
Narrow AI refers to artificial intelligence designed specifically to solve specific problems or complete specific tasks, like virtual assistants Siri and Alexa and recommendation systems on online platforms.
By contrast, general AI describes systems with human-like intelligence capable of performing any intellectual task a person might perform; unfortunately this type of AI still doesnt exist yet - an ambitious long-term goal in AI research and development.
AI has experienced remarkable strides over recent years due to improvements in computing power and availability of large datasets, as well as refinements to machine learning algorithms - one subset of AI called machine learning playing an essential part in helping computers learn and adapt over time.
AI can be found everywhere today - from chatbots and virtual assistants to medical diagnosis tools, trading algorithms and financial markets.
AI technology could transform industries while improving human life; its influence may even shape techs future direction. While this emerging tech holds great promise for human advancement and shaping the future of tech development - there may also be significant ramifications that must be considered carefully before being adopted into society or shaping technologys direction.
Data Insights: Artificial Intelligence Solutions
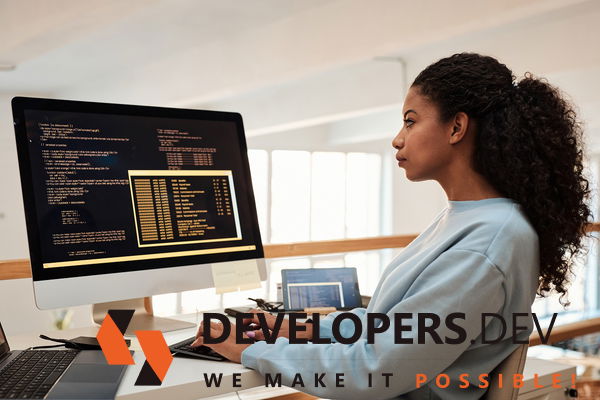
Data has long been considered a commodity in our modern digital era. Harnessing its power to maintain competitive advantages for organizations and businesses requires harnessing data insights derived from vast datasets to make informed decisions, detect trends and uncover hidden opportunities.
Artificial Intelligence solutions play a pivotal role in turning raw data into useful knowledge that can improve operations, boost customer service, encourage innovation and foster change. In this article we explore data analytics as well as various AI technologies and techniques utilized to obtain valuable information from data sets.
Artificial intelligence continues to revolutionize businesses and industries worldwide. AI models become more adaptable and efficient with techniques like transfer learning and reinforcement learning.
Explainable AI has become ever more essential as companies look for better insights and understand AI-powered recommendations and decisions.
In order to maintain trustworthiness with data insight and public confidence, practices involving ethical AI practices should also become increasingly prominent.
Forecasting and time series analysis enable organizations to make accurate predictions that lead to improved decision making.
With AI technologys continued advancements, data insights will become ever more sophisticated, expanding opportunities within data-driven decision making as it unfolds further. AI solutions offer organizations a competitive edge by helping them navigate todays digital environment more easily while leading towards success and lasting achievement.
Data insights have become an integral component of modern business. All industries gather massive amounts of information through customer interactions, IoT devices, business processes and social media - it is crucial that industries mine this data to gain meaningful insight and make more informed decisions and improve products, services and operations.
Data analysis used to be time consuming and manual. Thanks to AI insights in data, businesses now can process large volumes quickly while uncovering useful insights that drive innovation and growth for innovation purposes.
AI algorithms can identify anomalies, patterns and correlations that human analysts would miss giving businesses valuable intelligence that propel innovation and expansion.
Artificial Intelligence for Data Insights
AI technologies such as deep learning, machine learning and natural language processing (NLP) are the foundation of any data insight solution.
Explore these AI techniques to learn how they can help you extract valuable insights from your data.
Machine Learning (ML)
A subset of AI, machine learning focuses on the development of algorithms which can make decisions or predictions without explicit programming.
Machine learning uses supervised, unsupervised, and reinforced learning.
Supervised learning algorithms can perform tasks such as classification and regression. In customer churn predictions, for example, a model that uses supervised learning can use historical data to determine which customers will likely leave in the future.
This helps businesses retain these customers.
Algorithms for unsupervised learning are used to perform tasks such as clustering and anomaly identification. Unsupervised learning is useful in customer segmentation.
It can help to group similar customers, allowing for targeted marketing.
Natural Language Processing
NLP, a branch within AI, aims to enable machines to interpret and create human language. NLP is crucial to processing unstructured information, like text documents, customer reviews, or social media posts.
Sentiment Analysis is one of the most popular NLP applications in Data Insights. Algorithms analyze customer feedback to determine sentiments or opinions regarding products and services.
The analysis can help businesses to identify improvement areas and better understand the preferences of customers.
Want More Information About Our Services? Talk to Our Consultants!
Deep Learning
A subset of Machine Learning, Deep Learning uses artificial neural networks to solve complicated problems. Deep learning models like convolutional and recurrent networks are used in data insight for tasks such as image and video analyses, speech recognition and translation.
These models extract useful information from multi-media data and enrich data insights.
Data Preprocessing & Feature Engineering
Data preprocessing, feature engineering, and data feeding into AI models are all crucial parts of the pipeline for data insight.
The data preprocessing process involves cleaning, transforming and normalizing raw data to prepare it for analysis. This phase deals with missing values, noise, and outliers.
The feature engineering process is the selection and creation of relevant features that are then used for training AI models.
AI algorithms are highly affected by the quality of their features. To create predictive and informative features, feature engineering requires domain expertise and a thorough understanding of data context.
Big Data Scalability
Big data is often a massive dataset that requires advanced technologies and tools to process and handle. AI-based solutions for distributed computing and scalability, like Apache Hadoop or Apache Spark, can be used to analyze and process large amounts of data.
The technologies enable organizations to increase their data insight capabilities as data volume increases. This allows them to continue extracting valuable insights without compromising performance.
Real-Time Data Analysis
Real-time insights into data are a must in todays business world. AI technology, combined with real-time processing frameworks, allows organizations to analyse streaming data, providing instant and actionable insight.
In industries such as healthcare, finance and e-commerce, real-time data insight is used. Real-time data insight can help detect fraud in financial institutions in real-time and minimize potential losses.
Data Visualization And Storytelling
Presenting insights gleaned from data is just as essential to its analysis. Visualization techniques like interactive dashboards and charts and graphs play a pivotal role in relaying complex information to decision-makers and other key players effectively.
Data storytelling, using insights gleaned from data to craft narratives with strong messages. Narrating makes understanding insights easier for audiences who may not possess technical savvy - data visualization with storytelling combined can assist organizations with taking data-driven decisions at every level of decision-making.
Privacy and Ethical Concerns
As data insight becomes more widespread, privacy and ethical concerns become ever more prominent. To safeguard individuals rights when AI analyzes personal data, stringent privacy protection measures must be put in place in order to maintain data anonymization, encryption, GDPR compliance as well as data protection laws are vital components.
Ethics in data insight entails transparency of AI decisions, avoidance of bias in algorithmic models and responsible application of insights.
Organizations seeking to foster trust among their stakeholders must find an equilibrium between using AI technology and adhering to ethical regulations.
Real-time data insights offer organizations instant feedback that helps make important decisions quickly. Data visualization and storytelling helps stakeholders grasp complex information effortlessly and engage them to take part.
Privacy and ethics remain critical as data insight shapes business strategies. To foster trust in AI systems, AI must follow ethical AI practices such as data anonymization and privacy regulations to build public confidence in this area of technology.
Data insights and AI solutions offer great potential to fuel innovation across industries and help propel economic expansion.
Furthermore, organizations using them effectively may remain competitive in todays digital environment by taking full advantage of data to remain ahead of competitors in this era. As AI advances further still, data insights become even deeper and more useful information from an ever-expanding trove of information.
The Interpretability of AI
Explainable AI is becoming more important as AI models get increasingly complex. Explainability is the ability to interpret and understand how AI models make predictions and decisions.
Explainable AI in the context of data insight is critical for building trust and understanding factors that influence the insights.
Data analysts and decision-makers can validate insights from interpretable AI models and detect biases. AI explainable techniques such as saliency and feature attribution provide insight into the features that are most important in the predictions of a model.
Interpretable AI models are increasingly being used by organizations, particularly in applications such as healthcare, finance and autonomous systems.
Transfer Learning And Pre-Trained Models
Transfer learning, a powerful AI technique, uses the knowledge gained by training one model to enhance the performance of a related model.
Transfer learning is a powerful technique that can be used to use pre-trained AI models, trained with large datasets, for specific tasks.
Read More: What Is The Most Intelligent Ai Software In The Market Today?
Pre-trained models, like BERT (Bidirectional encoder representations from Transformers), can be tuned for NLP tasks such as sentiment analysis or question answering.
Transfer learning allows models to be trained without extensive computation and data resources. This speeds up the data analysis process.
Time Series Analysis & Forecasting
In industries such as healthcare, finance and manufacturing, time series data is common. When dealing with time-dependent data, analysing time series and forecasting becomes essential.
AI models such as Autoregressive Integrated Moving Average (ARIMA) and Long Short-Term Memory Networks (LSTM) are used to analyze and forecast time series. The models can identify trends and patterns within the time series data. This allows businesses to take informed decisions by using future predictions.
Re-Enforcement Learning For Optimization
AI Reinforcement Learning (RL) involves agents learning to act in a particular environment to maximize a rewards signal.
While RL has been associated with robotics, gaming and other applications, the technique is also used to optimize data.
RL algorithms are used to optimize supply chains, business processes and resource allocation. RL algorithms can be used to optimize inventory management based on patterns of demand, thereby reducing stockouts and holding costs.
Federated Learning
In AI, federated learning has emerged as a new approach that allows AI models to train across decentralized servers or devices without sharing the raw data.
It is especially useful for sensitive data as the technique allows data to be centralized in one place, and models can then be collaboratively trained.
When organizations and entities want to gain insights from data, federated learning can be useful. Data collaboration is encouraged while data protection and ownership are maintained.
Artificial Intelligence Trends for 2023: Data Insights
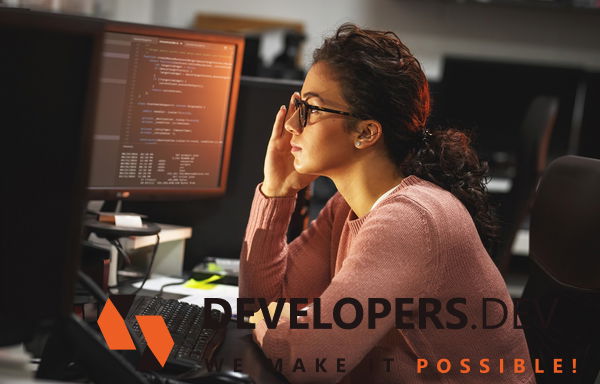
As we are an artificial intelligence model of language, we do not have access to real-time events or data since September 2021; thus we cannot make specific predictions for 2023 but may offer insight on potential trends based on their development since my last update.
AI solutions have experienced exponential growth over the years, revolutionizing how organizations use data insights for decision making and innovation.
Deep learning technologies combined with natural language processing (NLP) play an instrumental role in unlocking knowledge from vast datasets.
Artificial intelligence continues to advance rapidly. 2023 will likely witness major advances, with AI model improvements, integration with IoT devices and edge computing, plus greater personalized insights driving innovation throughout all industries.
AI-driven maintenance and federated learning both aim at optimizing operations for industrial sectors. Improved natural language processing will enhance user experience while responsible and ethical AI will safeguard data privacy and fairness.
Automating data insight workflow and gaining real-time insights will enable organizations to take informed decisions quickly.
Augmented analytics makes these insights even more accessible, allowing a broader set of users to take advantage of them.
Data-driven decision making will become an indispensable skill as AI technologies mature and businesses harness its insights, helping them remain competitive while responding quickly to market shifts.
While trends provide glimpses into what may lie ahead, real developments will depend on factors like regulatory changes, technological advances and business requirements.
Though the specific trends that will dominate data insight and AI landscape in 2023 could shift and evolve over the coming years, several areas should likely play a part in shaping its direction.
We will examine some potential data insight trends for 2023.
Ai Models Are Advancing Continuously
AI is a rapidly evolving field, with AI models likely to be more efficient and sophisticated by 2023. AI models may be able to handle more complex data faster and with greater accuracy due to continuous advancements in transfer learning, deep learning, and reinforcement algorithms.
Further, explainable AI is expected to play a greater role in critical decision-making. AI models will be sought by organizations that offer not just accurate insight but transparency and interpretability.
This allows users to understand better why the model made its predictions.
Integrating AI with IoT, Edge Computing and Edge Computing
In 2023, the integration of AI and Internet of Things devices (IoT), as well as edge computing, will gain momentum.
Combining AI with IoT data can help organizations make better decisions by gaining real-time insight.
Edge computing allows AI to be processed closer to data sources, which reduces latency. This integration leads to more autonomous and intelligent IoT applications ranging from industrial automation to smart cities.
The Contextualization And Personalization Of Insights
In 2023, data insights will be more contextualized and personalized. AI-driven systems aim to tailor insights for specific audiences by understanding individual users' preferences, behaviour, and needs.
To gain contextualized insights, it is necessary to understand the context of data generation, including environmental factors, interactions with users, and current market conditions.
The result will be more actionable and relevant recommendations for both businesses and users.
Federated Learning for Privacy-Preserving Insights
In 2023, federated learning will be an increasingly popular trend. This is especially true in industries that are concerned about data security.
The use of federated learning allows AI technology to be developed across multiple devices and servers without having raw data shared.
Healthcare and Finance, two industries that deal with sensitive data, can use federated learning to gain insight from their datasets and collaborate without having to compromise data security and privacy.
AI Predictive Maintenance
AI-driven maintenance will become increasingly common in industrial sectors such as manufacturing and transport by 2023.
AI-driven predictive maintenance analyzes sensor data to predict the likelihood of equipment or machinery failing.
Predictive maintenance allows organizations to optimize their maintenance schedules and reduce equipment downtime.
This results in increased efficiency and cost savings.
Enhanced Natural Language Processing Applications
Natural Language Processing has advanced rapidly. In 2023 we should expect to see more sophisticated NLP apps. AI-powered virtual assistants and chatbots will be more intelligent.
Theyll understand natural language more accurately and offer more personalized answers.
Further, the sentiment analysis technology will evolve to allow businesses to gauge customer and public feedback better and to react quickly to new trends.
AI Data Analysis for Ethical and Responsible Data Insights
In 2023, as AI becomes more prevalent in the decision-making process, its importance will increase. In 2023, organizations will be focusing on developing AI models to mitigate biases and ensure fairness while upholding ethical principles.
Data privacy laws such as General Data Protection Regulations (GDPR) or other new data protection laws will force organizations to adopt AI privacy practices that ensure responsible usage of personal data.
AI-Driven Automation for Data Insights Workflow
In 2023, AI will play an increasingly important role in automating different aspects of data insight workflow. AI automation streamlines processes from data preprocessing, model selection and deployment to reducing manual intervention.
AI pipelines that are automated will be more advanced and allow organizations to analyse large datasets with less human input.
Data scientists and analysts can now focus their efforts on more complex tasks, such as improving models or business strategies.
Real-Time Data Insight to Inform Time-Critical Decisions
2023 will see real-time data insight remain an industry trend, particularly within industries where quick decisions must be taken immediately in time-critical situations.
When combined with AI algorithms, real-time analytics enables organizations to quickly detect anomalies or respond swiftly to market changes.
Real-time insights will prove immensely useful to sectors such as healthcare, finance and cyber security, enabling risk management and proactive decisions to be made quickly and decisively.
AI in Augmented Analytics
In 2023, the use of augmented analytics will grow. This is a mix between AI and analytics. AI-powered platforms for data insights will help users generate insights, identify patterns and ask meaningful questions without needing extensive data science knowledge.
Data-driven decisions will be more accessible for all business users, regardless of their level.
Want More Information About Our Services? Talk to Our Consultants!
Conclusion
Artificial Intelligence has revolutionised many fields. From transportation and finance, to healthcare and even sports - AIs impactful reach extends far across industries ranging from transportation, finance and healthcare.
Through AIs ability to reason and learn, machines now perform tasks once performed only by humans.
Artificial Intelligence applications like computer vision, natural language processing and robotics have revolutionized our daily lives, increasing efficiency and decision-making abilities while simplifying daily interactions and tasks.
Chatbots and virtual assistants have become more widespread. AI does present its own set of challenges; as technology becomes more ubiquitous it should ensure AI development and use comply with ethical practices while building public trust through transparent, fair and private algorithms.
AI represents both promise and uncertainty for societys future, raising important ethical biases, job displacement and other concerns to consider as it advances.
Government leaders, industry experts and researchers need to collaborate closely in shaping its development if AIs potential is to become reality and make our lives better through technology.
As AI will transform how we work, live and interact with it - to use its potential responsibly while harnessing its promise in creating an AI-enhanced age, lets aim at aligning AI development with human values while working toward contributing towards strengthening humanitys development as we advance into this AI age!