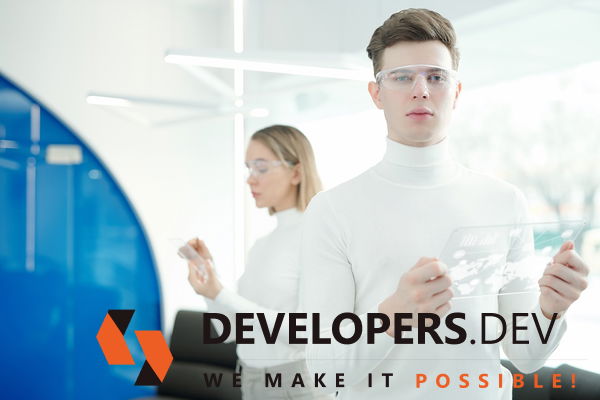
Big Data: A History
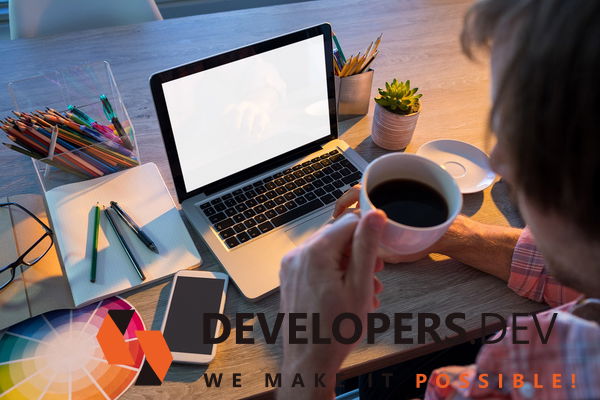
Handling and processing data collected during the census would require eight years, which reduced the number of calculations required.
The 20th century saw a rapid growth of data. The evolution of big data has now taken place.
At the time, computers, scanners for message patterns, and magnetic storage devices were developed, to store tax returns and millions of fingerprint sets, the US Government built the first data center.
Big Data Is A Term That Has Been Thrown Around A Lot
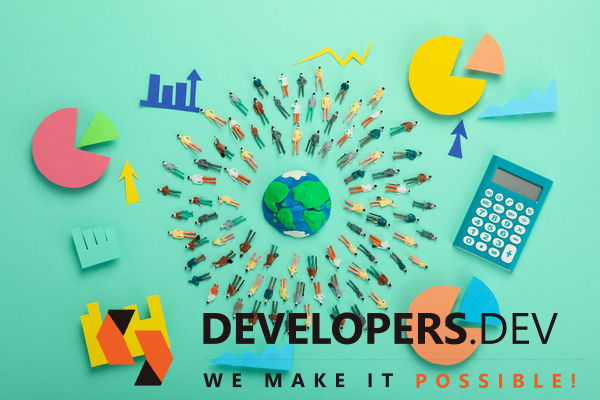
Big Data refers to high volumes, high speeds, or high variety information assets which require new processing methods to enhance decision-making, insights discovery, and processes optimization.
The phrase big data speaks for itself - it is a group of large data sets that are too big to be processed by normal computer techniques.
This term refers not just to data but to all the tools and frameworks that are involved. The advent of social media and other new communication channels, as well as new devices that are more powerful and capable of handling data, have created a new challenge for industry players.
The entire planet had only five gigabytes of data from the dawn of time, the same volume of data was produced in only two days, the same volume of data was produced every 10 minutes.
This is why its not surprising to see 90% of the global data generated in just the last few years. Big data can be a useful tool when it is processed. However, this information was largely ignored before big data.
Big Data: The Three Vs
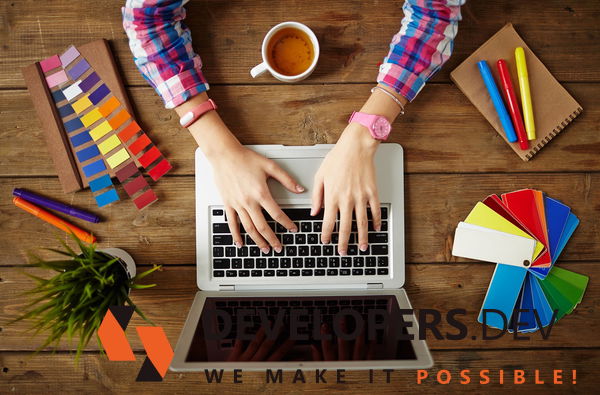
The Volume
Lets start with one of the more obvious ones. Quantity is the key to big data. Data volumes that could reach previously unimaginable levels.
According to estimates, there will be over 40 zettabytes by 2020. This represents a 300-fold increase. Terabytes, and sometimes Petabytes, of data are stored in servers and storage.
This data is used to track success and help shape a businesss future.
Velocity
Data has changed our perceptions of its importance and expansion. Data was once underestimated in business, but now we rely heavily on it.
The Velocity metric measures how fast data enters the system. Some data may be delivered in large batches while others arrive intermittently. Since not all systems process data in the same way, it is important to refrain from making assumptions until you have all of the necessary information.
Variety
Data was once only available in one format and from a singular source. Data was previously delivered in traditional formats like csv and Excel files.
Now, data is being sent via wearable devices, social media, video, text and pdf files. This data can be very useful, but it requires more work and analytic skills to manage, interpret, and use it.
Data has increased dramatically with the growth of social media, apps, and online businesses. Social media sites attract and interest over one million people daily.
This means that data is being collected at a greater rate than ever. How is all this data processed, stored and handled? Here is where Big Data enters the picture.
Big Data Analytics has transformed the IT field, adding value and enhancing organizations. This involves analytics and new-age techs such as machine learning, mining statistics, and others.
It can be used to help teams and organizations perform many operations using a single platform. They can store Tbs worth of data and pre-process them, then analyze and visualize all of it.
What is Big Data?
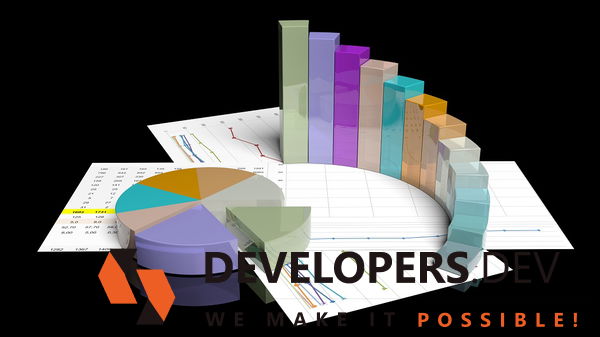
To make data-driven decisions, analytics of big data requires identifying trends, patterns and correlations in vast quantities of raw data.
These methods apply well-known statistical analyses, like clustering and regression, to large datasets using more modern instruments.
Data Collection
Each company takes a different approach to collecting data. Modern technology allows businesses to gather unstructured data and structured information from many sources.
These include cloud storage, mobile applications, IoT sensors in stores, etc.
Organize your Data
To ensure that analytical queries are accurate, it is important to organize data once it has been collected and stored.
This is especially true if data collection and storage are large and unstructured.
Clean Data
To improve data quality, all data must be cleaned, no matter how large. All data should be structured correctly, and duplicate or useless data should be removed.
Data that is dirty can conceal or deceive and lead to incorrect findings.
Google Trends and Google Finance are similar examples. These examples show that big data does not only refer to volumes.
The data is also diverse and fast, an analyst in the industry, articulated three Vs for big data: volume, velocity and variety.
Data is streaming at an unprecedented speed today, which makes it hard to keep up with. Data torrents are a necessity for smart meters, RFID tags, and sensors.
The majority of organizations find it hard to respond quickly to data. Back in the day, too much data meant that you had to store it. With increased storage capacity and lower storage costs, players in the industry, like Remote Support, are now focused on how relevant data can be used to create value.
Data is more diverse today than it was just a few short years ago. The data is classified into three categories: structured (relational), semi-structured (in the form of XML sheets) and unstructured (media logs, Word and Text documents, as well as PDF files).
Many businesses are faced with the challenge of governing, managing and merging different types of data.
Data also has to have other characteristics, such as honesty (the accuracy of the data), complexity (when dealing with large amounts of data coming from multiple sources), and variability.
To become a Big Data Engineer, you must first understand what Big Data is and where it comes from.
Types Of Big Data
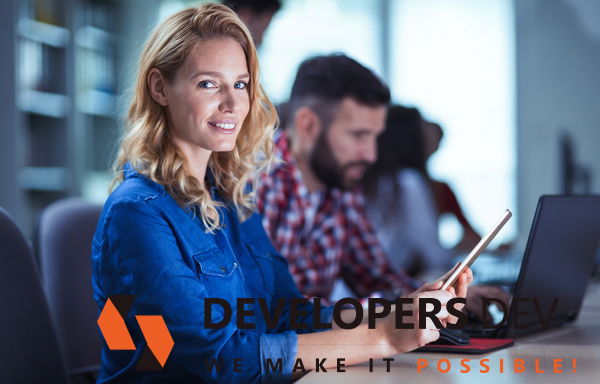
Structured Data
Data that is organized is easier to sort and evaluate because it is predetermined and has been provided with a schema or structure.
Since each field has been specified, it can be accessed independently or in conjunction with data from other fields. Structured data has become very important because of its ability to collect data quickly from multiple database locations.
Data That Is Not Structured
Unstructured data is information without predefined conceptual meanings, which makes it difficult to understand or analyze by conventional databases or models.
Unstructured data includes facts, numbers, and dates. Big data includes video and audio files as well as mobile activity, satellite images, and more.
Semi-Structured Data
Semi-structured data is a combination of structured and unstructured data. It has some of the characteristics and organization of structured data but lacks clarity.
Big Data Use Cases
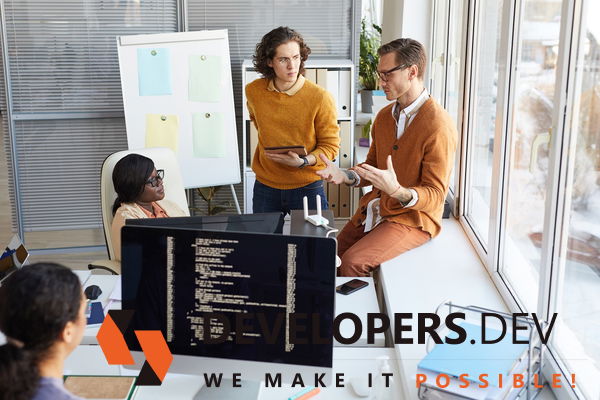
360° View of the Product
Businesses often use big data to develop dashboards that provide a 360-degree view of their customers. The dashboards collect information from internal and external sources and evaluate it.
They then present it in a manner that helps customer service, marketing, or sales staff with their jobs.
Enhancing Client Retention and Acquisition
Businesses can use big data to understand their clients interests, patterns of usage, reasons for customers stopping using products or services and the reasons why they stop buying.
Big data apps can help businesses better understand what their customers want and how they behave. Then, they can use these patterns to improve their products and increase conversion rates.
Improved Cybersecurity and Fraud Prevention
Businesses are constantly fighting fraud. Big data analytics is used by organizations to identify fraud trends, abnormal system behavior, and criminals.
To identify, detect and minimize fraud, big data systems can sift data from servers, databases and apps.
Improved Forecasting and Pricing Optimization
Big data may not have the ability to predict the future accurately. Still, it allows companies to identify patterns and trends ahead of others.
Businesses can make adjustments to their supply chains if they detect problems early. Information on demand can be used to improve sales forecasting and help determine the best price before the product is launched.
Big data strategy helped businesses make better decisions because it provided them with information about the probability of certain outcomes.
Big Data Best Practices
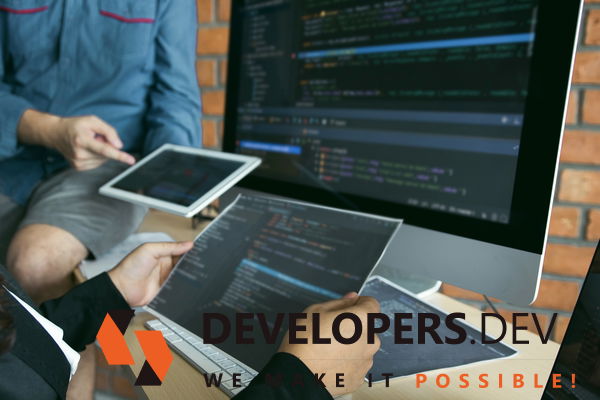
Analyzing and understanding the business and organizational requirements is the most crucial step to take before incorporating big-data analytics into projects.
Finding out which data types are entering and being produced within the company is the second best way to use big data. The third step is to understand and analyze whats missing. Determine if any additional information is needed for the project after gathering all necessary data.
After gathering and analyzing data from different sources, its now time to determine which technologies are best suited for your firm, including predictive analytics, streaming analytics, fraud detection and data preparation.
Big Data Examples
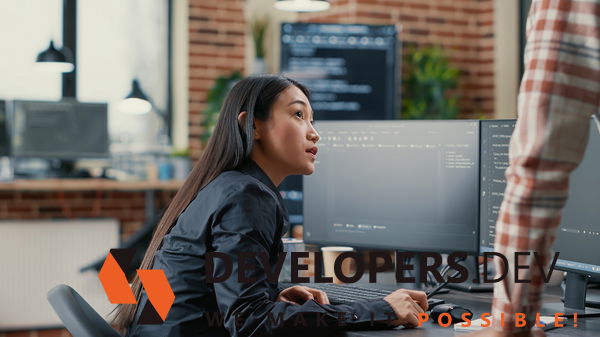
Transport
GPS applications on smartphones use a large amount of data to help you get from A-B in the least amount of time.
Satellite photos and government organizations are the two main sources of GPS data.
Advertisement
Advertising and marketing campaigns have always been aimed at specific customer groups. Marketers have tried to predict consumer reactions to ads using focus groups, surveys, television and radio preferences and other methods.
The techniques used were at best, educated guesses.
Financial and Banking Services
Fraud Detection the banks track the spending patterns of their customers and look for abnormal behaviors and transactions.
Management of Risk big data analytics allows banks to monitor, report, and analyze company metrics and employee behavior.
The Government
Many government organizations, especially at the local level, do not use the latest data analytics and mining tools.
The IRS and Social Security Administration are two organizations that use data analytics to detect fraudulent tax claims and disability claims.
Big data is used by the FBI and SEC to monitor markets and identify unethical business practices.
Read More: All You Need To Know About Big Data
Big Data: Benefits
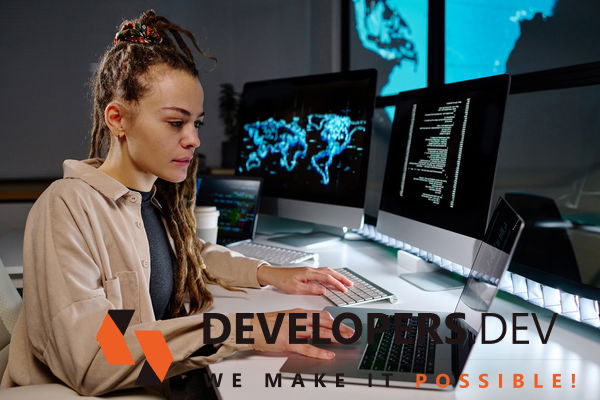
The consumer of today is extremely demanding. The consumer is very demanding today. He uses social media to talk with other customers and considers different options.
The customer would like to be acknowledged and treated individually after purchasing a product. You can engage your customers in real-time by using actionable information from big data. Big data will allow you to perform this task by checking a customer profile and getting information on what they are complaining about in real-time.
Then, you will be able to perform reputation management. You can use big data to improve the services and products you sell. You can use social media text to learn what people think of your product.
You can use big data to compare different variations of computer-aided designs (CAD) to see how small changes will affect your product or process.
Big data is, therefore, invaluable to the manufacturing process. You will always be one step ahead of the competition with predictive analysis. This can be done by analyzing and scanning social media and newspaper articles.
You can also use big data to perform health tests on customers, suppliers and other stakeholders to reduce risk, such as default.
Big data helps keep data safe. The use of big data tools helps you to map your data landscape, which helps analyze internal threats.
You will be able to see if sensitive data is protected or not. You can flag emailing and storing 16-digit numbers, which could be credit card numbers. Diversifying your revenue streams is possible with big data.
You can get trend data by analyzing big data. This could lead you to a new revenue stream.
If you want to succeed in the competitive online market, your website must be dynamic. Big data analysis allows you to personalize your websites look and content based on visitor characteristics such as gender and nationality to power its features "People you might know" and "Frequently purchased together".
Big data can be important for a manufacturing company because it will allow you to avoid replacing technology due to the length of time theyve been used.
It is both expensive and inefficient to replace parts based on their age. The use of big data will allow you to identify devices that are failing and predict the time when they should be replaced.
The healthcare industry is still one of those few that is stuck in a conventional, generalized approach. If you are diagnosed with cancer, for example, your doctor may recommend a different treatment if the first one doesnt work.
A cancer patient can get a medication developed according to their genetics based on big data.
Big Data: The Challenges
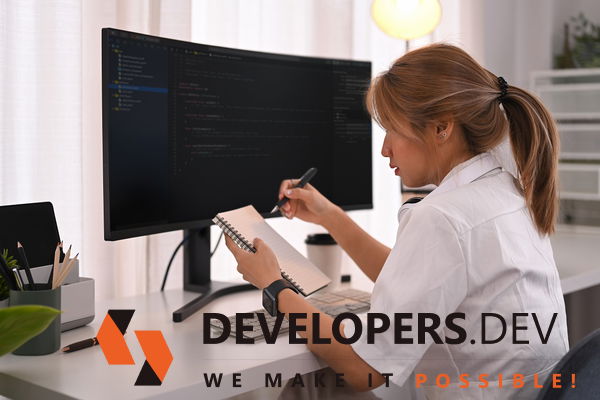
The exponential growth in raw data can be a problem with big data. Data centers and databases are storing huge volumes of data that are growing rapidly.
Organizations often struggle to store data properly due to the rapid growth in data.
Next, you need to choose the best Big Data tool. The choice of the right Big Data tool is crucial. There are many options, but choosing incorrectly can lead to wasted time, effort and even money.
The next challenge for Big Data is to secure it. Many organizations spend so much time analyzing and understanding the data that they neglect to secure it. This leaves the security of the data for later.
Unprotected data becomes a breeding ground for hackers.
This article should have helped you to understand Big Data. If you still want more information, you can read another piece titled "Is Big Data overhyped?" This article goes into greater detail about the value of technology and how it is hyped.
Why Your Software Should Apply Big Data
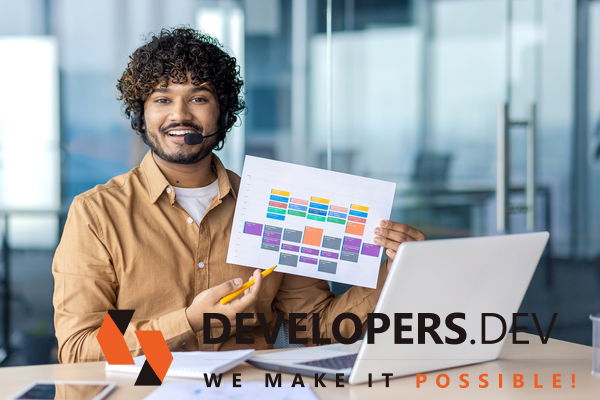
It is important to discuss all of the advantages that Big Data technologies can bring to your company.
Savings In Time/Costs
Big Data can help you to identify the problematic areas that we discussed earlier. You will be able to identify the reasons behind them and optimize your business processes in order for them to become more profitable and attractive.
Risk Management
Predictive analytics can help you know what risks are coming up for your company. You will also be able to know the problems that your competitors are facing and prevent their mistakes.
Better Decision-Making
Big Data software can take the decision-making process to an entirely new level. In turn,
Product Quality Can Be Improved
Understanding the preferences and needs of your customers and analyzing their data will help you to create products that they are likely to want and use.
Innovation: A Contribution
Your business will be able to incorporate the innovations that customers expect through research and development using Big Data Analytics.
Stay Competitive
Finally, youll be able to know which trends are going to dominate your field in the future. You can use this information to gain an advantage over competitors.
You will also have more insight into the buying habits of your customers.
Read More: Big Data: What are they?
Big Data and Software Development: How they Interrelate
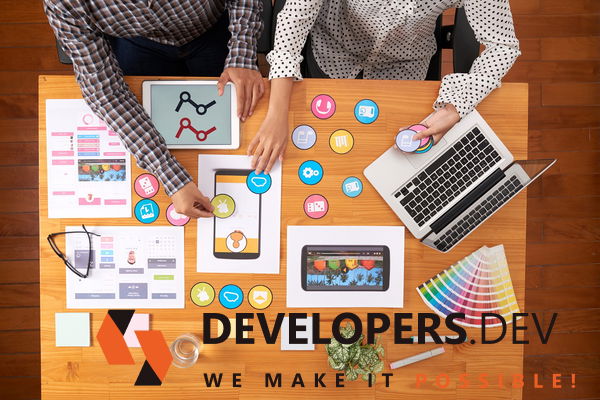
Businesses generate exabytes and exabytes worth of data, which they can access from a variety of sources. To fully exploit all of this information, enterprises must analyze, recognize patterns and gain actionable insight.
The only way to gain a competitive edge is with organized and quality data. This will improve forecasting accuracy, facilitate decision-making, and accelerate all business processes.
Big Data Software Architecture
To leverage Big Data, it is essential to have a software development architecture that assembles the data and analyzes it.
Software developers start by creating strategies using Data analytics software, which generates reports based on data.
When building architectures for software in Big Data, the typical method is to build them gradually. Big Data architectures are event-driven and data-centric.
They test each software component and then analyze the results. The process is then repeated to fix any detected bugs and to redesign the final product.
Big Data Development Services
In the last two decades, IT outsourcing has increased Big Data Development development is not an exception, analysts of data, project managers and account managers.
Other reasons to consider looking eastward include the reduction of development costs, improved quality, and a wider business reach due to time zone differences.
Data Strategy
The Big Data Market will double in size within five years. Data continues to present many challenges, including disorganized processes in business, the failure to identify basic business requirements, as well as data quality, integrity, and privacy.
A company can suffer from inefficient planning, but a data strategy that addresses all issues can be a solution.
To manage massive volumes of data, organizations must work with reliable and experienced service providers. When you partner with data scientists, engineers and analysts who are experts in their fields, you can expect to see innovation and agility.
You will also experience a better user experience and be able to comply with regulatory requirements. Scalefocus will help you create a strategic, holistic approach that maximizes your data.
DB Architecture and Design
To improve the quality of data and optimize resources, enterprises need to structure and organize their database.
It is the service providers job to standardize data protocol and define database objectives. This would not be possible without updating legacy tools and systems. Updating data is essential to utilize the capabilities of modern technology fully.
Our data teams are experienced with a wide range of databases, use cases, and business requirements. We also provide solutions that meet the performance, security, and business needs of our partners.
This expertise covers relational and non-relational databases on-premises, vendor-specific cloud databases, OLTP and OLAP solutions, and custom solutions.
Data Modelling
The data modeling process incorporates local and national policies, business rules and regulatory compliance. It also ensures consistency with naming conventions and default values.
The purpose of data modeling is to understand relationships in a database/warehouse and reduce development errors.
Scalefocus uses top-of-the-line tools such as ER/Studio and Toad Data Modeler to ensure the visual presentation of data.
We can then help accelerate our partners database mapping and design.
Cloud Migration: From On-Prem Infrastructure
The Public Cloud Market revenue is expected to reach USD 650 Billion by 2025. These staggering figures should not be surprising as remote working and COVID have accelerated the digital transformation.
The majority of businesses are migrating their on-premise data to the cloud in order to cut costs associated with on-premise infrastructure and servers. Cloud technology will be around for a long time as it offers flexibility, scalability and agility.
When migrating from the on-premises to the cloud, there are many challenges. Data regulations, performance quality, and data security, for example, can all be a problem.
Scalefocus remote development centers have experience in creating and managing cloud-to-on-prem migration strategies for different industry and technology environments. This includes AWS, GCP Azure and IBM Cloud.
Advanced-Data Analytics
Companies can increase their business value by gaining data insights through various content sources. To extract data and detect anomalies, and predict future events, predictive modeling, deep learning, artificial intelligence and business process automation are used.
Data analytics can improve decision-making and management of risk by providing deeper insight into data. Scalefocus offshore and nearshore teams employ techniques such as data mining, machine learning, and big data analytics and have expertise across different industries to manage Big Data infrastructure, perform predictive analytics and forecast efficiently.
Machine Learning and Artificial Intelligence
The global AI market revenue will surpass the $500 billion mark by 2024. Machine Learning and Artificial Intelligence have been quickly recognized by enterprises as a way to increase efficiency, improve business results, and gain a competitive edge.
When faced with massive amounts of diverse data and multiple sources from which to gather it, planning and making decisions can be difficult. ML and AI allow companies to make decisions and forecasts based on quantitative evidence, as they can identify patterns and anomalies that human experts are unable to detect.
ML, AI and advanced analytics to help our partners transform their business models. We do this by introducing effective processes for data management.
The expertise of our remote software development centers and the cutting-edge technology they use have enabled numerous companies to extract insights from data, make result-oriented decisions, and improve their customers experience.
Want More Information About Our Services? Talk to Our Consultants!
Conclusion
Agile Software Development and Big Data solutions are interdependent.
The software engineers, analysts and project managers of the Scalefocus offshore and nearshore development centers are adopting a modern approach to analytics and data. Contact us to learn more about how we can help your company overcome any challenges it may be experiencing with digitalization or datafication.