carefully, only 2 percent of projects grow after several years have lapsed.
- Who will use the tool?- It might be more cost-effective to develop the tool if it is intended for data engineers. You might want to buy a collaborative and user-friendly tool if you have stakeholders from all over the company.
- What data problems does the tool solve?- It makes sense to develop the solution internally if the use case is very specific to your company. You might be able to benefit from a third party vendors expertise and experience if the tool solves a problem that is common in your industry.
- What is your data governance requirement?- Data governance will become an increasing focus for organizations in 2023. To meet your business requirements and remain compliant with regulations such as GDPR and CCPA regulations. Some firms that work with highly sensitive data feel more at ease developing customized solutions themselves in order to comply with various legal obligations.
No matter which path you take, laying out these layers gives you the foundation for growth and scaling, and most importantly, will deliver insights and products that your company can rely on.
How To Create A Data Analytics System
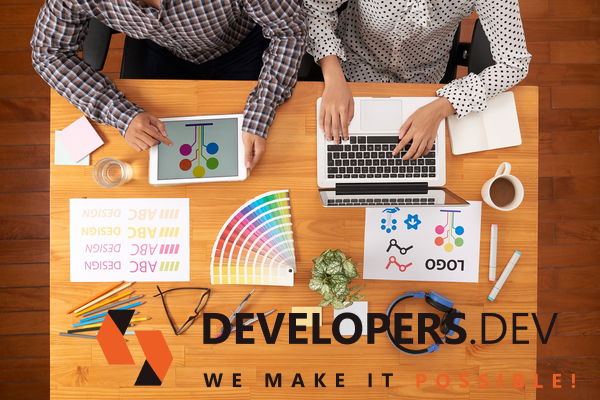
You should follow the SDLC (Software Development Lifecycle) to create a data analysis platform from scratch.
Discovery Phase
The discovery phase is essential to a successful software project. You can achieve the following by starting your software development process with a phase of discovery:
- Get a clear idea of what you want to create
- Validate your business idea to ensure your product meets market demand
- Find the best technology to implement your idea
This phase typically lasts around two weeks and involves business analysts (typically), project managers, designers of user experience (UI/UX), and technical specialists who assist stakeholders in setting product vision, developing requirements for software development projects, building prototypes of analytics platforms as well as providing estimations of time and costs associated with creating analytics platforms.
App Architecture And Design
Once stakeholders and software teams have developed a list of requirements, a Software Architect is brought on board to work on designing the logic and architecture of an app.
A skilled Software Architect knows technology inside out; therefore they offer tailored solutions tailored to every application they design for.
At this stage, UI/UX designers also play an essential part. It is vitally important that before development begins, its clear how your data analysis system will appear; at this time you should pay special attention to its design, making sure its UI and UX meet with your approval - missing some details in its initial design phase will require extra money and time in terms of making changes later on.
Once your architecture, user interface (UI/UX design and development have all been completed successfully, you are free to begin development of your product or website.
MVP Development
Minimum viable products (MVPs) are essential in building any software product successfully, serving as the initial version with all features necessary for satisfying user needs.
An MVP (Minimum Viable Product) is a simple product created using backend, frontend, and quality assurance testing in order to give users what they desire from an experience standpoint.
An MVP allows stakeholders to test out ideas they believe in while meeting the needs of their target audiences - and public MVPs enable stakeholders to gather user feedback while gathering improvement feedback to enhance analytics platforms.
Maintenance and Support
An MVP data analytics platform represents just the first step on your software development journey, much like Facebook has continued its development journey since 2004 when their first version launched.
You dont want your work to stop once an MVP has been completed - technology changes constantly so staying abreast of market changes is vitally important!
The Conclusion Of The Article Is:
Data analytics platforms are essential tools for organizations looking to make intelligent decisions and gather insightful intelligence.
Businesses require powerful analytics tools in order to identify patterns and garner insight from the overwhelming amount of digitally produced information. By investing in flexible, scalable and user-friendly data analysis platforms, organizations can expand their decision making abilities while streamlining data management processes.
Businesses leveraging data analytics platforms enable their business to harness machine learning and artificial intelligence technologies for automating data analyses, producing real-time insight, meeting their strategic goals and creating actionable insight from raw data. With data analytics constantly developing and evolving, it is imperative that businesses keep abreast of emerging technologies to stay ahead of competition and gain a competitive edge.