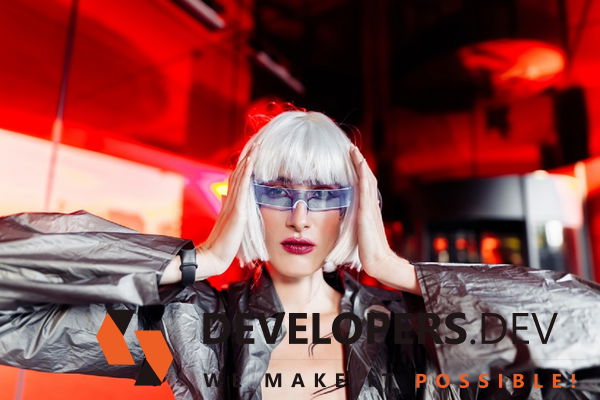
Opinions vary on what constitutes quality data both across industries and within companies, according to experts who suggest measuring various features or aspects for quality assessment purposes; data quality dimensions provide usable measurements.
What Is Data Quality
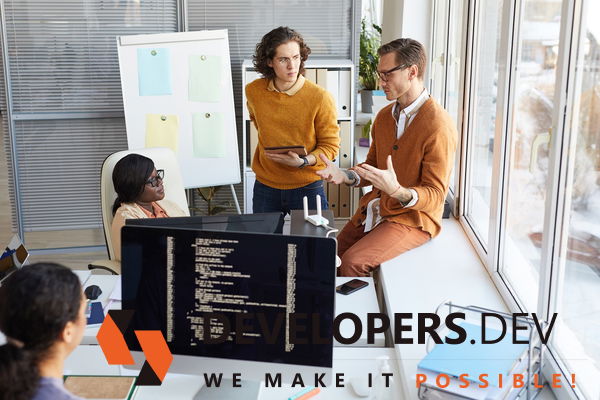
Data Quality can be defined as a measure of the datas condition. This takes into account factors like accuracy, consistency, integrity and completeness - with high scores across these aspects representing high-quality material thats suitable for analysis and processing.
Your company relies heavily on data. Poor data quality has the potential to cost companies up to 20% of their revenues, with business intelligence only ever being as accurate as its source material - dont allow inaccurate input to devalue data analysis efforts.
Data of high quality is of critical importance for businesses that rely heavily on customer databases and marketing campaigns, where customer trust must be built upon consistency in data that represents both current and past activities.
If data quality drops significantly below expected levels, trust may diminish with customers as your market position could suffer, as well as your business user .
How Should I Measure Data Quality?
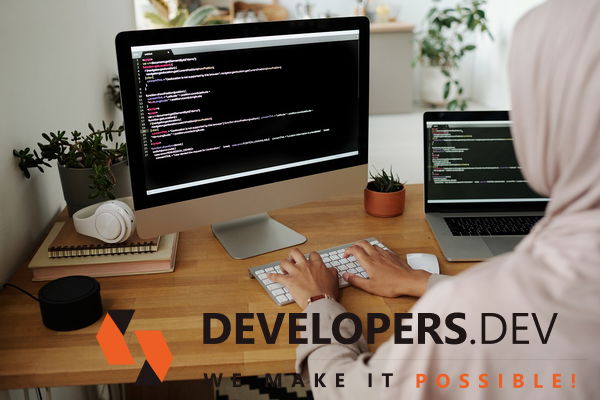
In order to effectively manage data quality solutions, regularly evaluate its quality with Gartners six points of reference for measuring it:
- Consistency: Should values of information stored across different locations remain consistent?
- Accuracy: Does the data accurately represent its properties in their intended application?
- Relevance: Do the values support goal pursuit? Existence: Is your organization possessing all required data sets?
- Integrity: How accurate are relationships among elements in data sets and their related datasets?
- Validity: Is The Value Acceptable?
Most companies rely on software to detect and correct errors in their data, with Informatica Data Quality currently one of the market leaders offering software designed specifically to do that job.
A robust market exists for Data Quality Software that ensures clean, useful information to organizations of any size - some programs even go further by offering features beyond data assessments like program management, role, use case, processes (for monitoring remediating reporting data quality issues etc.) setting organizational structures as well as program assessments.
Do You Have Bad Data Quality? Luckily, four steps exist to assist in creating an improved data quality strategy.
- Create departments within your company dedicated to data administration, providing executives with all of the tools required for quality. The data value should also be carefully assessed as its numbers will directly relate to key performance indicators of your business for optimal success.
- Establish accurate time estimates when planning to implement data quality software. Many organizations underestimate this timeframe, leading them to mistrust IT with business.
- Make the most out of your tools to ensure data quality. Optimizing costs means being flexible, optimizing value and upholding high standards.
- Effective data quality management will enable organizations to avoid crises. At its heart is an effective data team led by an effective CDO who ensures top management buy-in, program directors who oversee daily operations and Business Analysts who analyze organizational needs for developers.
Data Quality Management

Data profiling is an integral component of Big Data management, comprising three steps: reviewing data, comparing it against metadata, running statistical models and reporting.
Profiling helps establish standards and facilitate organization while the repair of errors is possible as part of data repair; repair involves discovering why and how errors occurred as well as the most efficient ways to correct them.
No doubt, it comes as no surprise that businesses are seeking one central point for data management and relevant updates.
A business intelligence (BI) platform combines all forms of data sources into one coherent collection for optimal management - leaving more resources freed up for strategic goals rather than warehouse maintenance tasks.
Data Quality Management: How To Implement And How It Works
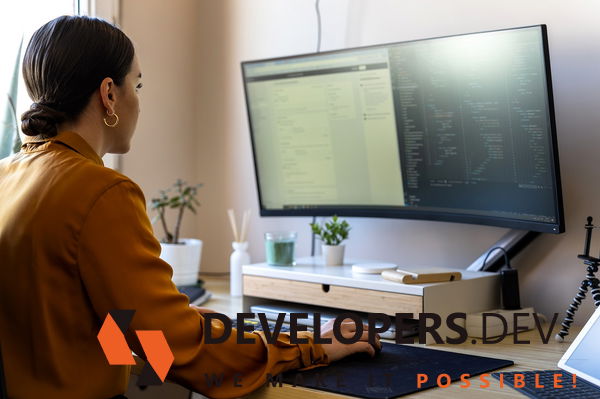
DQM (Data Quality Management) is an umbrella term covering best practices designed to increase and sustain data quality across an enterprises business units.
An expert on DQM implementation and maintenance, its effects should be permanent rather than reactive; its essence entails continual analysis, improvement, observation and monitoring - not fixing problems after they arise. This cycle should allow proactive control rather than reactive solutions being needed later.
1. Assessing Data Quality Helps Determine Its Performance Impact
Data analysts should first conduct an in-depth review of data to detect issues that could cause delays and, consequently, reduce revenue or alter margins.
A qualitative data review provides insights into which flaws in the information are having the biggest effect on business operations and processes. Afterwards, an expert or experts will outline quality requirements as well as critical dimensions within an organization.
The team then begins assessing data quality using both top-down and bottom-up approaches, respectively. Top-down assessments allow employees to gain an understanding of how employees use and create data, any problems encountered along the way and which are most significant; bottom-up approaches allow an evaluation of operations most negatively affected by poor-quality information.
Data quality analysts often conduct user interviews or surveys, assess data storage arrangements in databases and organize surveys in order to track any issues with quality.
Data profiling is one of several statistical tools and techniques used to implement the bottom-up methodology. Data Profiling employs different analytical and statistical algorithms as well as business rules in order to investigate both content characteristics of datasets as well as any specific characteristics within them.
Structure discovery (structure analysis) is used to verify if data are consistent and accurately formatted, using pattern matching of record structures to explore record structures further.
To gain more insights into the validity of their data, analysts can utilize statistical measures like minimum/maximum values along with medians/standard deviations/means to gain further knowledge of data validity.
- Content discovery involves searching each record in a database for invalid values or formatting issues that require attention.
- Relationship discovery refers to understanding the interrelationships among datasets, records, fields or cells in a database.
- Metadata review and analysis serve as essential steps toward this end; both serve to detect duplicate entries that might exist between datasets that do not align directly.
- Analysts may then consult domain experts regarding any concerns that they may have identified.
2. Definitions and Benchmarks
To establish metrics and rules related to data quality, analysts first assess assessments that reveal which elements seem critical given user needs.
According to experts in The Practitioners Guide to Data Quality Improvement, empirical analysis provides various measures that can be utilized when judging quality in specific business contexts.
DQ analysts use business rules to correlate errors with business impact. If any mismatch exists between errors and business impact, data quality metrics specialists then create metrics used for operational and analytical use of the data.
Data users are then consulted regarding acceptable thresholds of metrics scores; otherwise, it must be corrected so as not to have negative consequences on operations by integrating measurement methods and acceptable thresholds together into one metric for measuring the quality of data quality.
3. Establish Data Standards, Metadata Management Standards And Validation Rules
Once data analysis and quality metrics and rules have been clearly established, techniques and activities for quality enhancement must be undertaken to bring out their full potential.
This stage aims to develop an enforceable set of data/metadata rules which can be utilized throughout its entire data lifecycle life.
Data quality standards. Data standards refer to agreements across organizations on data representation, format, exchange and entry processes.
Data governance and successful analytics depend heavily on policies and rules for metadata creation and management.
There are three general categories of metadata standards management:
- Business: Use of terms, definitions and acronyms within different contexts of the business world; data security level and privacy settings.
- Technical: Data storage structures, including structure rules (i.e. format size indexes etc.) as well as models.
- Operational Rules: Rules used with ETL metadata that describe events and objects (i.e. update date, loading date, confidence indicator).
Note that some professionals consider operational metadata a technical type.
Rules for Data Validity, Data validity rules, evaluate data for consistency. Written by developers and integrated into software applications, validity rules help detect mistakes even as new information enters systems; effectively managing quality management can become simpler with data validity rules in place.
Deliberating how you will keep track of data issues is also of vital importance. A data quality issue tracker log may contain details regarding flaws and their severity as well as who was responsible for fixing them and any report notes from employees who report these flaws; George Firican of the University of British Columbia offers helpful insight on what attributes should be included.
Enhancing data is another factor to keep in mind and approve; we will discuss them further below.
4. Implementation and Administration of Data Quality Standards
To guarantee data quality throughout its entire lifecycle, the team charged with data quality will implement standards and procedures outlined earlier to maintain it in an effort to preserve quality control standards and procedures that have been drafted earlier.
Team members may arrange meetings to familiarize employees with new rules of data management or create an approved glossary of business terms approved by managers and stakeholders.
Data quality teams also train employees how to correct data using any tool - be it customized or off-the-shelf.
Also Read: What Are The Possible Solutions Of Using Big Data?
5. Monitoring and Correcting Data
Data cleaning (also referred to as remediation or preparation) involves the process of finding, correcting and deleting erroneous, incomplete, or inaccurate records in databases.
Data preparation may take many forms: manual processes using tools specifically tailored for quality analysis or through batch processing scripts such as those found with tools for data quality, scripts or migration software.
Data remediation comprises various activities, such as:
- Root Causes: of errors can be identified by looking at their source, reasons leading up to them, contributing factors and solutions available for removal of them.
- Standardization and Parsing: Review records in database tables to match predetermined grammar patterns to find instances of incorrect data within fields and format them appropriately in order to detect incorrect values which have been entered incorrectly into fields or are in incorrect fields altogether.
- Data Quality Analysts: may perform such practices to standardize values from various systems like the pounds-kg scale or geographic abbreviations for analysis.
- Matching Data: involves finding entities that are similar or identical in one dataset and merging those together into a single entity.
- Matching Closely: resembles record linking and identity resolution techniques used when joining datasets together or merging multiple sources data together (the ETL). Identity resolution helps create single customer views from datasets containing records for individuals, while record linking can link records not related directly (such as database key, social security number or URL address ) through shared characteristics like shape, location or curator preferences.
- Addition of Data: Gathering new information from both internal and external sources. Monitoring is the practice of reviewing information at regular intervals to make sure it serves its intended purpose.
Who defines and applies the metrics and standards necessary for perfect data? Who would evaluate data, train other employees on its use or be accountable for technical aspects?
Roles and Responsibilities of the Data Quality Team
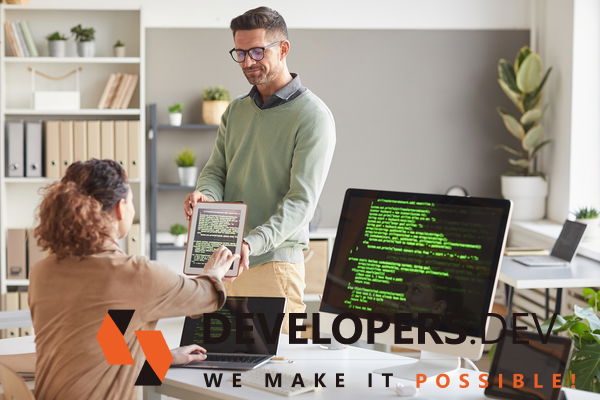
Data governances Data Quality aspect seeks to maximize data usage at company-wide levels for optimal effectiveness, overseeing CDOs (Chief Data Officers) who have oversight for usage within an organization as well as overseeing data quality specialists from within an exemplary data team.
CDOs oversee all aspects of governance within an enterprise while being charged with building it themselves as needed.
Data quality teams depend on the size and scope of their respective businesses to be formed accordingly, while members typically include both technical experts as well as business expertise collaborating together on solving data quality problems.
Roles may include:
Data Owner - Manages data quality within one or multiple datasets for control. Sets requirements.
In teams, data owners usually represent senior executives.
Data Consumer - Someone who regularly consumes data and reports errors as well as sets standards.
Data Producer - Capturing data while meeting consumer quality requirements.
Data Stewards - typically oversee the content, context and business rules associated with any data.
They ensure employees adhere to written guidelines and standards regarding the generation, use and access of data/metadata. Sometimes sharing responsibility with data custodians; offering advice to improve data governance as needed.
Data Custodians - oversee technical environments to maintain and store information securely. Data custodians also ensure its integrity and quality processes when engaging in ETL activities (extract transform and load).
You can learn more about common job titles held by data custodians here.
Data Analyst - Explore, evaluate and summarize data before reporting results back to stakeholders.
Multitasking Data Quality Analyst
Data quality analyst duties may range in scope. Experts in data consumers duties, such as standardizing and documenting information, can perform data custodian-type duties or maintain the quality before data warehouses load it all up into warehouses for warehouse storage - among many other activities performed by data quality analysts.
Their responsibilities could also include:
- Monitor and review data quality (accuracy and integrity) that users enter into corporate systems as well as that which is extracted, transformed and loaded into data warehouses, discovering causes and rectifying data problems as quickly as possible.
- Assess and report to management on data quality assessments and improvements that occur, along with measures taken for ongoing data quality management. Quality control procedures and policies, such as communication protocols or service agreements with suppliers of data, should also be established and adhered to closely.
- Data quality initiatives can be documented to demonstrate their return on investment.
Company Management may entrust an analyst with organizing and providing data quality training for employees as well as suggesting steps that will increase the suitability of data for specific tasks or applications. Furthermore, this specialist could be held accountable for upholding the data privacy policies of their organization.
Your data quality team responsibilities should include an administrator for managing and overseeing all processes involved, someone to perform quality assurance checks and enforce data quality rules, someone who develops models and data sources, as well as technical experts who keep things flowing throughout the company.
2023 Trend Analysis on Data Quality Trends
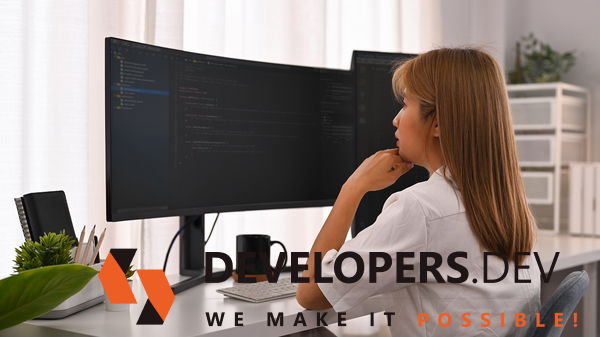
1. An Approach to Data Quality
An Approach to Data Quality with High Volume Needs Cloud-based platforms for analytics allows enterprises to perform large-scale computations at an economic level, something not possible just a few short years ago.
Digitized processes in business, widespread smartphone adoption and IoT sensors that generate masses of data generate mountains of new information every year.
Data quality programs must adapt as the interconnectivity of our world increases. Organizations once struggled to manage small data sets effectively; duplicate records and declining quality were major concerns when managing customer databases; now, however, these issues have grown far more complicated and numerous than before.
This documents goal is to assist in understanding how you can address data quality problems. Not only will it identify specific patterns or types of issues, but it will also describe how data management and governance help solve such problems.
Enterprises of today must consider data quality from an enterprise-scale viewpoint. Data quality tools provide powerful mechanisms for discovering, profiling, cataloging and creating sophisticated business rules to define quality data - in turn, automating tasks to bring problems directly to the line of business data owners for attention.
2. Data Democratization Relies on High Data Quality
Data democratization can enable all members of an organization to make better decisions; however, its success depends on high data quality; otherwise, it could result in poor decisions with serious repercussions for an organizations stakeholders.
Recent Data Trust Survey results illustrate this contrast, showing that frontline employees from sales/marketing, operations and HR tend to be least confident about the quality of data used when making important decisions versus top executives who tend to trust it more when taking those important steps.
Leaders looking to democratize data within their organizations face major implications from low trust levels; when that occurs, usage will decrease, and businesses wont maximize all available assets, such as data assets resulting in missed opportunities and lost potential revenue streams.
Proactive, scalable programs to improve data quality offer two significant advantages to organizations: they increase overall trust in data integrity within an organization while simultaneously providing more precise information for improved business decisions.
3. AI/ML Raiser the Stakes for Data Quality
Businesses are investing heavily in AI/ML technologies, but for algorithms to function accurately, they require clean and accurate data for training.
Imagine having recorded incorrectly formatted addresses or missing income levels; machine-learning algorithms would then use such information as input into its prediction of purchasing behavior by demographic groups without specific instructions unless told not to; your team could use such inaccurate forecasts when building demand forecasts.
AI/ML can be an incredibly valuable business asset, yet its usage should always be carefully considered to prevent misguided outcomes.
AI functions better, with data quality being high. Poor data may cause issues that only become clear after it has happened - which may not even become evident until after too much damage has been done to reputational assets like businesses have already taken.
4. High-Quality Data Is Essential For Compliance
Businesses invest more resources, time and energy towards meeting government and contractual regulations or reporting standards with more robust data quality requirements than ever.
Reporting on environmental, social and governance (ESG) issues is becoming a priority for global corporations. Without standards in reporting ESG metrics accurately, critics accuse enterprises of "greenwashing", misrepresenting ESG metrics to meet financial goals or impress investors.
Even when false accusations exist against enterprises, they must work tirelessly to dispel any doubt.
Government regulators continue to increase requirements for timely and accurate reporting to comply with various new mandates, while large customers demand vendors meet increasingly stringent standards while regularly reporting key metrics.
Improved data quality reduces financial and reputational risk.
5. Data Quality Is At the Core of Effective Governance
Data governance has quickly become a standard feature within most enterprises; by 2023, it will have become de facto mandatory across any and every enterprise that wants to leverage its data for strategic or tactical advantage.
Data in business has grown increasingly complex over time. Digital consumer interactions, geospatial data processing and digitizing business processes provide ample opportunity for organizations of all sizes while increasing regulatory complexity presents unique challenges.
Businesses of all kinds must understand what data has been given to them by customers or partners so that they may properly protect it and control this vital resource.
Data Quality should always come first when considering data governance to achieve its aim of increasing trust and compliance within company data.
Effective management of data quality on an ongoing basis is vitally important.
More and more organizations are searching for proactive methods of data quality management and governance. This report can assist in the identification and resolution of root causes resulting in poor data quality, not only describing various forms of issues but revealing roles played by both data quality management and governance in providing solutions.
Conclusion
Data quality is of great significance, yet many can take its importance for granted. Proper data management enables greater operational efficiencies, cost reductions and a solid decision-making foundation.
Even for large volumes of information, successful analytics operations become much simpler to manage and more accurate over time.